Test-Time Augmentation for Document Image Binarization.
IbPRIA(2023)
摘要
Document binarization is a well-known process addressed in the document image analysis literature, which aims to isolate the ink information from the background. Current solutions use deep learning, which requires a great amount of annotated data for training robust models. Data augmentation is known to reduce such annotation requirements, and it can be used in two ways: during training and during prediction. The latter is the so-called Test Time Augmentation (TTA), which has been successfully applied for general classification tasks. In this work, we study the application of TTA for binarization, a more complex and specific task. We focus on cases with a severe scarcity of annotated data over 5 existing binarization benchmarks. Although the results report certain improvements, these are rather limited. This implies that existing TTA strategies are not sufficient for binarization, which points to interesting lines of future work to further boost the performance.
更多查看译文
关键词
document image binarization,test-time
AI 理解论文
溯源树
样例
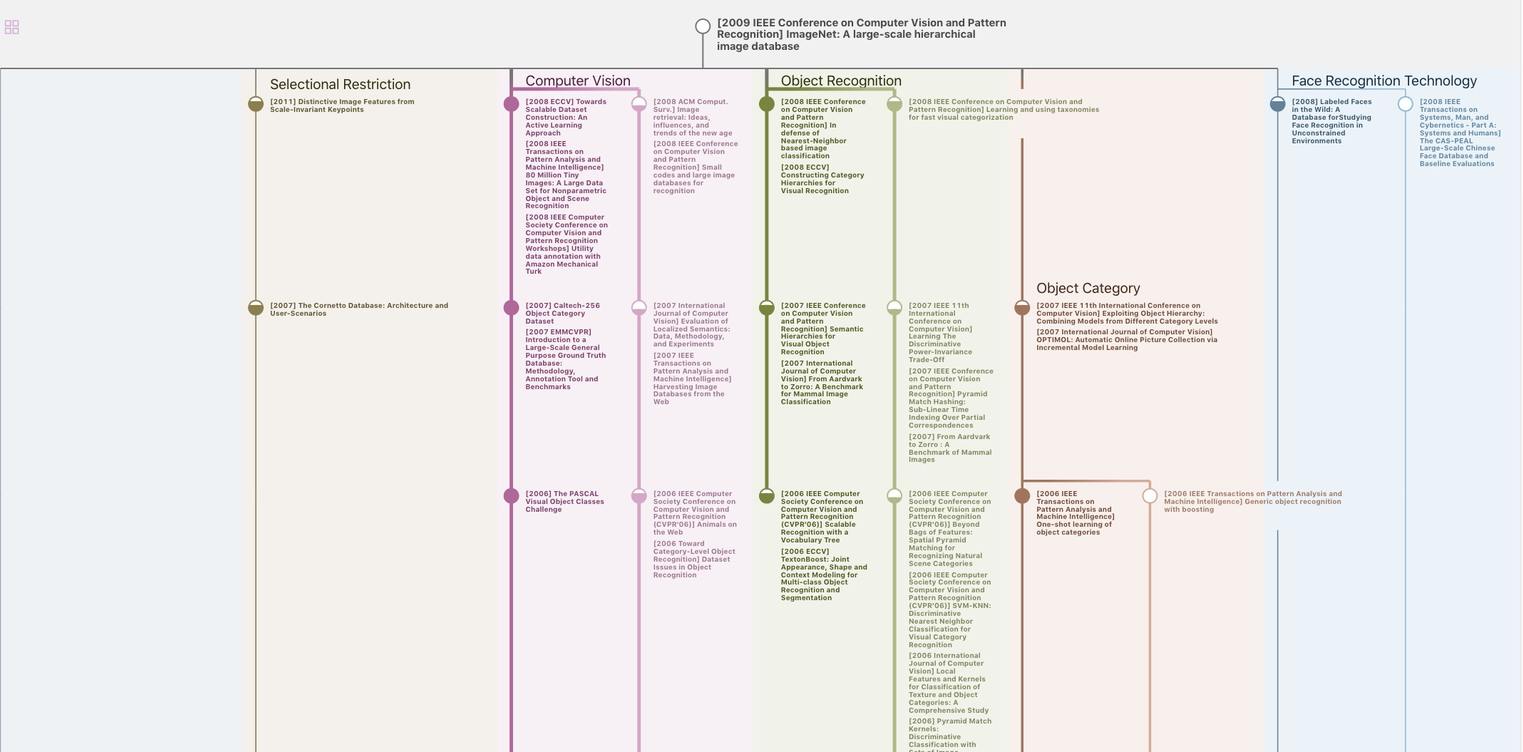
生成溯源树,研究论文发展脉络
Chat Paper
正在生成论文摘要