Employing connectome-based models to predict working memory in multiple sclerosis
bioRxiv (Cold Spring Harbor Laboratory)(2021)
摘要
AbstractBackgroundIndividuals with multiple sclerosis (MS) are vulnerable to deficits in working memory, and the search for neural correlates of working memory in circumscribed areas has yielded inconclusive findings. Given the widespread neural alterations observed in MS, predictive modeling approaches that capitalize on whole-brain connectivity may better capture individual-level working memory in this population.MethodsHere, we applied connectome-based predictive modeling to functional MRI data from working memory tasks in two independent samples with relapsing-remitting MS. In the internal validation sample (ninternal = 36), functional connectivity data were used to train a model through cross-validation to predict accuracy on the Paced Visual Serial Addition Test, a gold-standard measure of working memory in MS. We then tested its ability to predict performance on the N-back working memory task in the external validation sample (nexternal = 36).ResultsThe resulting model successfully predicted working memory in the internal validation sample but did not extend to the external sample. We also tested the generalizability of an existing model of working memory derived in healthy young adults to people with MS. It showed successful prediction in both MS samples, demonstrating its translational potential. We qualitatively explored differences between the healthy and MS models in intra- and inter-network connectivity amongst canonical networks.DiscussionThese findings suggest that connectome-based predictive models derived in people with MS may have limited generalizability. Instead, models identified in healthy individuals may offer superior generalizability to clinical samples, such as MS, and may serve as more useful targets for intervention.Impact StatementWorking memory deficits in people with multiple sclerosis have important consequence for employment, leisure, and daily living activities. Identifying a functional connectivity-based marker that accurately captures individual differences in working memory may offer a useful target for cognitive rehabilitation. Manglani et al. demonstrate machine learning can be applied to whole-brain functional connectivity data to identify networks that predict individual-level working memory in people with multiple sclerosis. However, existing network-based models of working memory derived in healthy adults outperform those identified in multiple sclerosis, suggesting translational potential of brain networks derived in large, healthy samples for predicting cognition in multiple sclerosis.
更多查看译文
关键词
multiple sclerosis,working memory,models,connectome-based
AI 理解论文
溯源树
样例
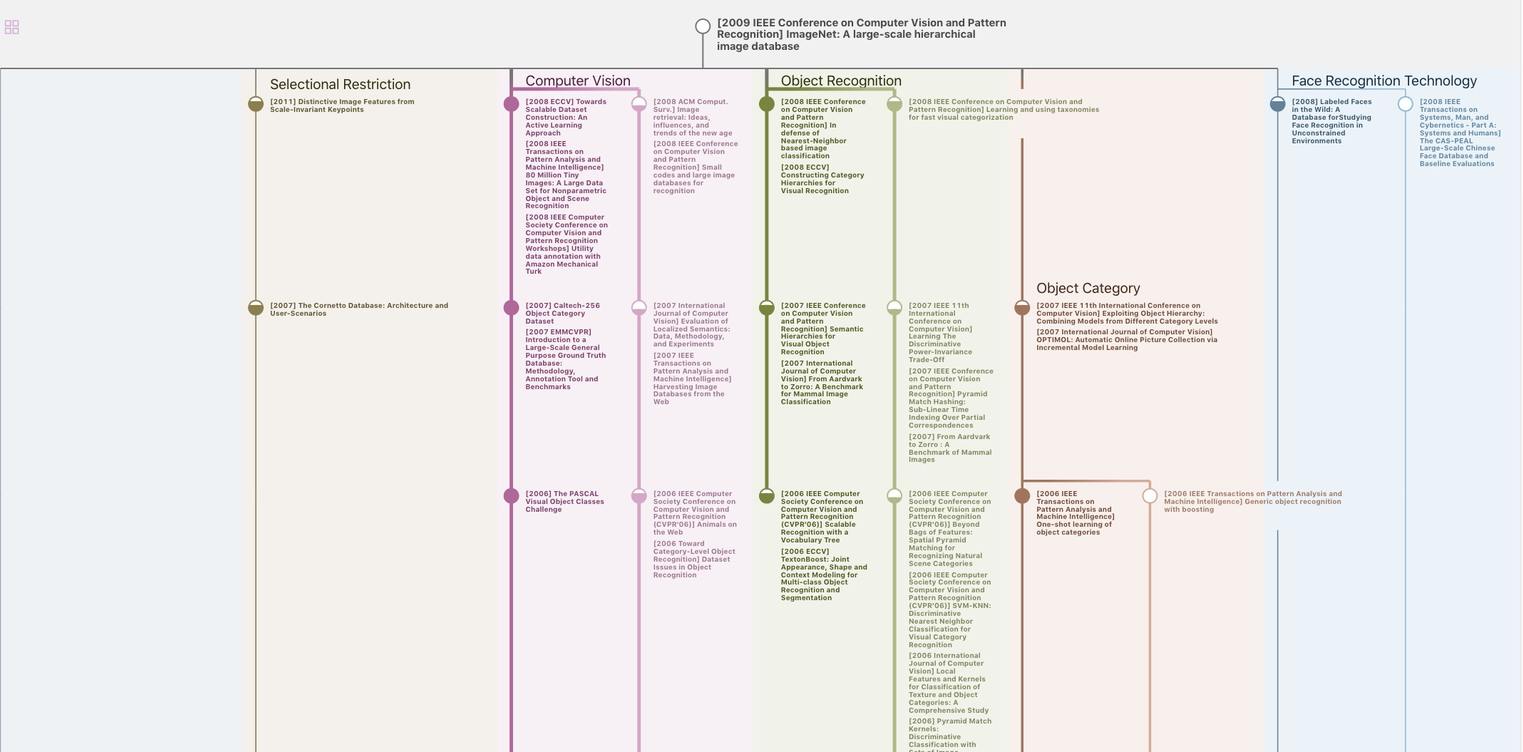
生成溯源树,研究论文发展脉络
Chat Paper
正在生成论文摘要