Semi-supervised multi-view concept decomposition
EXPERT SYSTEMS WITH APPLICATIONS(2024)
摘要
Concept Factorization (CF), as a novel paradigm of representation learning, has demonstrated superior perfor-mance in multi-view clustering tasks. It overcomes limitations such as the non-negativity constraint imposed by traditional matrix factorization methods and leverages kernel methods to learn latent representations that capture the underlying structure of the data, thereby improving data representation. However, existing multi-view concept factorization methods fail to consider the limited labeled information inherent in real-world multi-view data. This often leads to significant performance loss. To overcome these limitations, we propose a novel semi-supervised multi-view concept factorization model, named SMVCF. In the SMVCF model, we first extend the conventional single-view CF to a multi-view version, enabling more effective exploration of complementary information across multiple views. We then integrate multi-view CF, label propagation, and manifold learning into a unified framework to leverage and incorporate valuable information present in the data. Additionally, an adaptive weight vector is introduced to balance the importance of different views in the clustering process. We further develop targeted optimization methods specifically tailored for the SMVCF model. Finally, extensive experiments on four diverse datasets with varying label ratios demonstrated the effectiveness and superiority of the proposed SMVCF in multi-view clustering tasks.
更多查看译文
关键词
Concept decomposition,Multi-view clustering,Label propagation,Manifold learning
AI 理解论文
溯源树
样例
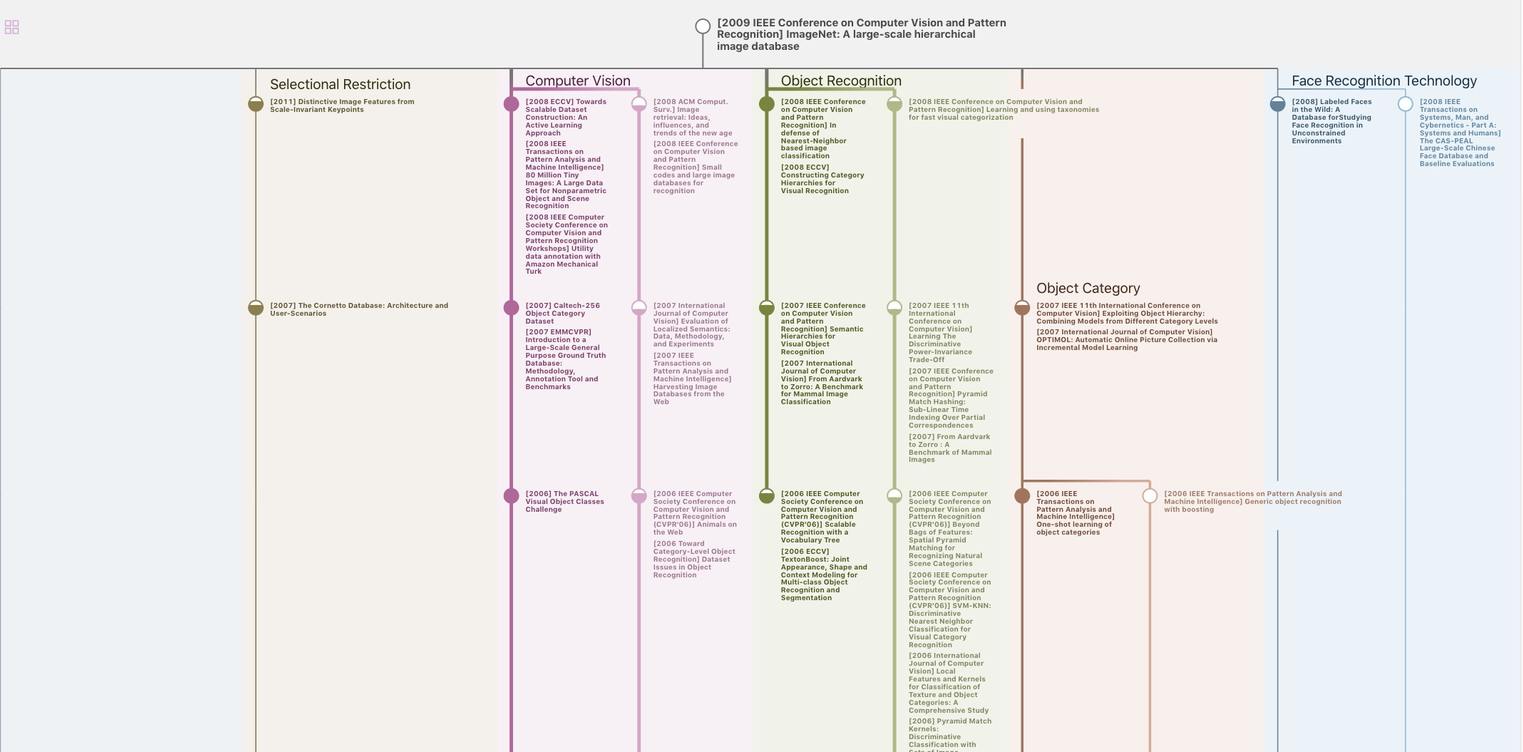
生成溯源树,研究论文发展脉络
Chat Paper
正在生成论文摘要