Task-driven neural network models predict neural dynamics of proprioception
Cell(2024)
摘要
Proprioception tells the brain the state of the body based on distributed sensory neurons. Yet, the principles that govern proprioceptive processing are poorly understood. Here, we employ a task-driven modeling approach to investigate the neural code of proprioceptive neurons in cuneate nucleus (CN) and somatosensory cortex area 2 (S1). We simulated muscle spindle signals through musculoskeletal modeling and generated a large-scale movement repertoire to train neural networks based on 16 hypotheses, each representing different computational goals. We found that the emerging, task-optimized internal representations generalize from synthetic data to predict neural dynamics in CN and S1 of primates. Computational tasks that aim to predict the limb position and velocity were the best at predicting the neural activity in both areas. Since task optimization develops representations that better predict neural activity during active than passive movements, we postulate that neural activity in the CN and S1 is top-down modulated during goal-directed movements.
更多查看译文
关键词
proprioception,task-driven models,neural networks,somatosensory cortex,cuneate nucleus,state estimation,efference copy,goal-driven models,biomechanics,statistics of movement
AI 理解论文
溯源树
样例
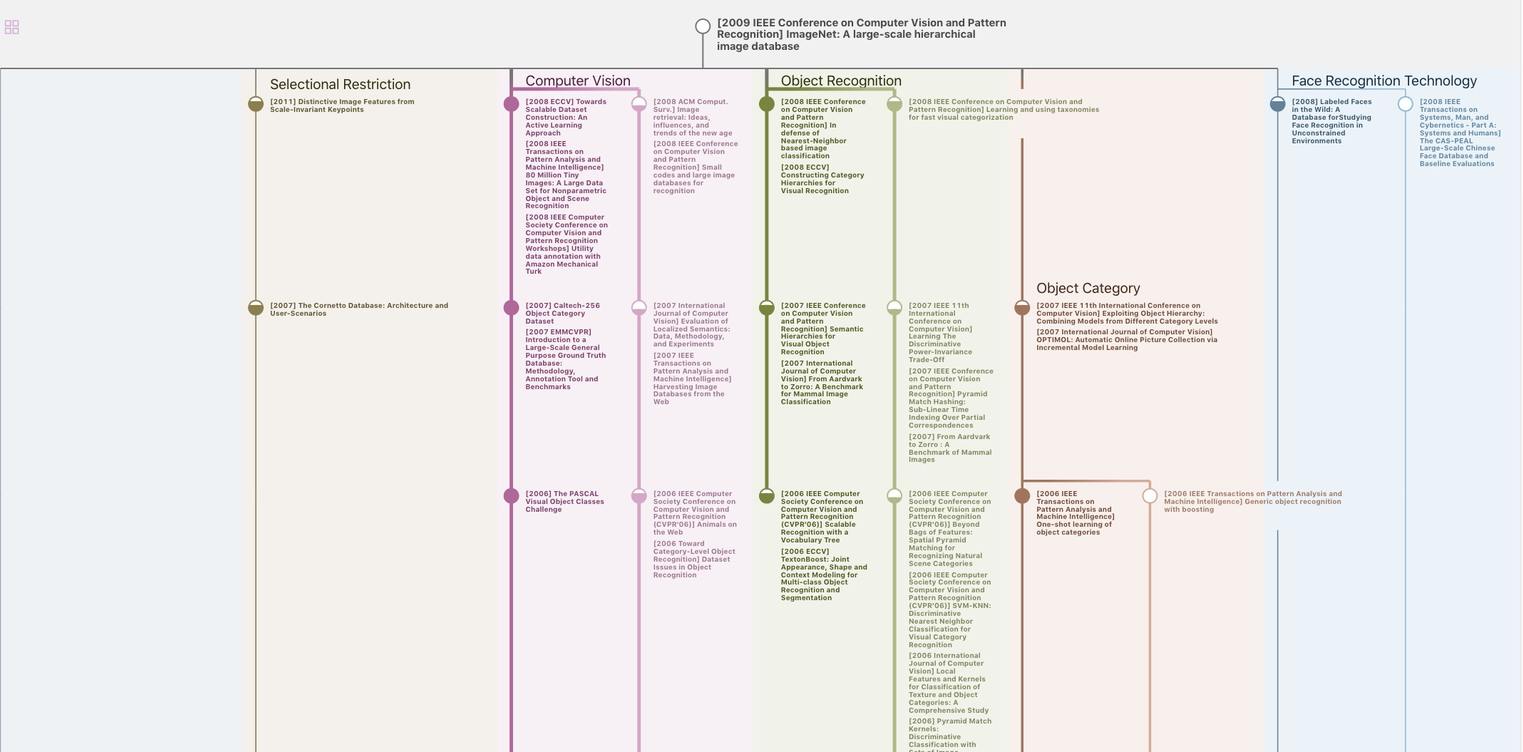
生成溯源树,研究论文发展脉络
Chat Paper
正在生成论文摘要