Identification of Mild Cognitive Impairment Subtypes Predicting Conversion to Alzheimer’s Disease Using a Heterogeneous Mixture Learning
Research Square (Research Square)(2020)
摘要
Abstract BackgroundMild cognitive impairment (MCI) is a high-risk condition for conversion to dementias, including Alzheimer's disease (AD) dementia. However, individuals with MCI show heterogeneity in patterns of pathology, and MCI does not always convert to AD dementia. Detailed subtyping of MCI and accurate prediction of the patients in whom MCI will convert to AD dementia may support new trial designs and enable evaluation of the efficacy of drugs within small numbers of patients during clinical trials. MethodsWe constructed a decision tree model by the heterogeneous mixture learning (HML) method, integrating cerebrospinal fluid (CSF) biomarker data, structural MRI data, APOE genotype data, and a recorded age at examination. The decision tree model was applied to predict conversion to AD dementia and to identify subtypes of MCI. After the test performances of HML models were assessed, MCI subjects were classified into some subtypes based on a decision tree. Then, we characterized each MCI subtype in terms of the degree of CSF biomarker abnormalities and brain atrophy, declines of cognitive functions, and gene expression alterations derived from peripheral blood samples.ResultsWe identified five subtypes of MCI using the HML approach and categorized them into three groups: those similar to CN subjects with low conversion rates; those with intermediate conversion rates; and those similar to patients with AD with high conversion rates. Furthermore, the subtypes with intermediate conversion rates were separated into the subtype with CSF biomarker abnormalities or the subtype with brain atrophy. The results from the CSF inflammation marker and gene expression analysis suggested the occurrence of aberrant inflammatory immune responses in the CSF and blood of the subjects in the subtypes with CSF biomarker abnormalities. ConclusionThe subtypes that were identified in this study exhibited varying conversion rates to AD as well as differing levels of biological features. Focusing on specific subtypes in which conversion to AD can be predicted with the most accuracy could enable more efficient clinical trials to be conducted.
更多查看译文
关键词
heterogeneous mixture learning,alzheimers disease,cognitive impairment
AI 理解论文
溯源树
样例
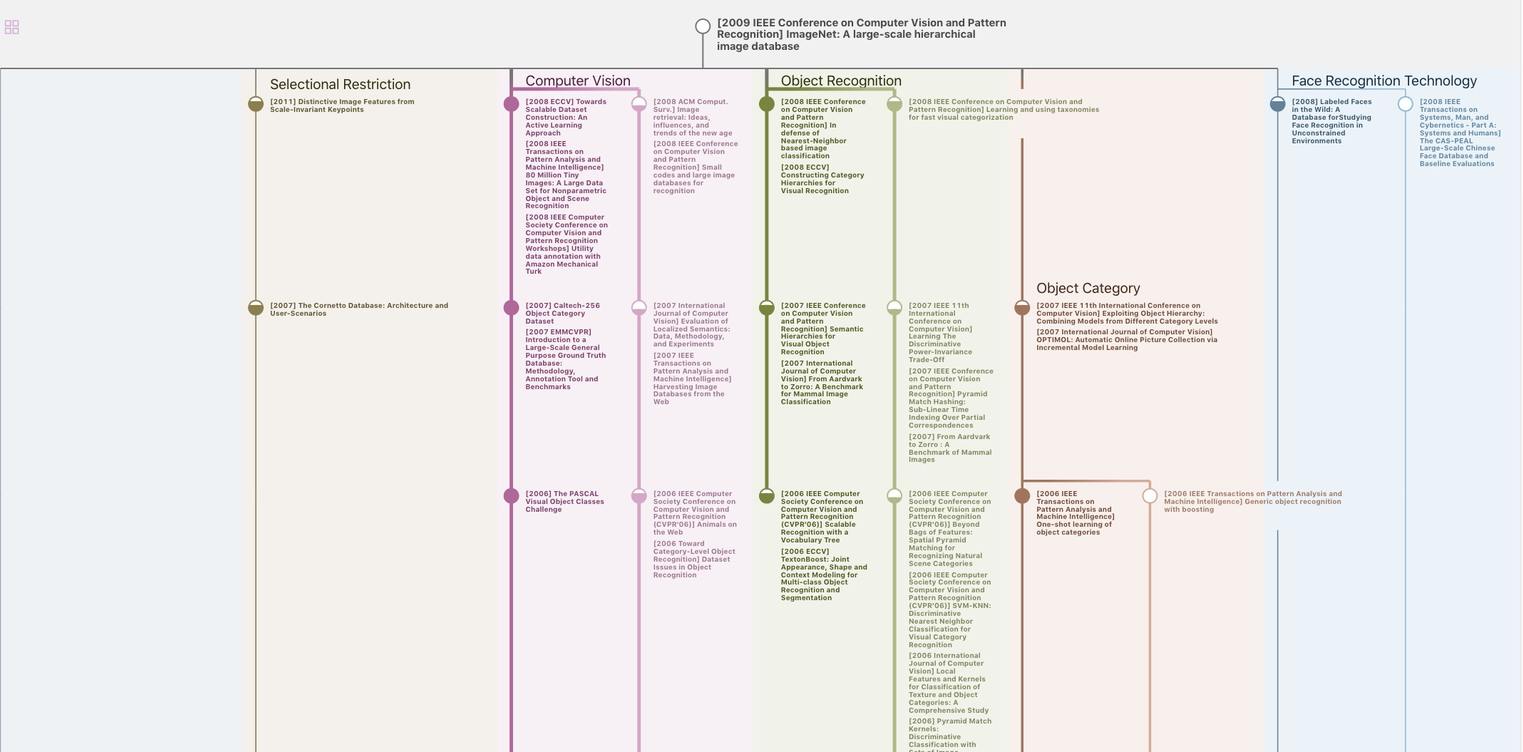
生成溯源树,研究论文发展脉络
Chat Paper
正在生成论文摘要