A Mean Field to Capture Asynchronous Irregular Dynamics of Conductance-Based Networks of Adaptive Quadratic Integrate-and-Fire Neuron Models.
Neural computation(2024)
摘要
Mean-field models are a class of models used in computational neuroscience to study the behavior of large populations of neurons. These models are based on the idea of representing the activity of a large number of neurons as the average behavior of mean-field variables. This abstraction allows the study of large-scale neural dynamics in a computationally efficient and mathematically tractable manner. One of these methods, based on a semianalytical approach, has previously been applied to different types of singleneuron models, but never to models based on a quadratic form. In this work, we adapted this method to quadratic integrate-and-fire neuron models with adaptation and conductance-based synaptic interactions. We validated the mean-field model by comparing it to the spiking network model. This mean-field model should be useful to model large-scale activity based on quadratic neurons interacting with conductance-based synapses.
更多查看译文
AI 理解论文
溯源树
样例
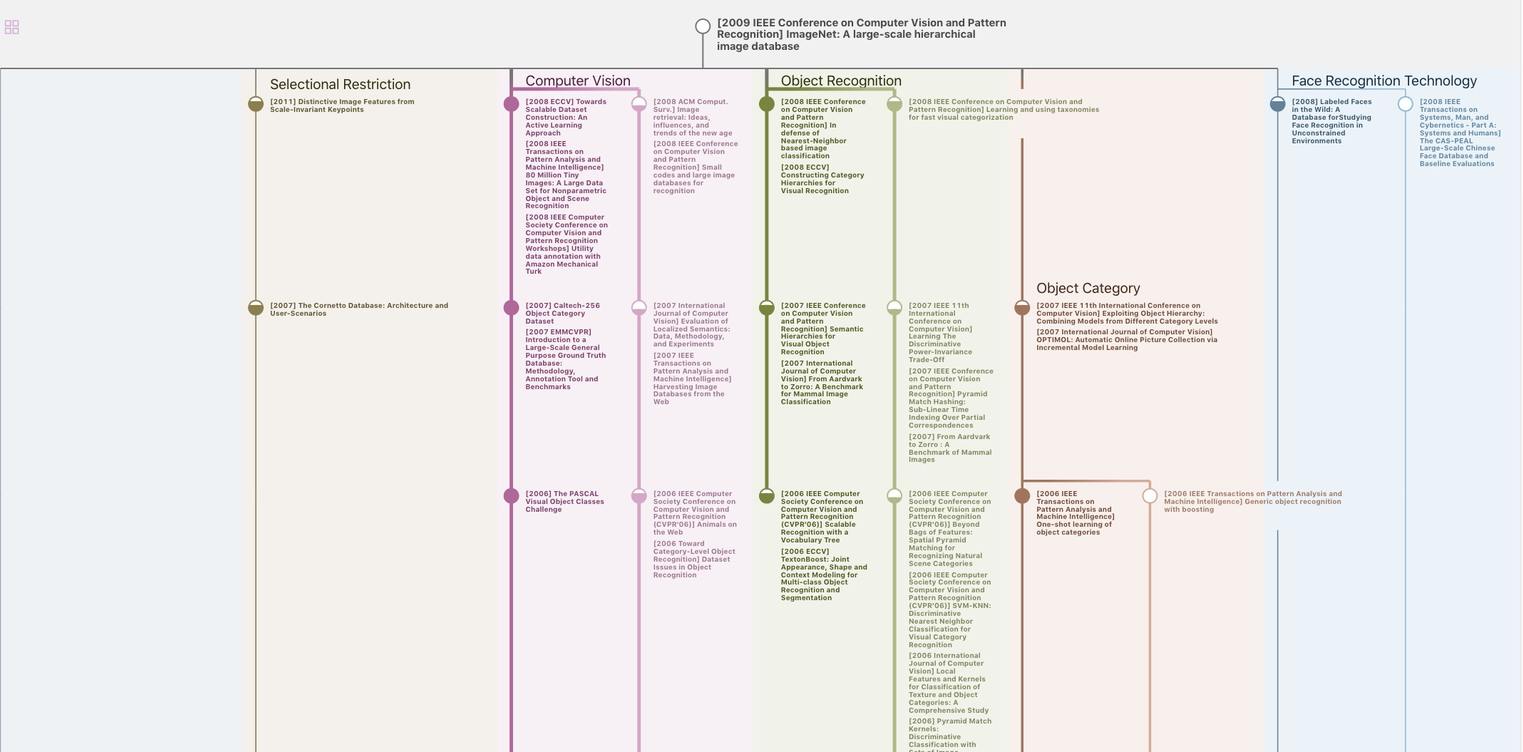
生成溯源树,研究论文发展脉络
Chat Paper
正在生成论文摘要