Ariadne: Synthetic Long Read Deconvolution Using Assembly Graphs
crossref(2021)
摘要
AbstractBackgroundDe novo assemblies are critical for capturing the genetic composition of complex samples. Synthetic Long Read sequencing techniques such as 10x Genomics’ Linked-Reads, UST’s TELL-Seq, Loop Genomics’ LoopSeq, and BGI’s Long Fragment Read combines 3′ barcoding with standard short-read sequencing to expand the range of linkage resolution from hundreds to tens of thousands of base-pairs. The application of SLR sequencing to genome assembly has demonstrated that barcoding-based technologies balance the tradeoffs between long-range linkage, per-base coverage, and costs. However, multiple long fragments may be associated with the same 3′ nucleotide-based unique molecular identifier (UMI). The lack of a 1:1 correspondence between a long fragment and a UMI, in conjunction with low sequencing depth, confounds the assignment of linkage between short-reads.ResultsWe introduce Ariadne, a novel SLR deconvolution algorithm based on assembly graphs, that can be used to extract single-species read-sets from a large SLR dataset. Ariadne deconvolution of SLR clouds increases the proportion of read clouds containing only reads from a single fragment by up to 37.5-fold. Using these enhanced read clouds in de novo assembly significantly improves assembly contiguity and the size of the largest aligned blocks in comparison to the non-deconvolved read clouds.ConclusionsIntegrating Ariadne into the post-processing pipeline for SLR technologies increases the quality of de novo assembly for complex populations, such as microbiomes.
更多查看译文
AI 理解论文
溯源树
样例
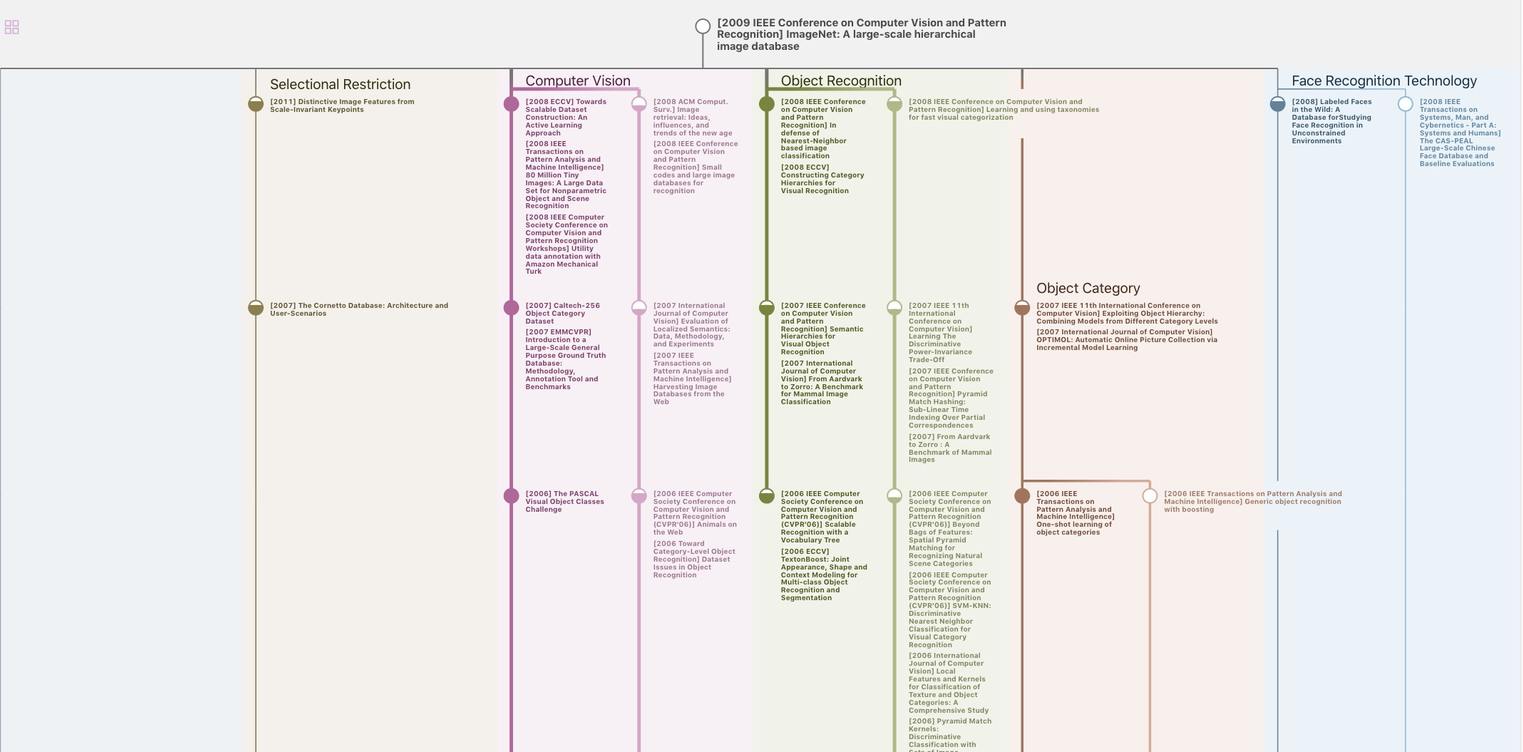
生成溯源树,研究论文发展脉络
Chat Paper
正在生成论文摘要