Representation learning applications in biological sequence analysis
bioRxiv (Cold Spring Harbor Laboratory)(2021)
摘要
Remarkable advances in high-throughput sequencing have resulted in rapid data accumulation, and analyzing biological (DNA/RNA/protein) sequences to discover new insights in biology has become more critical and challenging. To tackle this issue, the application of natural language processing (NLP) to biological sequence analysis has received increased attention, because biological sequences are regarded as sentences and k-mers in these sequences as words. Embedding is an essential step in NLP, which converts words into vectors. This transformation is called representation learning and can be applied to biological sequences. Vectorized biological sequences can be used for function and structure estimation, or as inputs for other probabilistic models. Given the importance and growing trend in the application of representation learning in biology, here, we review the existing knowledge in representation learning for biological sequence analysis.
更多查看译文
关键词
biological sequence analysis,representation,learning
AI 理解论文
溯源树
样例
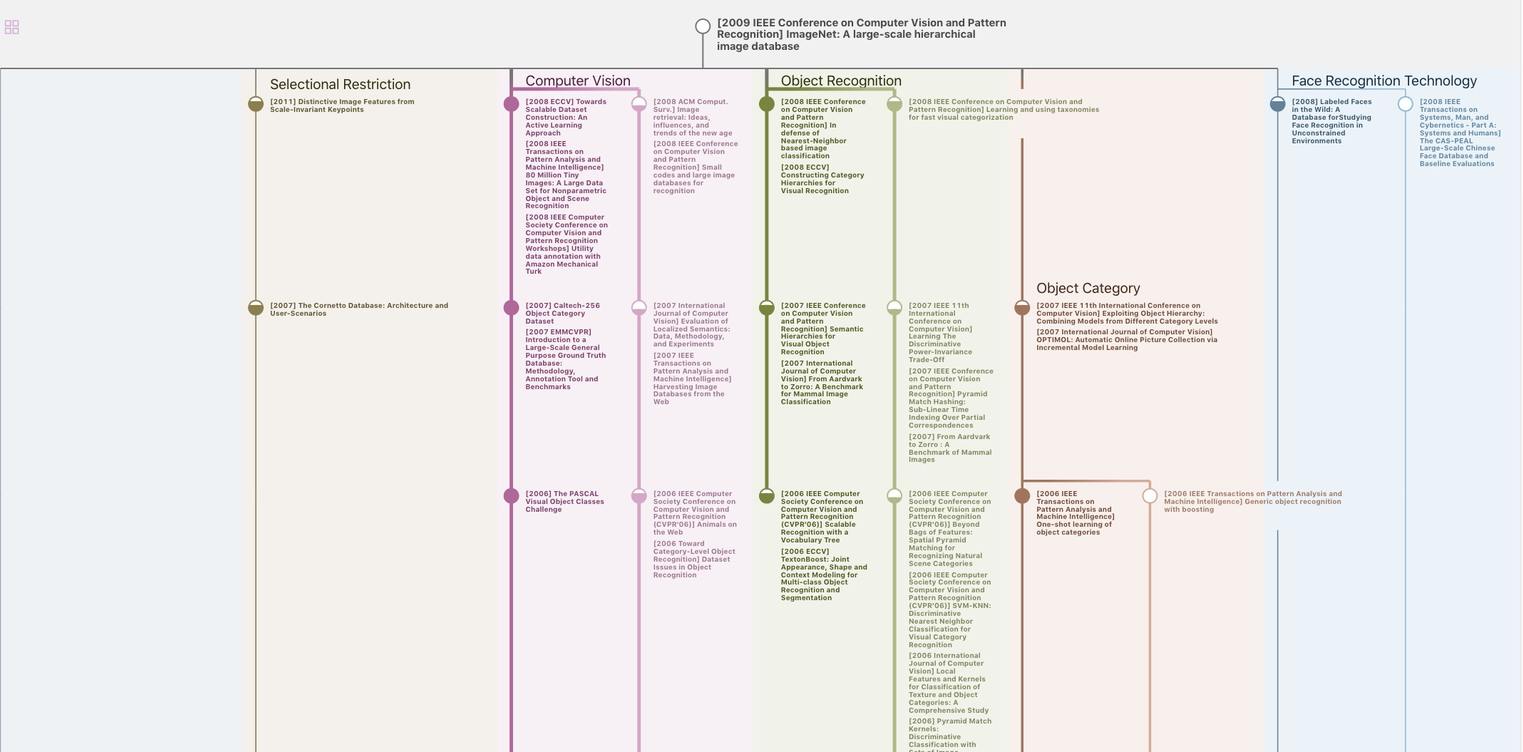
生成溯源树,研究论文发展脉络
Chat Paper
正在生成论文摘要