First Steps towards a Runtime Analysis of Neuroevolution
CoRR(2023)
摘要
We consider a simple setting in neuroevolution where an evolutionary algorithm optimizes the weights and activation functions of a simple artificial neural network. We then define simple example functions to be learned by the network and conduct rigorous runtime analyses for networks with a single neuron and for a more advanced structure with several neurons and two layers. Our results show that the proposed algorithm is generally efficient on two example problems designed for one neuron and efficient with at least constant probability on the example problem for a two-layer network. In particular, the so-called harmonic mutation operator choosing steps of size $j$ with probability proportional to $1/j$ turns out as a good choice for the underlying search space. However, for the case of one neuron, we also identify situations with hard-to-overcome local optima. Experimental investigations of our neuroevolutionary algorithm and a state-of-the-art CMA-ES support the theoretical findings.
更多查看译文
关键词
runtime analysis
AI 理解论文
溯源树
样例
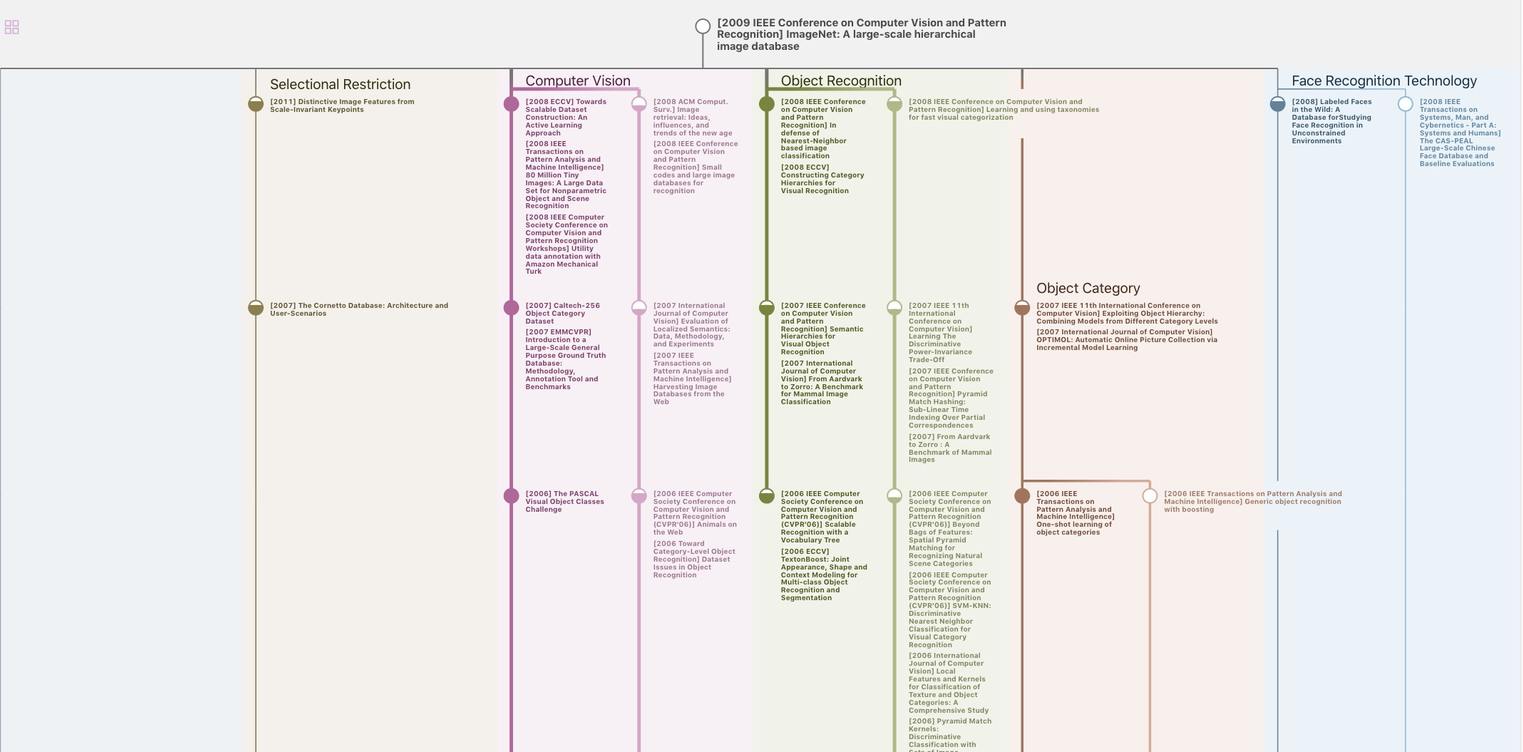
生成溯源树,研究论文发展脉络
Chat Paper
正在生成论文摘要