Tapping the Potential of Earth Observation - Calving Front Detection in SAR Images using Deep Learning Techniques
crossref(2021)
摘要
<p>The calving fronts of lake or marine terminating glaciers provide information about the state of glaciers. A change in its position can affect the flow of the entire glacier system, and the loss of ice mass as icebergs calve-off and discharge into the ocean has a multi-scale impact on the global hydrological cycle. The calving fronts can be manually delineated in Synthetic Aperture Radar (SAR) images. However, this is a time-consuming, tedious and expensive task. As deep learning approaches have achieved tremendous success in various disciplines, such as medical image processing and computer vision, the project Tapping the Potential of Earth Observation (TAPE) is amongst other things dedicated to applying deep learning techniques to calving front detection. So far, all our experiments have employed U-Net based architectures, as the U-Net is state-of-the-art in semantic image segmentation. A major challenge of front detection is the class imbalance: The front has significantly fewer pixels than the remaining parts of the SAR image. Hence, we developed variants of the U-Net specifically addressing this challenge including an Attention U-Net, a probabilistic Bayesian U-Net, as well as a U-Net with a distance map-based binary cross-entropy (BCE) loss function and a Mathews correlation coefficient (MCC) as early stopping criterion. In future work, we plan to investigate multi-task learning and a segmentation of the SAR image into different classes (i.e. ocean, glacier and rocks) to enhance the quality and efficiency of the front detection.</p>
更多查看译文
AI 理解论文
溯源树
样例
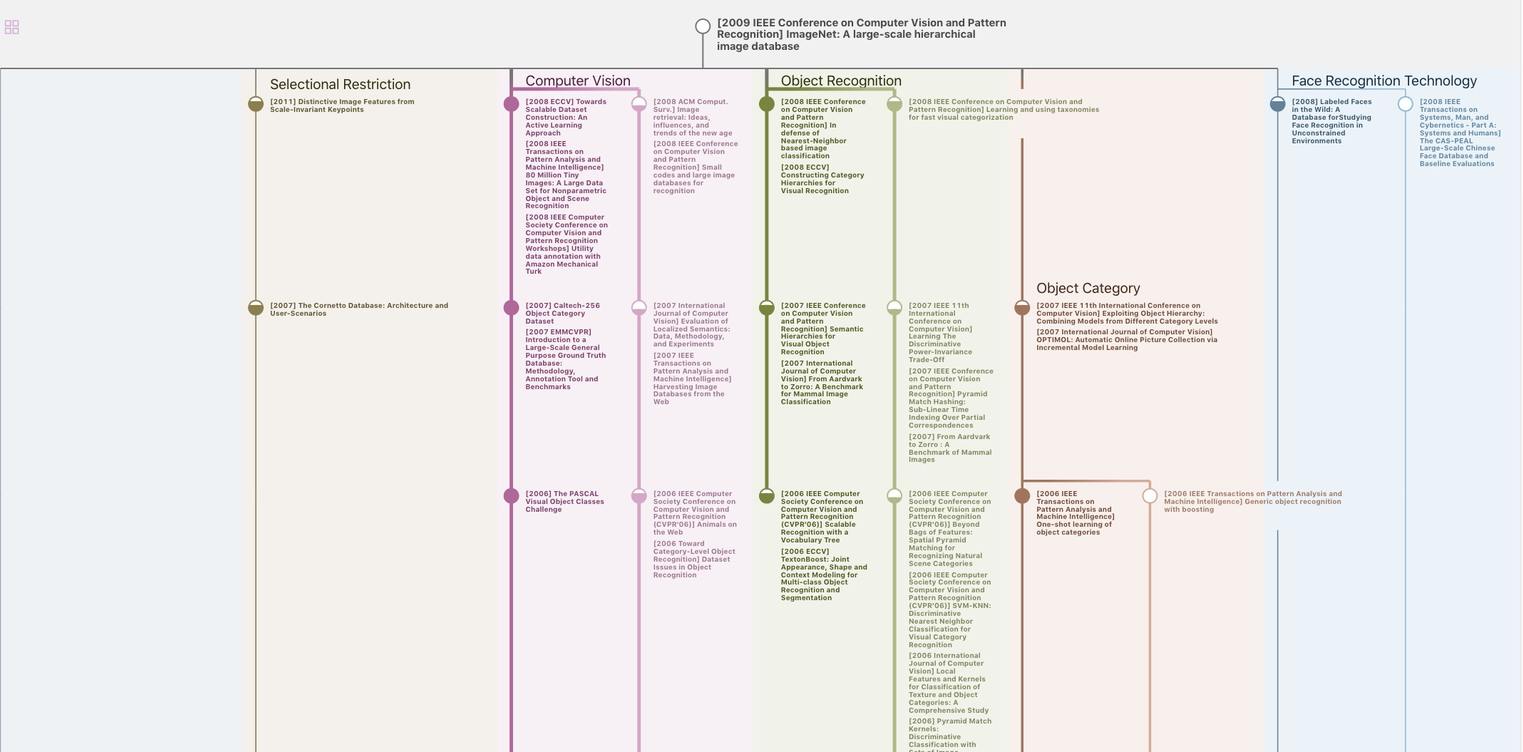
生成溯源树,研究论文发展脉络
Chat Paper
正在生成论文摘要