Model-free Identification of Phenotype-Relevant Variables From Dose Response Data
bioRxiv (Cold Spring Harbor Laboratory)(2023)
摘要
Complex phenotypic changes occur during development and in response to injury and disease. Identifying key regulators of phenotypic change is a shared aim of many different fields of research, including life history, tissue regeneration, and cancer. These examples of phenotypic change involve coordinated changes in cellular behaviors and associated changes in gene expression which if disrupted, can alter and even block completion of the phenotypic transition. Experimental treatments that effectively block the completion of a phenotypic transition can be quantitatively manipulated to identify key regulatory genes through changes in transcriptional dose response. In this paper, we present a “model free” approach to identify “bifurcation-like” behaviors of key regulatory genes by tracking spikes in their transcriptional sensitivities. Genes that exhibit such behavior are predicted to comprise nodes in subnetworks or modules that regulate the phenotypic transition. We applied our method to an in-silico data set where we also studied the impact of noise in the predictions. We also applied the method to a gene expression data that were collected during tail regeneration in axolotls. Our code for gene identification, which can be extended more generally to other component variables of complex phenotypic change, is freely available via the following GitHub site [github.com/alanavc/id-vars-from-resp-data][1].
### Competing Interest Statement
The authors have declared no competing interest.
[1]: http://github.com/alanavc/id-vars-from-resp-data
更多查看译文
关键词
model-free,phenotype-relevant
AI 理解论文
溯源树
样例
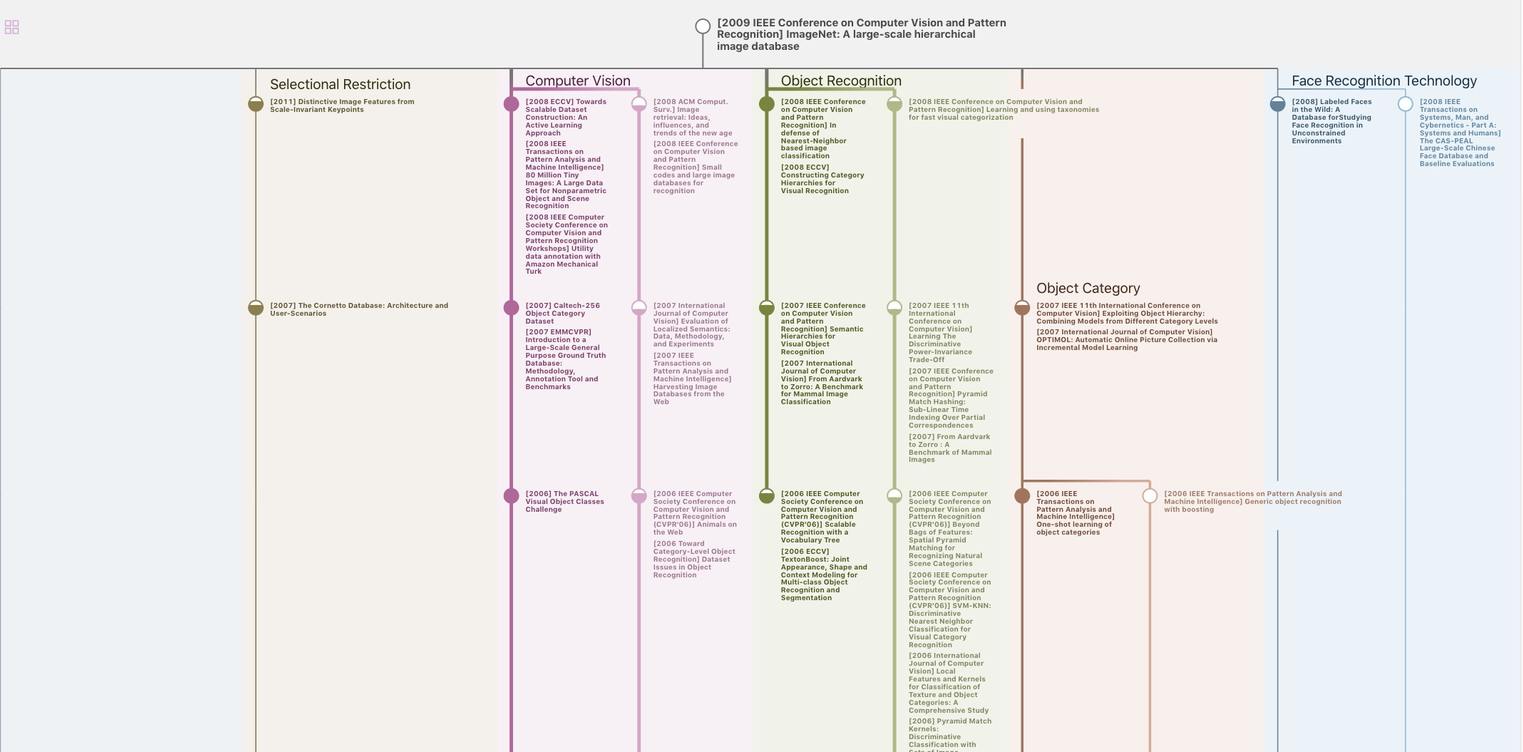
生成溯源树,研究论文发展脉络
Chat Paper
正在生成论文摘要