Thermal Comfort Control on Sustainable Building via Data-Driven Robust Model Predictive Control
2023 AMERICAN CONTROL CONFERENCE, ACC(2023)
摘要
While implementing renewable energy systems and model predictive control (MPC) could reduce non-renewable energy consumption, one challenge to building climate control using MPC is the weather forecast uncertainty. In this work, we propose a data-driven robust model predictive control (DDRMPC) framework to address climate control of a sustainable building with renewable hybrid energy systems under weather forecast uncertainty. The control and energy system configurations include heating, ventilation, and air conditioning, geothermal heat pump, photovoltaic panel, and electricity storage battery. Historical weather forecast and measurement data are gathered from the weather station to identify the forecast errors and for the use of uncertainty set construction. The data-driven uncertainty sets are constructed with multiple machine learning techniques, including principal component analysis with kernel density estimation, K-means clustering coupled with PCA and KDE, density-based spatial clustering of applications with noise, and the Dirichlet process mixture model. Lastly, a data-driven robust optimization problem is developed to obtain the optimal control inputs for a building with renewable energy systems. A case study on controlling a building with renewable energy systems located on the Cornell University campus is used to demonstrate the advantages of the proposed DDRMPC framework.
更多查看译文
AI 理解论文
溯源树
样例
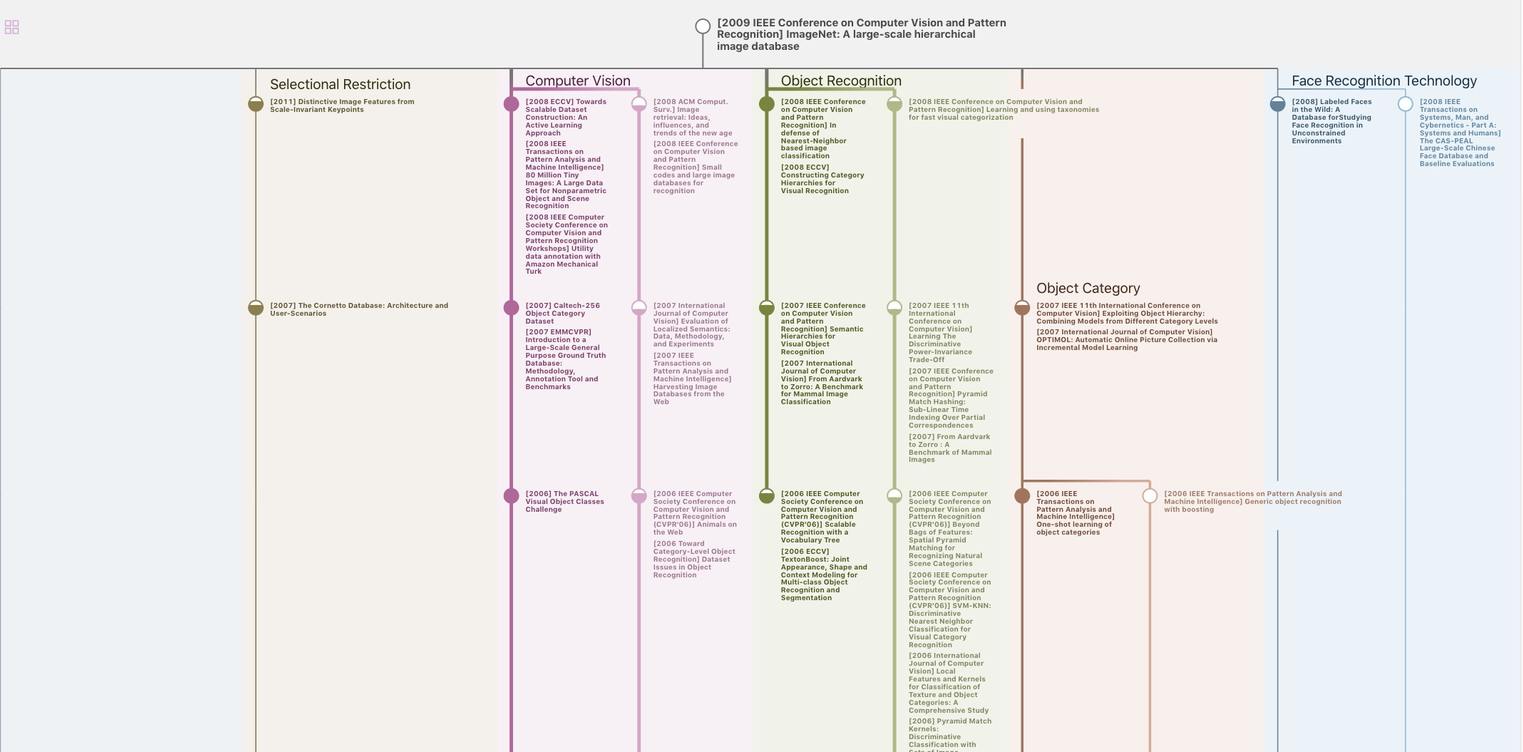
生成溯源树,研究论文发展脉络
Chat Paper
正在生成论文摘要