Explaining Autonomous Drones: An XAI Journey
crossref(2021)
摘要
COGLE (COmmon Ground Learning and Explanation) is an explainable artificial intelligence (XAI) system for autonomous drones that deliver supplies in mountainous areas to field units. The drone missions have risks that vary with topography, flight decisions, and mission goals in a simulated environment. Users must determine which AI-controlled drone is better for a mission. Narrative explanations identify the advantages of a drone’s plan (“What?”) and reasons that the better drone is able to do them (“Why?”). Visual explanations highlight risks from obstacles that users may have overlooked (“Where?”). A model induction user study showed that post-decision explanations produced a small effect on the participants’ abilities to identify the better of two imperfect drones and their plans for a mission, but they did not teach participants to judge the multiple success factors in complex missions as well as the AI pilots. In a decision support variation of the task, users would receive pre-decision explanations to help them to decide when to trust the XAI’s decision. In a fielded XAI application, every drone available for a mission may lack some competencies. We created a proof-of-concept demonstration of automatic ways to combine knowledge from multiple imperfect AIs to get better solutions that the individual AIs do not find on their own. This paper reports on the research challenges, technical approach, and findings of the project and also reflects on the multidisciplinary journey that we took.
更多查看译文
AI 理解论文
溯源树
样例
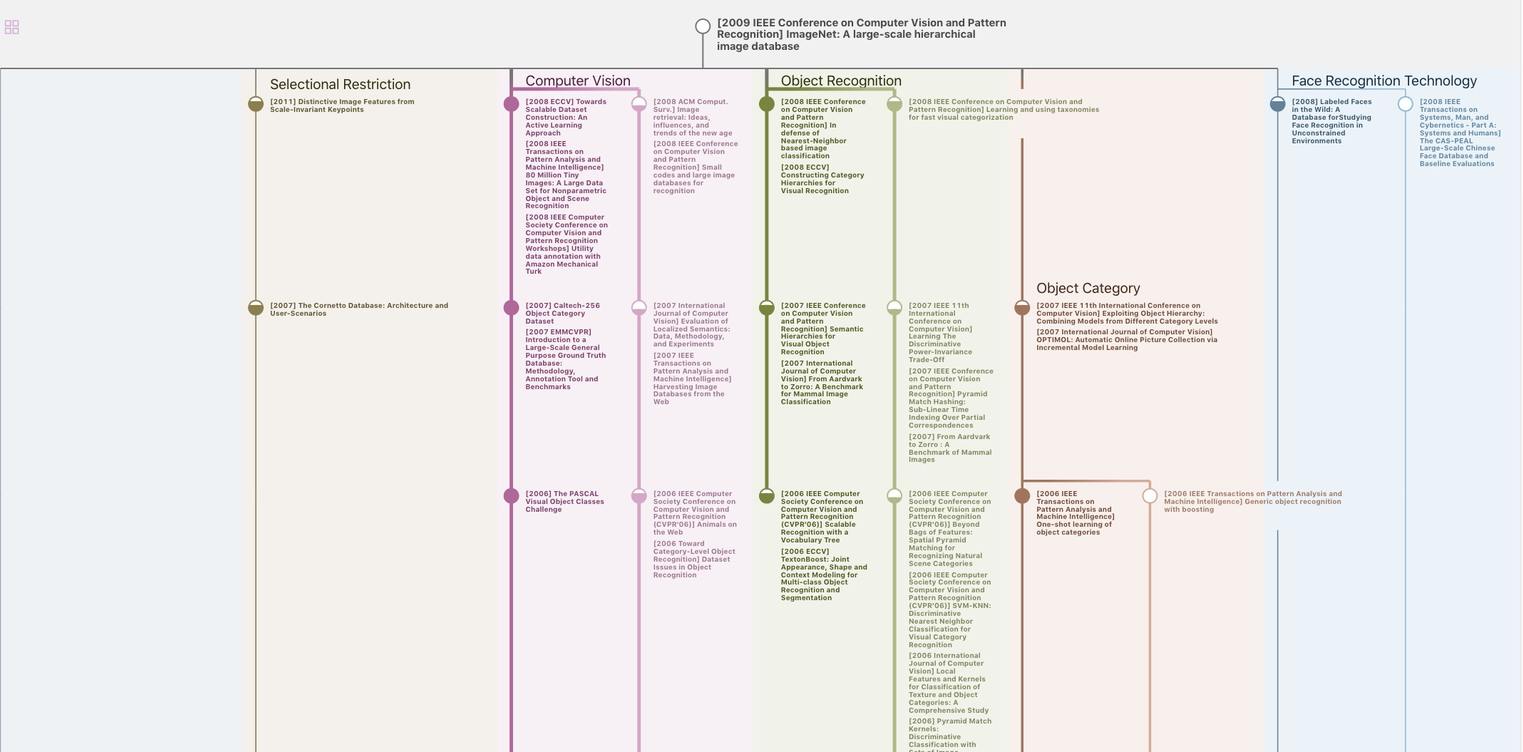
生成溯源树,研究论文发展脉络
Chat Paper
正在生成论文摘要