Run-Time Probabilistic Model Checking for Failure Prediction: A Smart Lift Case Study.
WF-IoT(2022)
摘要
Modern smart systems are powered by cyber-physical systems integrating sensor networks with service-oriented architecture to automate their operations. Control algorithms deployed on smart systems are now driven by connected sensors with control decisions being made based on the sensor generated data. As sensors tend to be unreliable and prone to failures, this has resulted in the increase of system errors due to the wrong control decisions derived from the faulty sensor readings, thus affecting the performance, safety and quality of the operational tasks. Existing methodologies to evaluate and test such systems do not take into account the complexity and uncertainty exhibited by the underlying sensor networks, and hence not being able to dynamically verify the behaviour of the smart systems at run-time. This paper proposes a novel run-time verification framework combining sensor-level fault detection and system-level probabilistic model checking. This framework rigorously quantifies the trustworthiness of sensor readings, hence enabling formal reasoning for system failure prediction. We evaluated our approach on a passenger lift equipped with sensor networks to monitor its main components continuously. The results indicate that the proposed verification framework involving the quantified sensor's trustworthiness enhances the accuracy of the system failure prediction.
更多查看译文
关键词
Probabilistic Model Checking,Sensor Confidence,Sensor Trustworthiness,Sensor Network
AI 理解论文
溯源树
样例
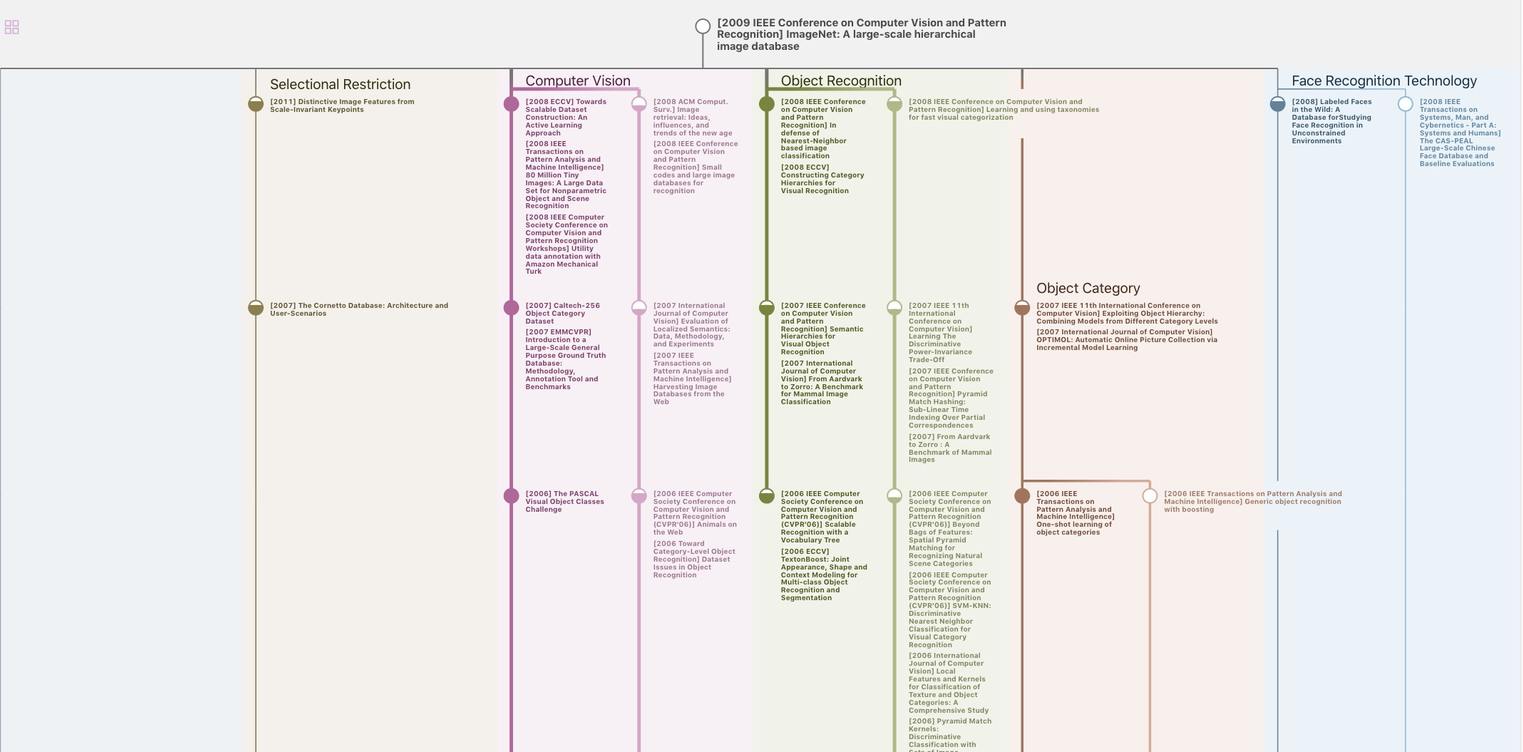
生成溯源树,研究论文发展脉络
Chat Paper
正在生成论文摘要