In-depth analysis on parallel processing patterns for high-performance Dataframes
CoRR(2023)
摘要
The Data Science domain has expanded monumentally in both research and industry communities during the past decade, predominantly owing to the Big Data revolution. Artificial Intelligence (AI) and Machine Learning (ML) are bringing more complexities to data engineering applications, which are now integrated into data processing pipelines to process terabytes of data. Typically, a significant amount of time is spent on data preprocessing in these pipelines, and hence improving its efficiency directly impacts the overall pipeline performance. The community has recently embraced the concept of Dataframes as the de-facto data structure for data representation and manipulation. However, the most widely used serial Dataframes today (R, pandas) experience performance limitations while working on even moderately large data sets. We believe that there is plenty of room for improvement by taking a look at this problem from a high-performance computing point of view. In a prior publication, we presented a set of parallel processing patterns for distributed dataframe operators and the reference runtime implementation, Cylon [1]. In this paper, we are expanding on the initial concept by introducing a cost model for evaluating the said patterns. Furthermore, we evaluate the performance of Cylon on the ORNL Summit supercomputer.
更多查看译文
关键词
Dataframes,High-performance computing,Data engineering,Relational algebra,MPI,Distributed Memory Parallel
AI 理解论文
溯源树
样例
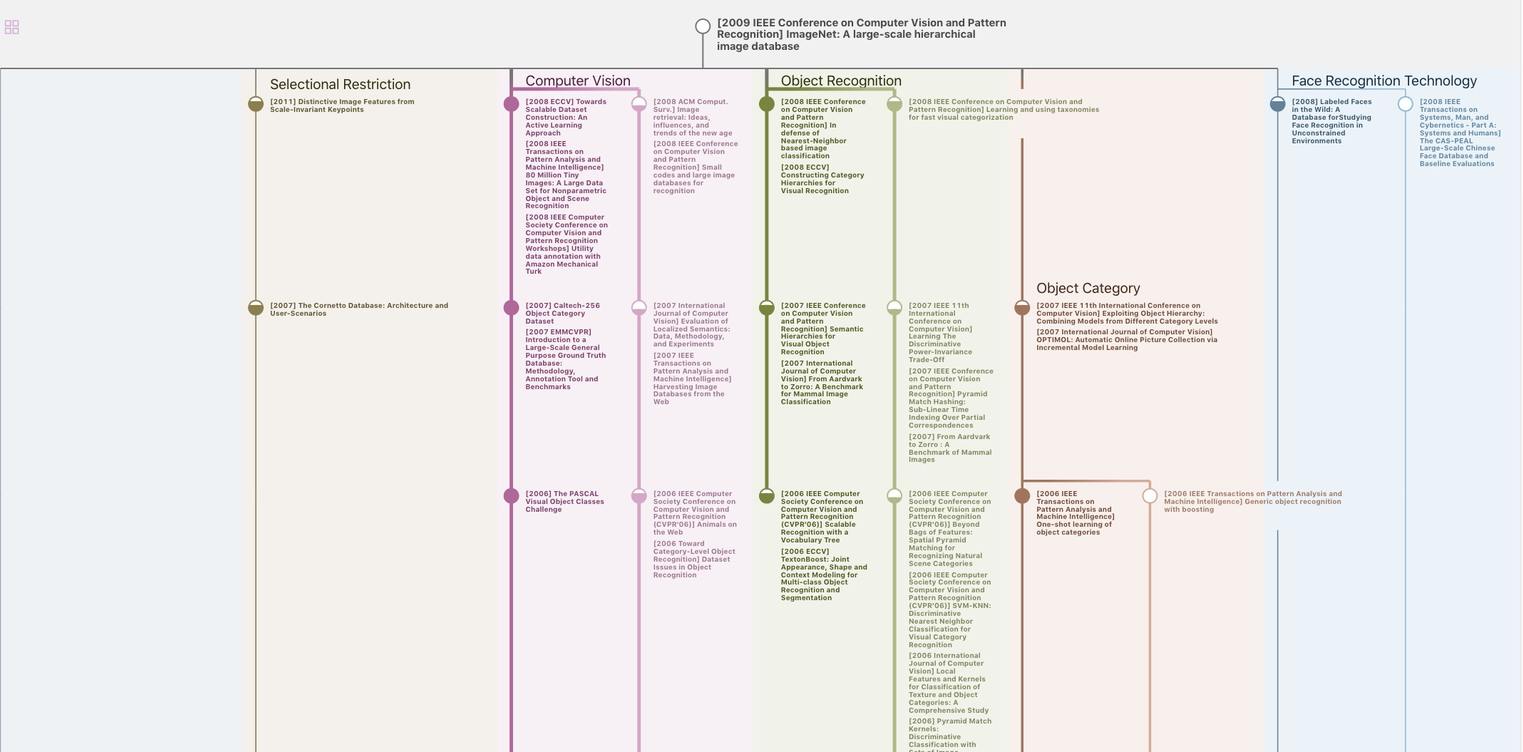
生成溯源树,研究论文发展脉络
Chat Paper
正在生成论文摘要