Detection of Local Mixing in Time-Series Data Using Permutation Entropy
crossref(2021)
摘要
<p>  While it is tempting in experimental practice to seek as high a  data rate as possible, oversampling can become an issue if one takes measurements too densely.  These effects can take many  forms, some of which are easy to detect: e.g., when the data sequence contains multiple copies of the same measured value.  In other situations, as when there is mixing—in the measurement apparatus and/or the system itself—oversampling effects can be harder to detect.  We propose a novel, model-free technique to detect local mixing in time series using an information-theoretic technique called permutation entropy.  By varying the temporal resolution of the calculation and analyzing the patterns in the results, we can determine whether the data are mixed locally, and on what scale.  This can be used by practitioners to choose appropriate lower bounds on scales at which to measure or report data.  After validating this technique on several synthetic examples, we demonstrate its effectiveness on data from a chemistry experiment, methane records from Mauna Loa, and an Antarctic ice core.</p>
更多查看译文
AI 理解论文
溯源树
样例
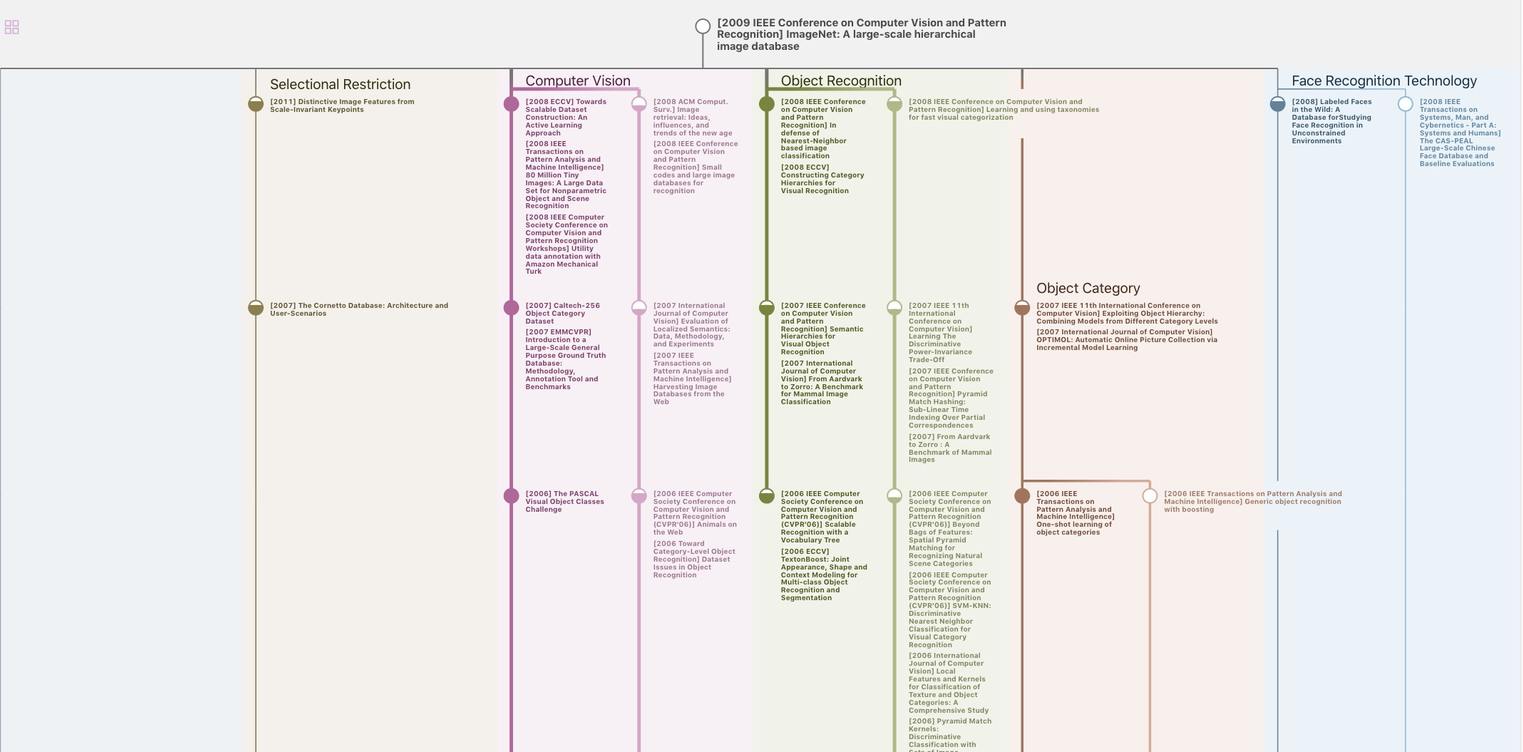
生成溯源树,研究论文发展脉络
Chat Paper
正在生成论文摘要