The effects of data leakage on neuroimaging predictive models
bioRxiv (Cold Spring Harbor Laboratory)(2023)
摘要
Predictive modeling has now become a central technique in neuroimaging to identify complex brain-behavior relationships and test their generalizability to unseen data. However, data leakage, which unintentionally breaches the separation between data used to train and test the model, undermines the validity of predictive models. Although previous literature suggests that leakage is generally pervasive in machine learning, few studies have empirically evaluated the effects of leakage in neuroimaging data. Here, using over 500 different pipelines spanning four large neuroimaging datasets and three phenotypes, we evaluated six forms of leakage fitting into three broad categories: feature selection, covariate correction, and lack of independence between subjects. As expected, leakage via feature selection and repeated subjects drastically inflated prediction performance. Notably, other forms of leakage had only minor effects (e.g., leaky site correction) or even decreased prediction performance (e.g., leaky covariate regression). In some cases, leakage affected not only prediction performance, but also model coefficients, and thus neurobiological interpretations. Overall, our results illustrate the variable effects of leakage on prediction pipelines and underscore the importance of avoiding data leakage to improve the validity and reproducibility of predictive modeling.
### Competing Interest Statement
The authors have declared no competing interest.
更多查看译文
关键词
data leakage,models
AI 理解论文
溯源树
样例
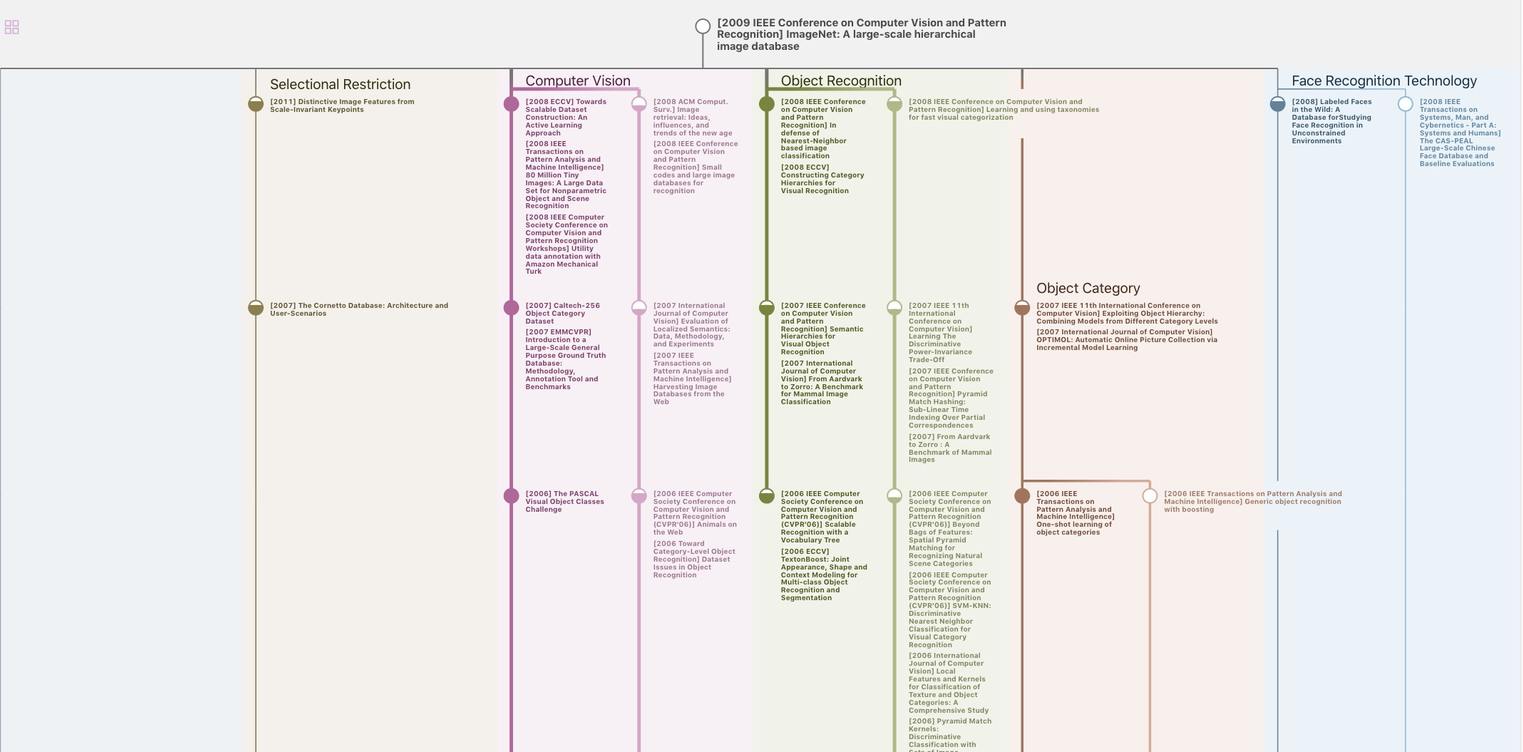
生成溯源树,研究论文发展脉络
Chat Paper
正在生成论文摘要