Multi-Task Learning Improves Performance In Deep Argument Mining Models
CoRR(2023)
摘要
The successful analysis of argumentative techniques from user-generated text is central to many downstream tasks such as political and market analysis. Recent argument mining tools use state-of-the-art deep learning methods to extract and annotate argumentative techniques from various online text corpora, however each task is treated as separate and different bespoke models are fine-tuned for each dataset. We show that different argument mining tasks share common semantic and logical structure by implementing a multi-task approach to argument mining that achieves better performance than state-of-the-art methods for the same problems. Our model builds a shared representation of the input text that is common to all tasks and exploits similarities between tasks in order to further boost performance via parameter-sharing. Our results are important for argument mining as they show that different tasks share substantial similarities and suggest a holistic approach to the extraction of argumentative techniques from text.
更多查看译文
关键词
deep argument mining models,multi-task
AI 理解论文
溯源树
样例
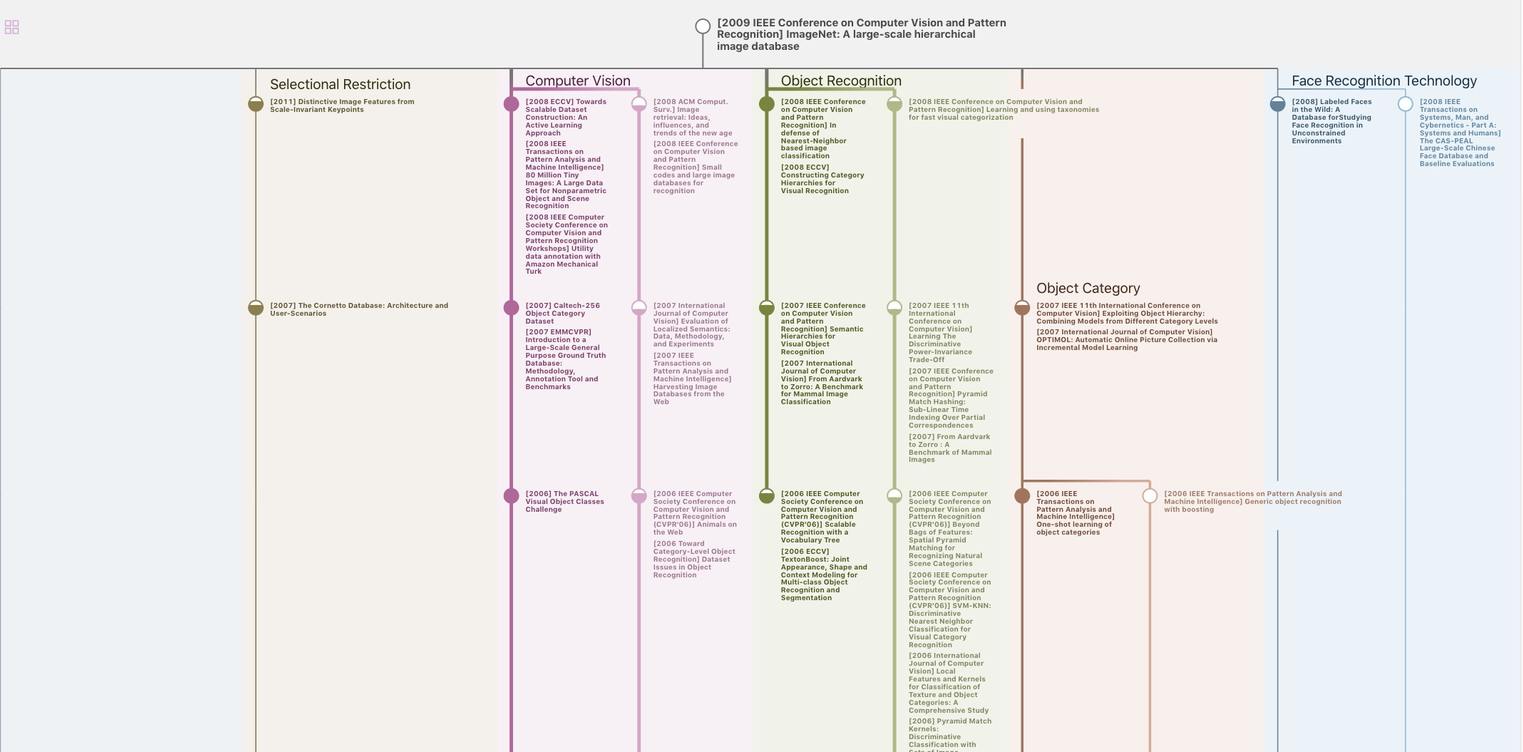
生成溯源树,研究论文发展脉络
Chat Paper
正在生成论文摘要