Consistent Multimodal Generation via A Unified GAN Framework
2024 IEEE/CVF Winter Conference on Applications of Computer Vision (WACV)(2023)
摘要
We investigate how to generate multimodal image outputs, such as RGB, depth, and surface normals, with a single generative model. The challenge is to produce outputs that are realistic, and also consistent with each other. Our solution builds on the StyleGAN3 architecture, with a shared backbone and modality-specific branches in the last layers of the synthesis network, and we propose per-modality fidelity discriminators and a cross-modality consistency discriminator. In experiments on the Stanford2D3D dataset, we demonstrate realistic and consistent generation of RGB, depth, and normal images. We also show a training recipe to easily extend our pretrained model on a new domain, even with a few pairwise data. We further evaluate the use of synthetically generated RGB and depth pairs for training or fine-tuning depth estimators. Code will be available at https://github.com/jessemelpolio/MultimodalGAN.
更多查看译文
关键词
consistent multimodal generation
AI 理解论文
溯源树
样例
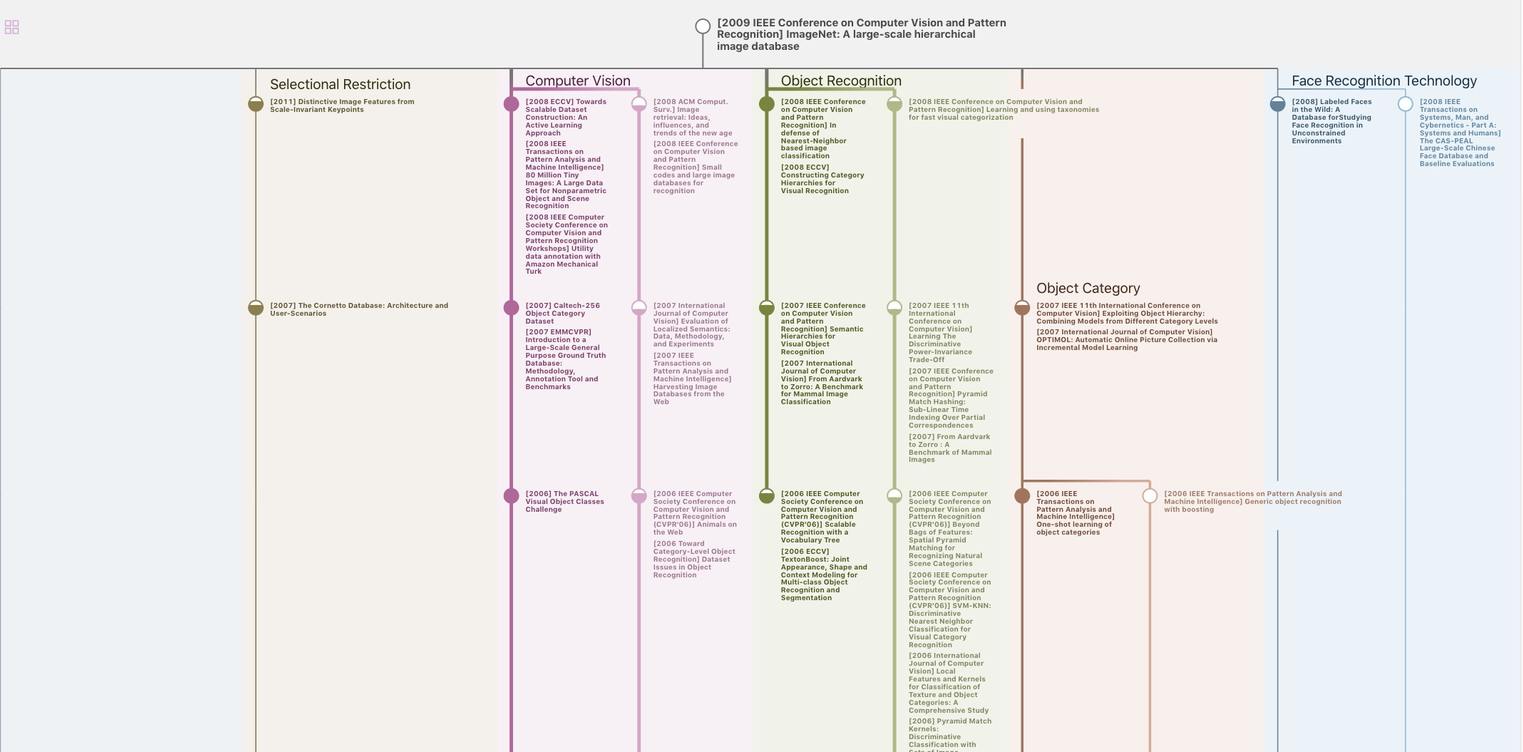
生成溯源树,研究论文发展脉络
Chat Paper
正在生成论文摘要