Deep Alternating Direction Multiplier Method Network for Event Detection
Journal of Electronics & Information Technology(2023)
摘要
Considering the Event Detection Problem (EDP) in the large-scale Wireless Sensor Network (WSN), the conventional methods rely generally on some prior information, which obstacles the actual application. In this paper, a deep learning-based algorithm, named as Alternating Direction Multiplier Method Network (ADMM-Net), is proposed for the EDP. Firstly, the low rank and sparse matrix decomposition is adopted to capture the spatial-temporal correlation of events. After that, the EDP is formulated as a constrained optimization problem and solved by the Alternating Direction Multiplier Method (ADMM). However, the optimization algorithm suffers from low convergence. Besides, the algorithm's performance relies heavily on the careful selection of prior parameters. By adopting the conception of "unfolding" in deep learning field, a deep learning network which is named ADMM-Net, is proposed for the EDP in this paper. The ADMM-Net is obtained by unfolding the ADMM algorithm. The ADMM-Net is with fixed layers, whose parameters can be trained via supervised learning. No prior information is required. Compared to the conventional methods, the proposed ADMM-Net does not require any prior information while enjoying fast convergence. Simulation results on both synthesis and realistic datasets verify the effectiveness of the proposed ADMM-Net.
更多查看译文
关键词
Event detection,Wireless Sensor Networks(WSN),Spatial-temporal correlation,Low rank and sparse matrix decomposition,Deep learning,Alternating Direction Multiplier Method Network(ADMM-Net)
AI 理解论文
溯源树
样例
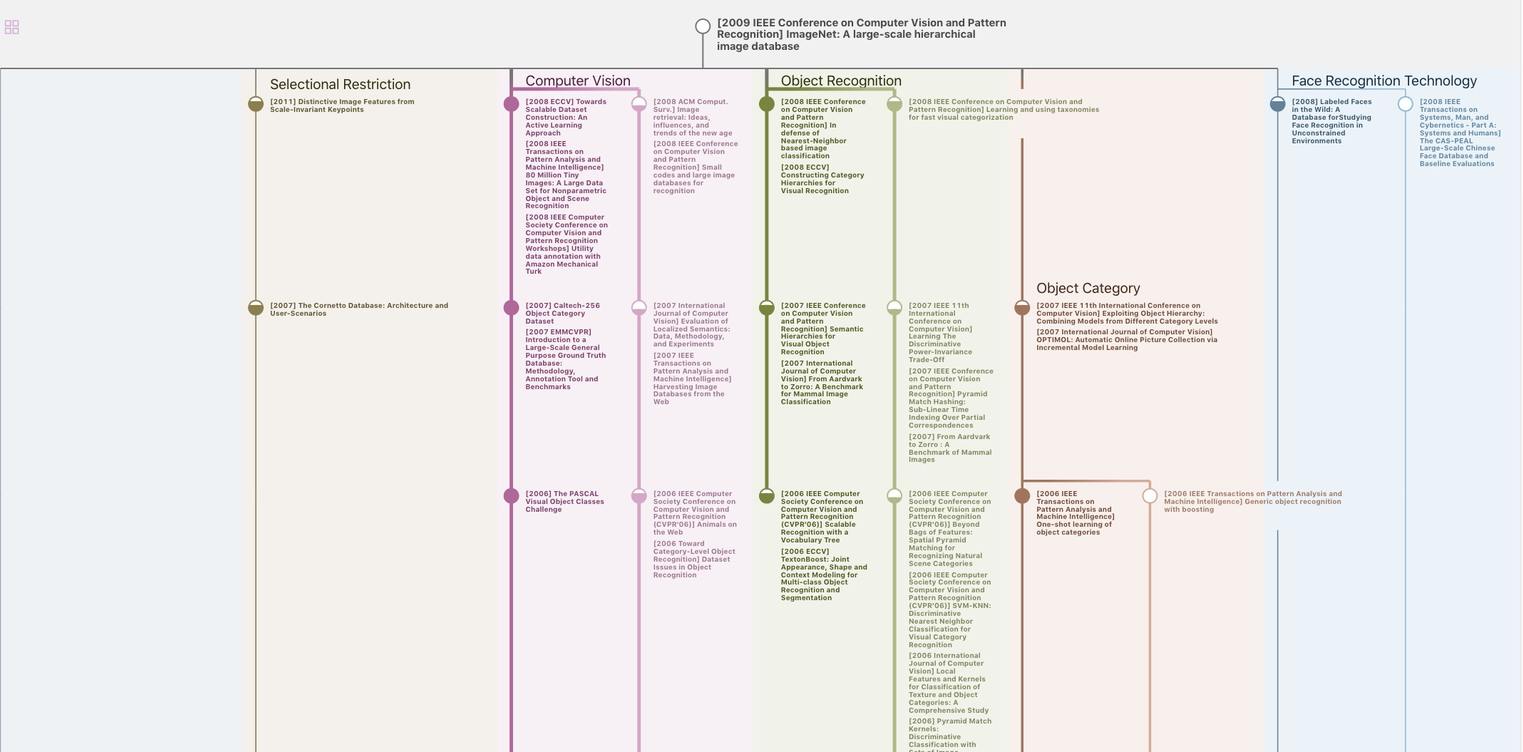
生成溯源树,研究论文发展脉络
Chat Paper
正在生成论文摘要