Federated reinforcement learning for generalizable motion planning
2023 AMERICAN CONTROL CONFERENCE, ACC(2023)
摘要
This paper considers the problem of learning a control policy that generalize well to novel environments given a set of sample environments. We develop a federated learning framework that enables collaborative learning of multiple learners and a centralized server without sharing their raw data. In each iteration, each learner uploads its local control policy and the corresponding estimated normalized arrival time to the server, which then computes the global optimum among the learners and broadcasts the optimal policy to the learners. Each learner then selects between its local control policy and that from the server for next iteration. By leveraging generalization error, our analysis shows that the proposed framework is able to provide generalization guarantees on arrival time and safety as well as consensus at global optimal value in the limiting case. Monte Carlo simulation is conducted for evaluation.
更多查看译文
AI 理解论文
溯源树
样例
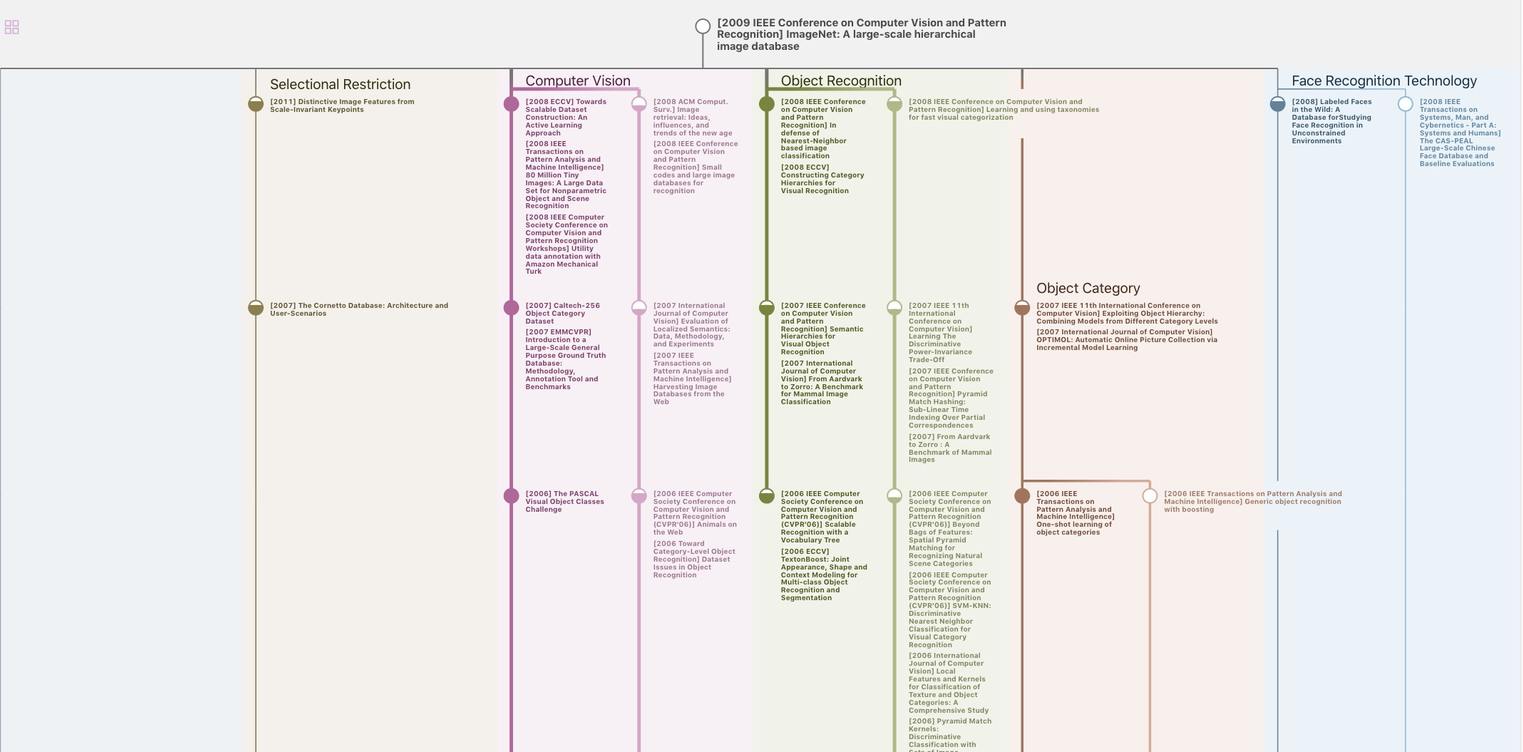
生成溯源树,研究论文发展脉络
Chat Paper
正在生成论文摘要