Representation Learning for Semantic Scene Understanding
HHAI 2023: AUGMENTING HUMAN INTELLECT(2023)
摘要
Recent advances in semantic scene understanding have underscored its growing significance in the field of computer vision. Enhanced representations can be achieved by incorporating semantic information derived from textual data and applying it to generative models for scene modeling. Nevertheless, the features extracted from text prompts may not seamlessly model a scene. Scene graphs offer a robust solution to address this challenge, serving as a powerful representation for semantic image generation and manipulation. In this study, we delve into the utilization of scene graphs for this purpose and propose novel methodologies to augment both the representation and learning processes involved in image generation and manipulation. For image generation, we examine meta-learning for producing images in unprecedented scenes and refine the generated images using an autoregressive scene graph generation model. In terms of image manipulation, we put forth a novel self-supervised method that eliminates the need for paired before-and-after data. Additionally, we boost image manipulation performance by disentangling latent and graph representations in a self-supervised manner. By evaluating the efficacy of our proposed approaches on a diverse range of publicly available benchmarks, we demonstrate their superiority, ultimately achieving state-of-the-art performance in the domain of semantic image generation and manipulation.
更多查看译文
关键词
Scene Graphs,Representation Learning,Image Generation and Manipulation
AI 理解论文
溯源树
样例
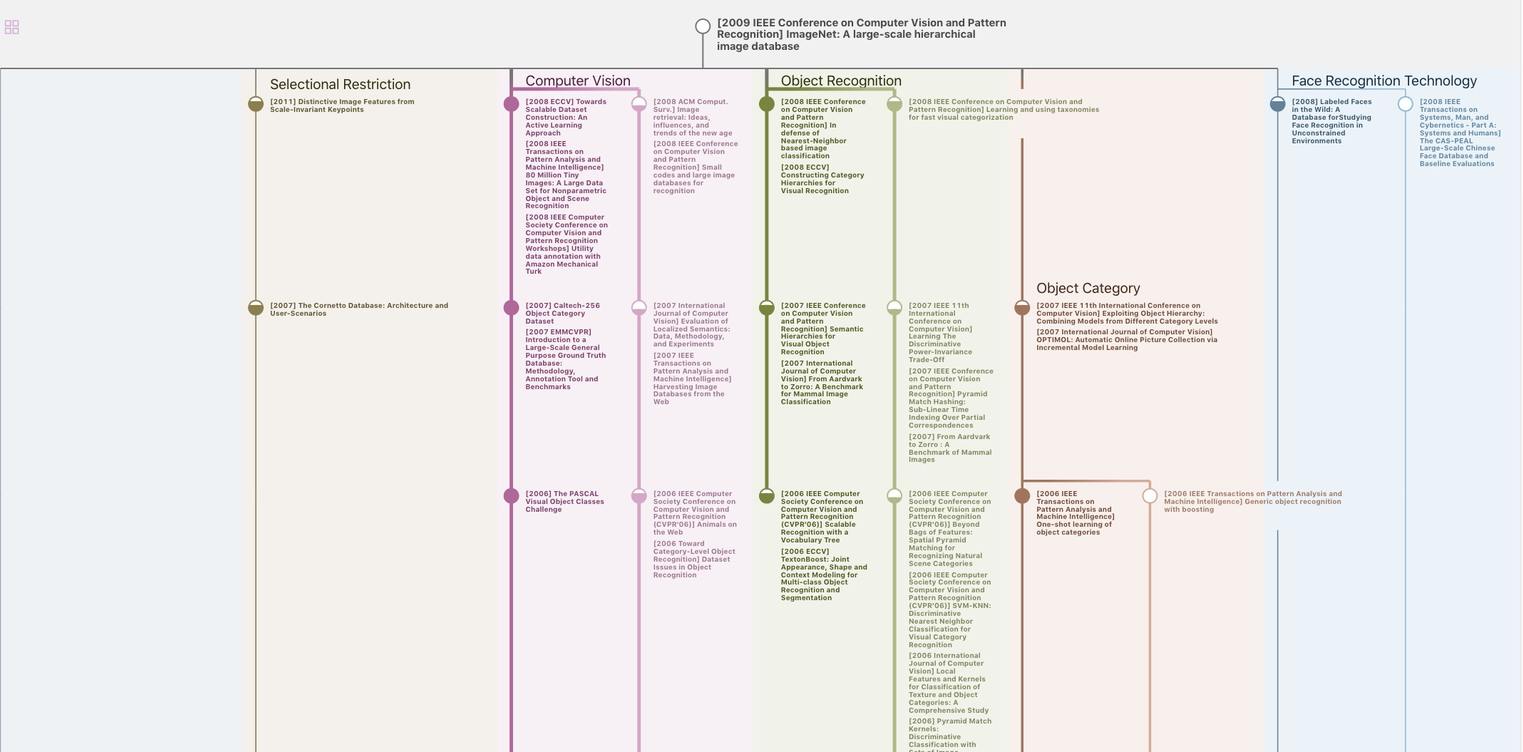
生成溯源树,研究论文发展脉络
Chat Paper
正在生成论文摘要