An Improved Residual-Network Model-based Conditional Generative Adversarial Network Plantar Pressure Image Classification: A Comparison of Normal, Planus, and Talipes Equinovarus Feet
Research Square (Research Square)(2021)
摘要
Abstract The number of layers of deep learning (DL) increases, and following the performance of computing nodes improvement, the output accuracy of deep neural networks (DNN) faces a bottleneck problem. The resident network (RN) based DNN model was applied to address these issues recently. This paper improved the RN and developed a rectified linear unit (ReLU) based conditional generative adversarial nets (cGAN) to classify plantar pressure images. A foot scan system collected the plantar pressure images, in which normal (N), planus (PL), and talipes equinovarus feet (TE) data-sets were acquired subsequently. The 9-foot types named N, PL, TE, N-PL, N-TE, PL-N, PL-TE, TE-N, and TE-PL were classified using the proposed DNN models, named resident network-based conditional generative adversarial nets (RNcGAN). It improved the RN structure firstly and the cGAN system hereafter. In the classification of plantar pressure images, the pixel-level state matrix can be direct as an input, which is different from the previous image classification task with image reduction and feature extraction. cGAN can directly output the pixels of the image without any simplification. Finally, the model achieved better results in the evaluation indicators of accuracy (AC), sensitivity (SE), and F1-measurement (F1) by comparing to artificial neural networks (ANN), k-nearest neighbor (kNN), Fast Region-based Convolution Neural Network (Fast R-CNN), visual geometry group (VGG16), scaled-conjugate-gradient convolution neural networks (SCG-CNN), GoogleNet, AlexNet, ResNet-50-177, and Inception-v3. The final prediction of class accuracy is 95.17%. Foot type classification is vital for producing comfortable shoes in the industry.
更多查看译文
关键词
generative adversarial,talipes equinovarus feet,pressure,classification,residual-network,model-based
AI 理解论文
溯源树
样例
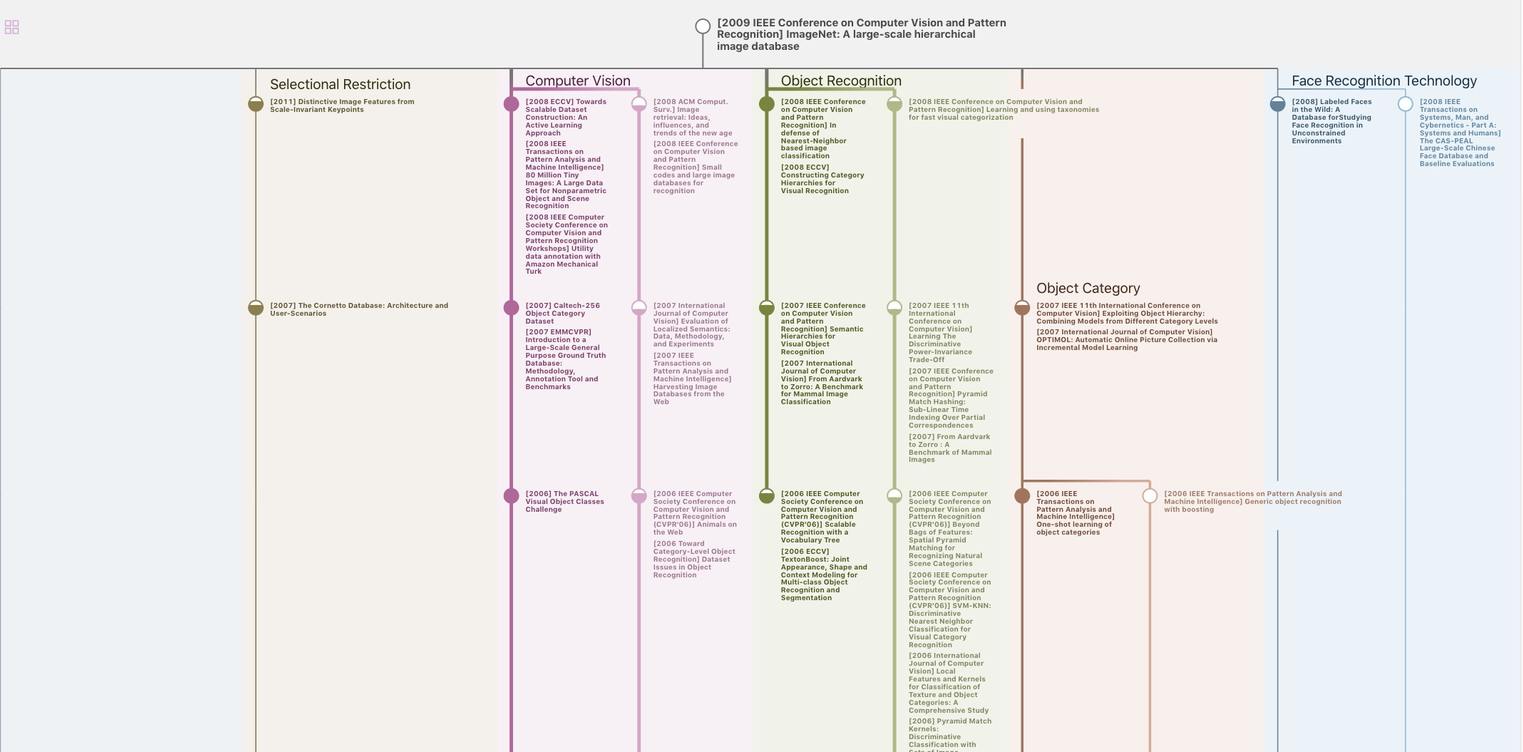
生成溯源树,研究论文发展脉络
Chat Paper
正在生成论文摘要