A Markov Framework for Learning and Reasoning About Strategies in Professional Soccer.
J. Artif. Intell. Res.(2023)
摘要
Strategy-optimization is a fundamental element of dynamic and complex team sports such as soccer, American football, and basketball. As the amount of data that is collected from matches in these sports has increased, so has the demand for data-driven decision-making support. If alternative strategies need to be balanced, a data-driven approach can uncover insights that are not available from qualitative analysis. This could tremendously aid teams in their match preparations. In this work, we propose a novel Markov model-based framework for soccer that allows reasoning about the specific strategies teams use in order to gain insights into the efficiency of each strategy. The framework consists of two components: (1) a learning component, which entails modeling a team's offensive behavior by learning a Markov decision process (MDP) from event data that is collected from the team's matches, and (2) a reasoning component, which involves a novel application of probabilistic model checking to reason about the efficacy of the learned strategies of each team. In this paper, we provide an overview of this framework and illustrate it on several use cases using real-world event data from three leagues. Our results show that the framework can be used to reason about the shot decision-making of teams and to optimise the defensive strategies used when playing against a particular team. The general ideas presented in this framework can easily be extended to other sports.
更多查看译文
关键词
markov framework,strategies,learning,reasoning
AI 理解论文
溯源树
样例
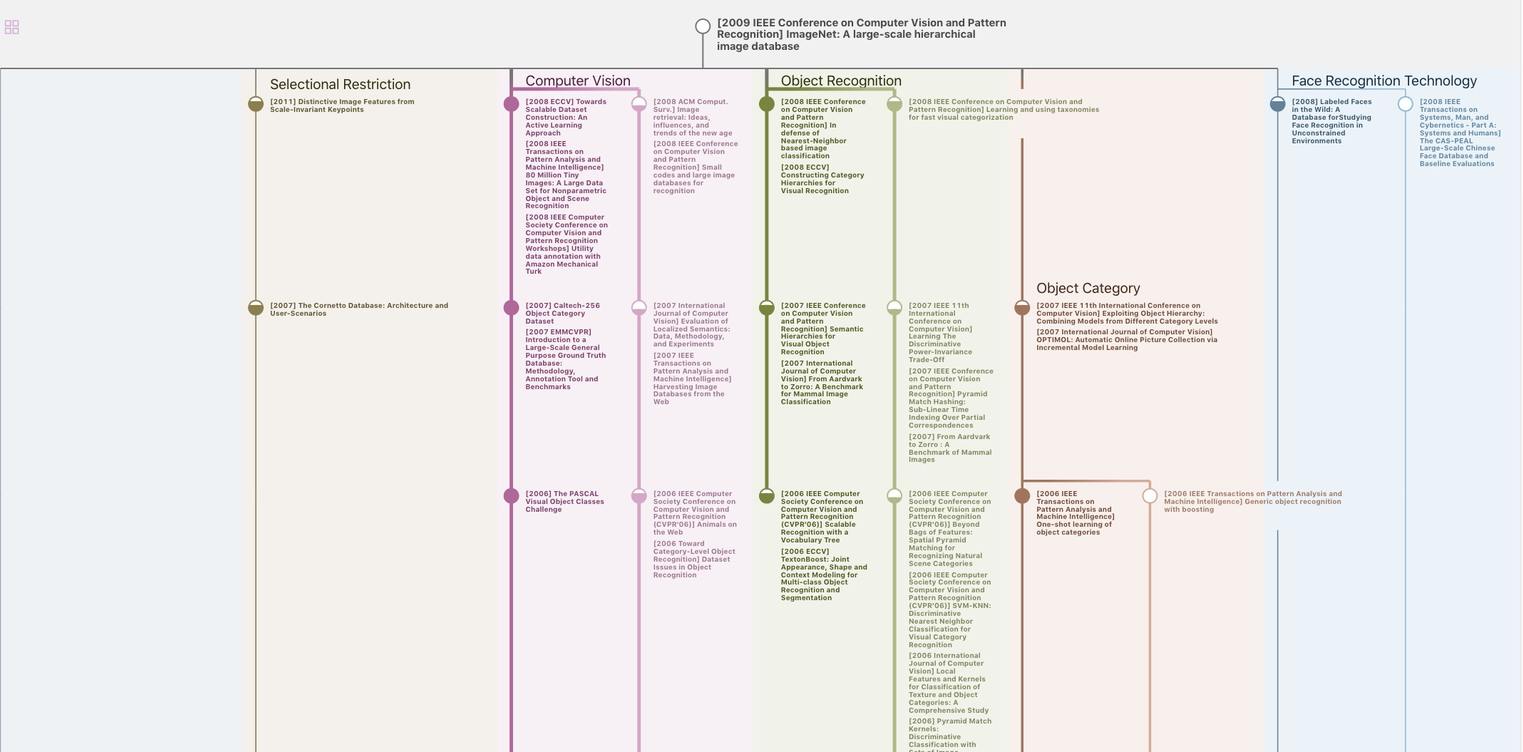
生成溯源树,研究论文发展脉络
Chat Paper
正在生成论文摘要