Evaluating risk detection methods to uncover ontogenic-mediated adverse drug effect mechanisms in children
medRxiv (Cold Spring Harbor Laboratory)(2021)
摘要
AbstractBackgroundIdentifying adverse drugs effects (ADEs) in children is essential for preventing disability and death from marketed drugs. At the same time, however, detection is challenging due to dynamic biological processes during growth and maturation, called ontogeny, that alter pharmacokinetics and pharmacodynamics. As a result, current data mining methodologies have been limited to event surveillance and have not focused on investigating adverse event mechanisms. There is an opportunity to design data mining methodologies to identify and evaluate drug event patterns within observational databases for ontogenic-mediated adverse event mechanisms. The first step of which is to establish statistical models that can identify temporal trends of adverse effects across childhood.ResultsUsing simulation, we evaluated a population stratification method (the proportional reporting ratio or PRR) and a population modeling method (the generalized additive model or GAM) to identify and quantify ADE risk at varying reporting rates and dynamics. We found that GAMs showed improved performance over the PRR in detecting dynamic drug event reporting across child developmental stages. Moreover, GAMs exhibited normally distributed and robust ADE risk estimation at all development stages by sharing information across child development stages.ConclusionsOur study underscores the opportunity for using population modeling techniques, which leverages drug event reporting across development stages, to identify adverse drug effect risk resulting from ontogenic mechanisms.
更多查看译文
关键词
adverse drug effect mechanisms,risk detection methods,children,ontogenic-mediated
AI 理解论文
溯源树
样例
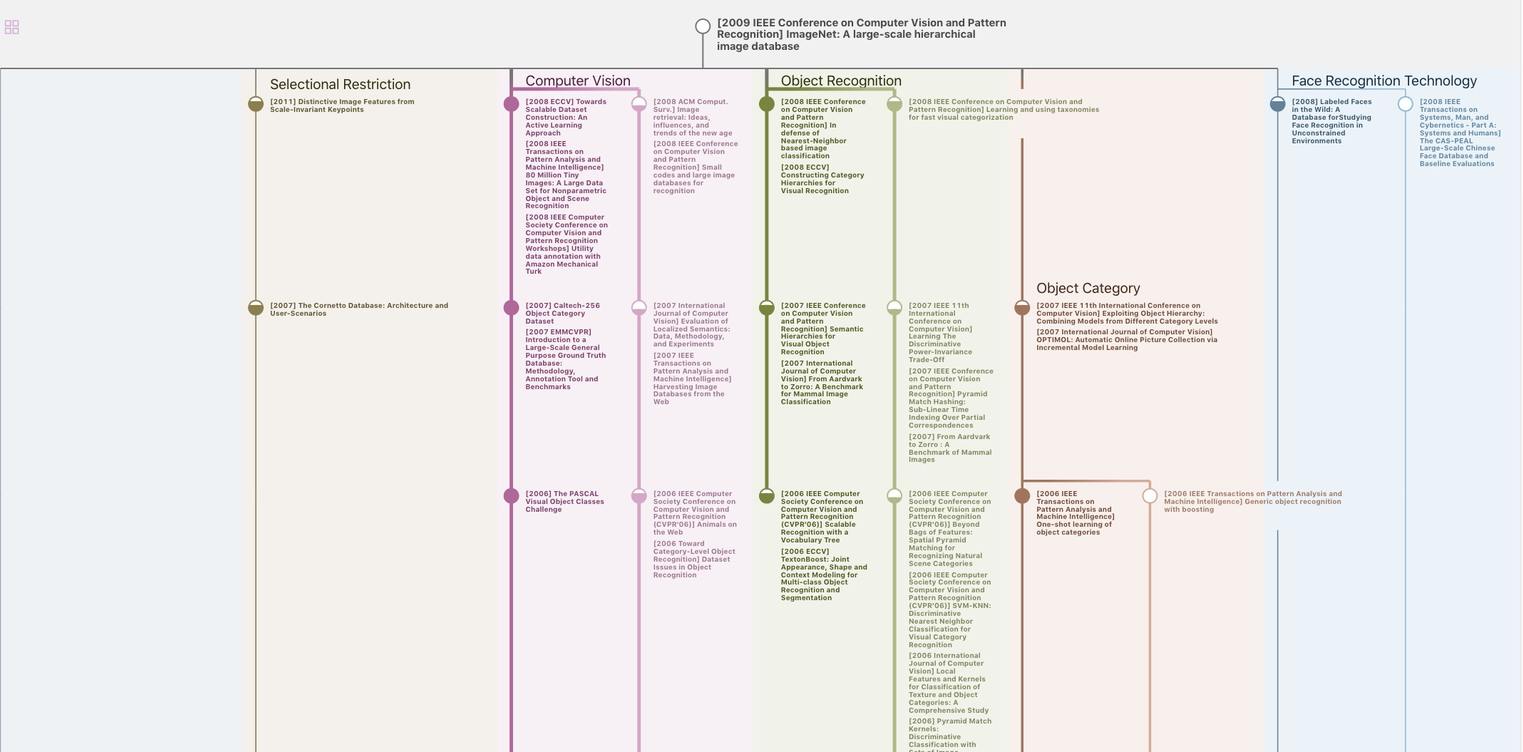
生成溯源树,研究论文发展脉络
Chat Paper
正在生成论文摘要