DeepHBV: A deep learning model to predict hepatitis B virus (HBV) integration sites
bioRxiv (Cold Spring Harbor Laboratory)(2021)
摘要
AbstractHepatitis B virus (HBV) is one of the main causes for viral hepatitis and liver cancer. Previous studies showed HBV can integrate into host genome and further promote malignant transformation. In this study, we developed an attention-based deep learning model DeepHBV to predict HBV integration sites by learning local genomic features automatically. We trained and tested DeepHBV using the HBV integration sites data from dsVIS database. Initially, DeepHBV showed AUROC of 0.6363 and AUPR of 0.5471 on the dataset. Adding repeat peaks and TCGA Pan Cancer peaks can significantly improve the model performance, with an AUROC of 0.8378 and 0.9430 and an AUPR of 0.7535 and 0.9310, respectively. On independent validation dataset of HBV integration sites from VISDB, DeepHBV with HBV integration sequences plus TCGA Pan Cancer (AUROC of 0.7603 and AUPR of 0.6189) performed better than HBV integration sequences plus repeat peaks (AUROC of 0.6657 and AUPR of 0.5737). Next, we found the transcriptional factor binding sites (TFBS) were significantly enriched near genomic positions that were paid attention to by convolution neural network. The binding sites of AR-halfsite, Arnt, Atf1, bHLHE40, bHLHE41, BMAL1, CLOCK, c-Myc, COUP-TFII, E2A, EBF1, Erra and Foxo3 were highlighted by DeepHBV attention mechanism in both dsVIS dataset and VISDB dataset, revealing the HBV integration preference. In summary, DeepHBV is a robust and explainable deep learning model not only for the prediction of HBV integration sites but also for further mechanism study of HBV induced cancer.Author summaryHepatitis B virus (HBV) is one of the main causes for viral hepatitis and liver cancer. Previous studies showed HBV can integrate into host genome and further promote malignant transformation. In this study, we developed an attention-based deep learning model DeepHBV to predict HBV integration sites by learning local genomic features automatically. The performance of DeepHBV model significantly improves after adding genomic features, with an AUROC of 0.9430 and an AUPR of 0.9310. Furthermore, we enriched the transcriptional factor binding sites of proteins by convolution neural network. In summary, DeepHBV is a robust and explainable deep learning model not only for the prediction of HBV integration sites but also for the further study of HBV integration mechanism.
更多查看译文
关键词
deephbv learning,deephbv learning model,virus
AI 理解论文
溯源树
样例
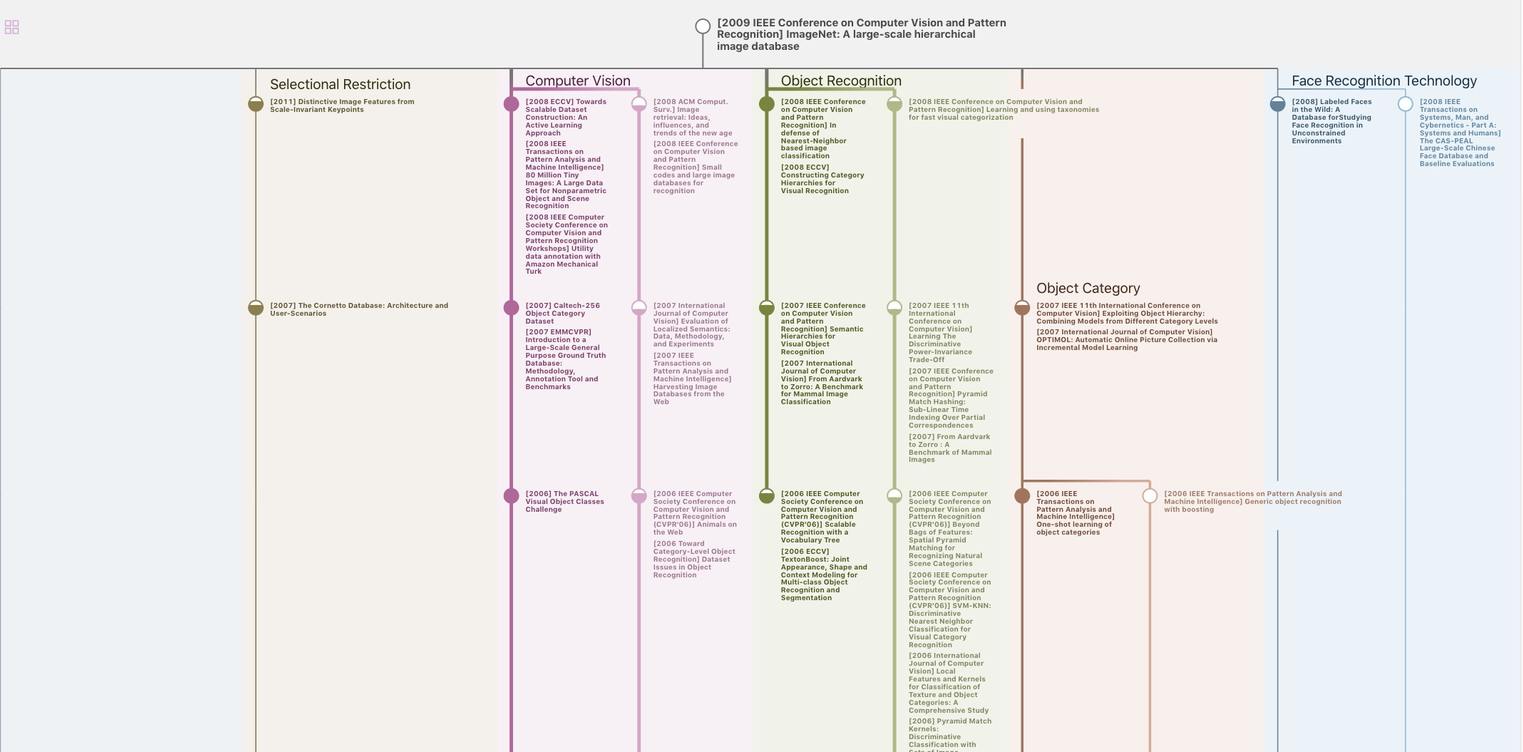
生成溯源树,研究论文发展脉络
Chat Paper
正在生成论文摘要