Quantum Machine Learning on Near-Term Quantum Devices: Current State of Supervised and Unsupervised Techniques for Real-World Applications
CoRR(2023)
摘要
The past decade has seen considerable progress in quantum hardware in terms of the speed, number of qubits and quantum volume which is defined as the maximum size of a quantum circuit that can be effectively implemented on a near-term quantum device. Consequently, there has also been a rise in the number of works based on the applications of Quantum Machine Learning (QML) on real hardware to attain quantum advantage over their classical counterparts. In this survey, our primary focus is on selected supervised and unsupervised learning applications implemented on quantum hardware, specifically targeting real-world scenarios. Our survey explores and highlights the current limitations of QML implementations on quantum hardware. We delve into various techniques to overcome these limitations, such as encoding techniques, ansatz structure, error mitigation, and gradient methods. Additionally, we assess the performance of these QML implementations in comparison to their classical counterparts. Finally, we conclude our survey with a discussion on the existing bottlenecks associated with applying QML on real quantum devices and propose potential solutions for overcoming these challenges in the future.
更多查看译文
关键词
machine learning,unsupervised techniques,supervised,near-term,real-world
AI 理解论文
溯源树
样例
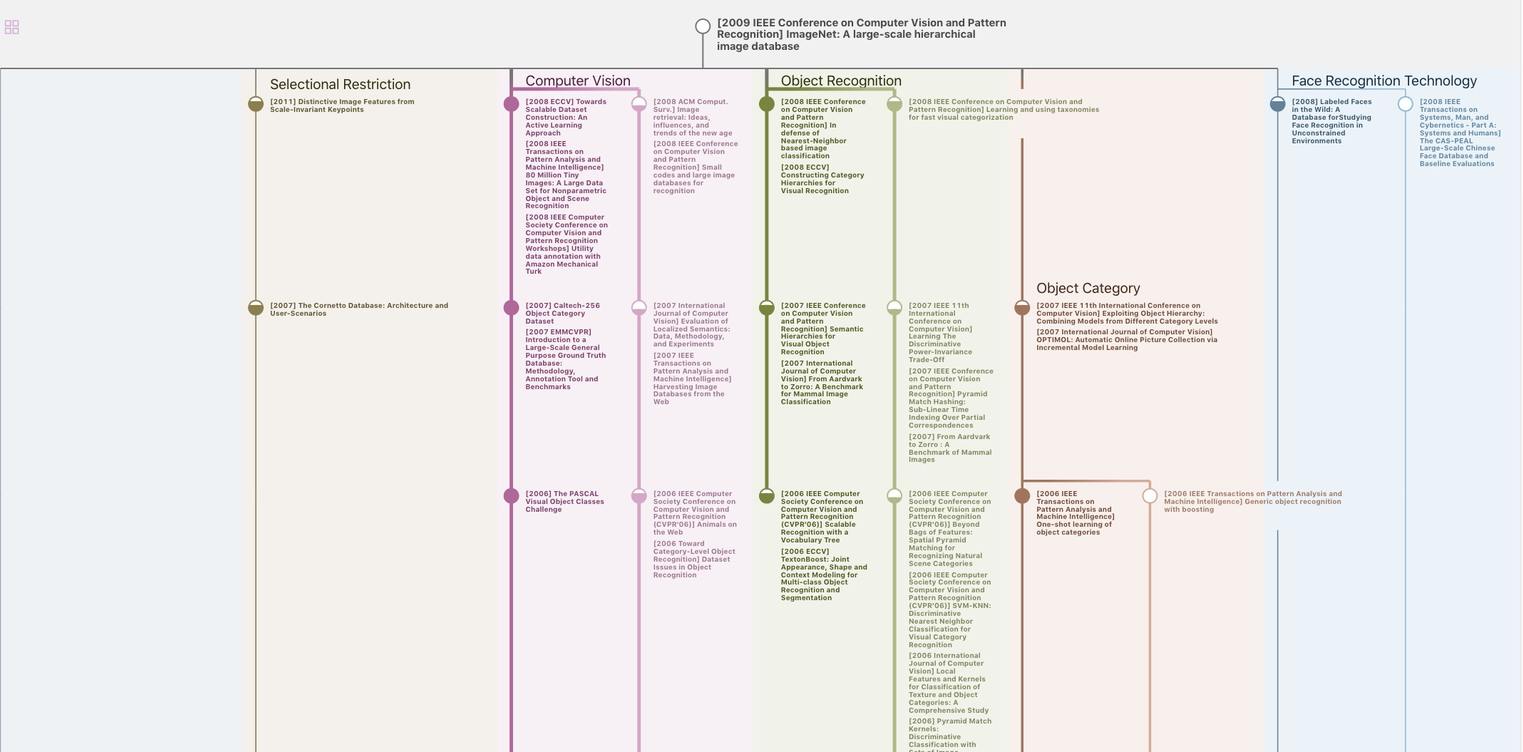
生成溯源树,研究论文发展脉络
Chat Paper
正在生成论文摘要