A New Approach for In Silico Prediction of Oral Drug Concentration Profiles in Human for Drug Candidates Lack Experimental Pharmacokinetic Data
crossref(2021)
摘要
Abstract The purpose of this study is to develop a novel protocol to preliminarily predict the concentration profiles of a target drug based on the PBPK model of a structurally similar template drug by combining two software for PBPK modeling, the SimCYP simulator and ADMET Predictor. The method was evaluated by utilizing 13 drug pairs which come from 18 drugs in the built-in database of the SimCYP software. All drug pairs have their Tanimoto scores no less than 0.5. As each drug in a drug pair can serve both the target and template roles, in total, there are 26 sets studied in this work. Three versions (V1, V2 and V3) of models for the target drug were constructed by replacing the corresponding parameters of the template drug step by step with those predicted by ADME Predictor for the target drug. Normalized RMSE (NRMSE) were introduced for the evaluation of the model performance. We found that the performance of the three versions of models depends on structure similarity of the drug pairs. For Group I drug pairs (TS ≤ 0.7), V2 and V3 perform better than V1 in term of NRMSE; for Group II drug pairs (0.7 < TS ≤ 0.9), 8 out of 10 V3 models have NRMSE lower than 0.2, the cutoff we applied to judge if the simulated C-T curve is satisfactory or not. Obviously, V3 outperforms the V1 and V2 versions. For the two drug pairs belong to Group III (TS > 0.9), V2 outperforms V1 and V3, suggesting more unnecessary replacement can lower the performance of PBPK models. We also investigated how the prediction accuracy of ADMET Predictor as well as its collaboration with SimCYP influence the quality of PBPK models constructed using SimCYP. In conclusion, we generated a practical guidance on applying two mainstream software packages, ADMET Predictor and SimCYP, to construct PBPK models for drugs or drug candidates which lack of ADME parameters in model construction.
更多查看译文
AI 理解论文
溯源树
样例
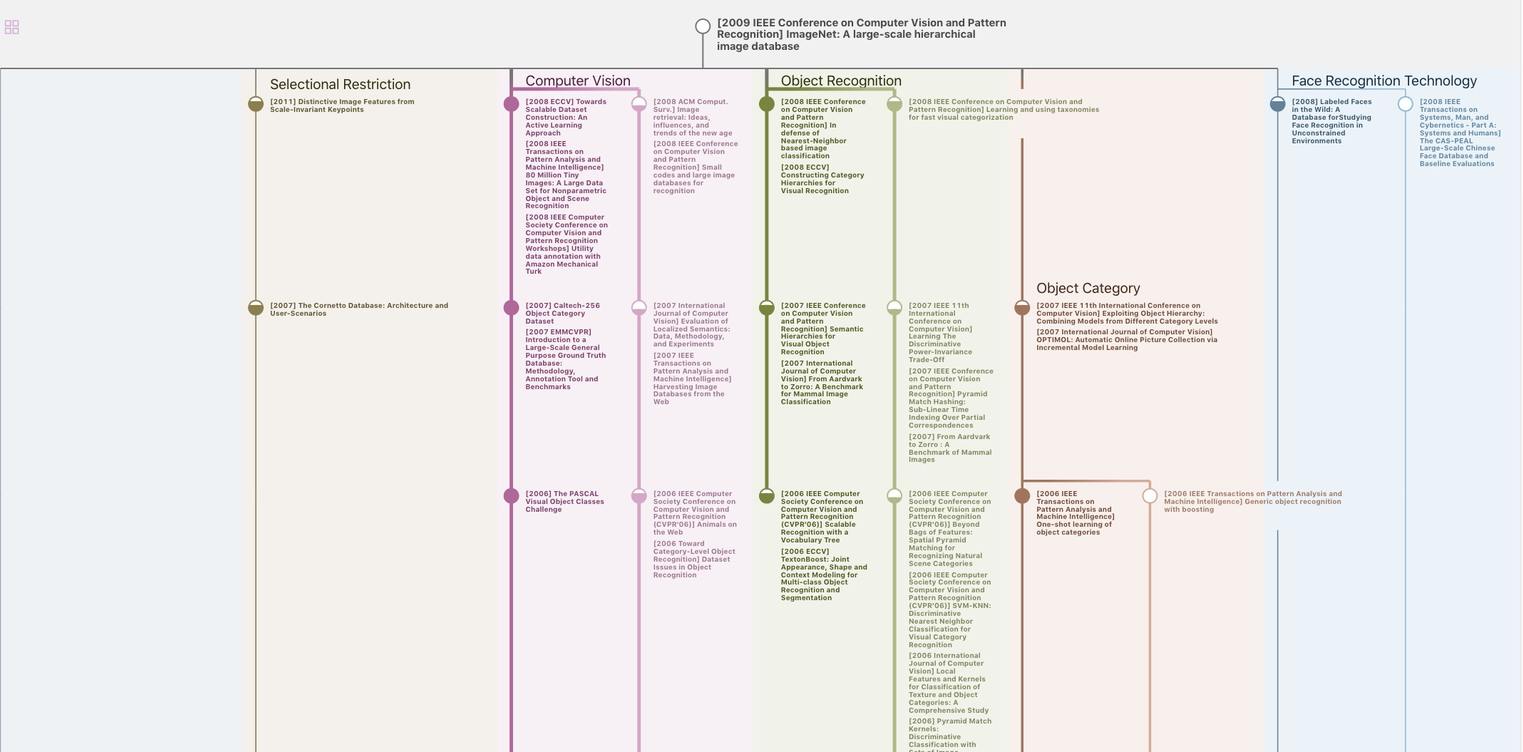
生成溯源树,研究论文发展脉络
Chat Paper
正在生成论文摘要