Supporting Information for "Large-scale Surface Shape Sensing with Learning-based Computational Mechanics"
crossref(2021)
摘要
Proprioception, the ability to perceive one’s own configuration and movement in space, enables organisms to safely and accurately interact with their environment and each other. The underlying sensory nerves that make this possible are highly dense and use sophisticated communication pathways to propagate signals from nerves in muscle, skin and joints to the central nervous system wherein the organism can process and react to stimuli. In a step forward to realize robots with such perceptive capability, we propose a flexible sensor framework that incorporates a novel hybrid modeling strategy, taking advantage of computational mechanics and machine learning. We implement the sensor framework on a large, thin and flexible sensor that transforms sparsely distributed strains into continuous surface shape. Finite element (FE) analysis is utilized to determine sensor design parameters, while an FE model is built to enrich the morphological data used in the supervised training to achieve continuous surface reconstruction. A mapping between the local strain data and the enriched surface data is subsequently trained using ensemble learning. This hybrid approach enables real-time, robust and high-order surface shape reconstruction. The sensing performance is evaluated in terms of accuracy, repeatability, and feasibility with numerous scenarios, which has not been demonstrated and reported on such a large-scale (A4-paper-size) sensor before.
更多查看译文
AI 理解论文
溯源树
样例
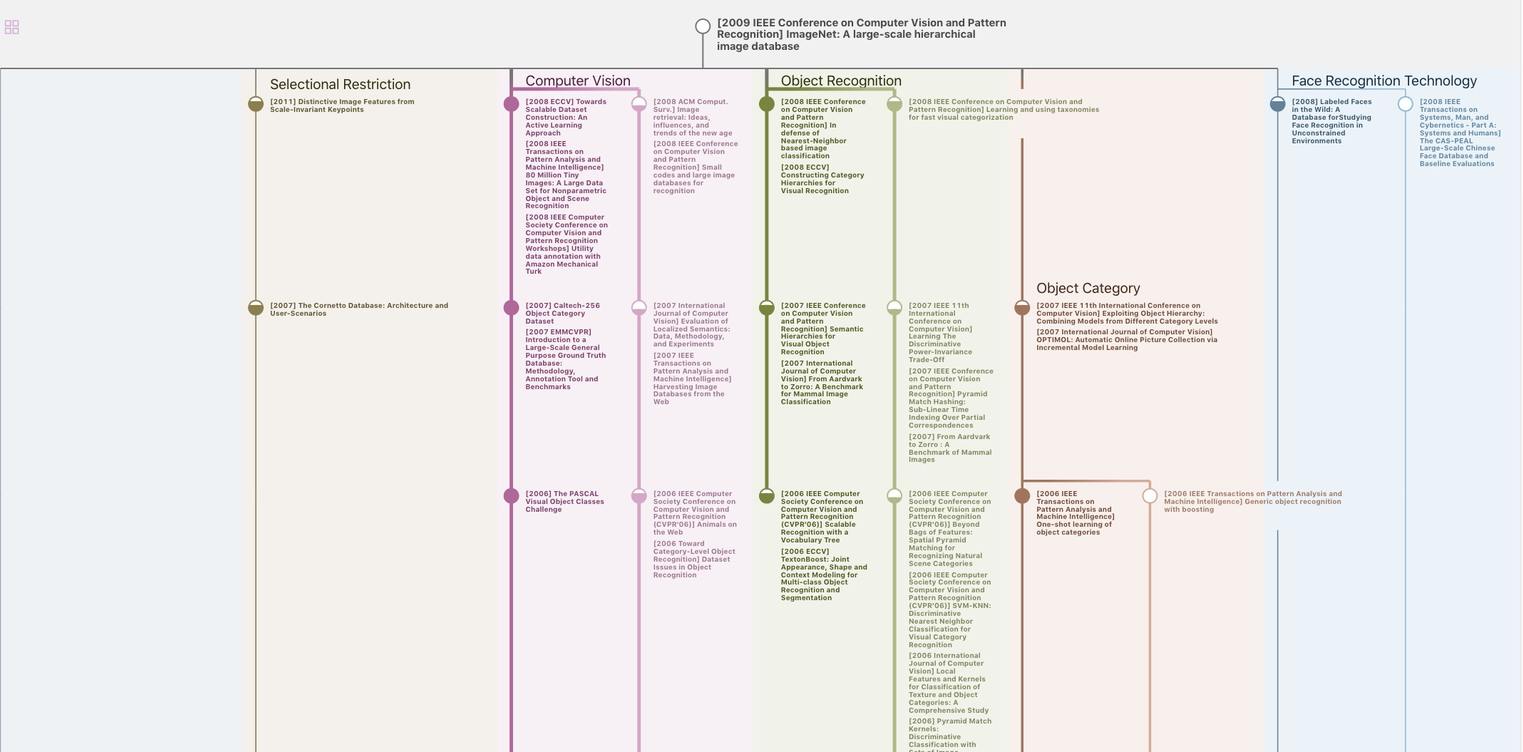
生成溯源树,研究论文发展脉络
Chat Paper
正在生成论文摘要