Data-driven simulation and characterization of gold nanoparticles melting
Research Square (Research Square)(2021)
摘要
Abstract We develop efficient, accurate, transferable, and interpretable machine learning force fields for Au nanoparticles, based on data gathered from Density Functional Theory calculations. We then use them to investigate the thermodynamic stability of Au nanoparticles of different sizes (1 to 6 nm), containing up to 6266 atoms, with respect to a solid-liquid phase change through molecular dynamics simulations. We predict nanoparticle melting temperatures in good agreement with respect to available experimental data. Furthermore, we characterize in detail the solid to liquid phase change mechanism employing an unsupervised learning scheme to categorize local atomic environments. We thus provide a rigorous and data-driven definition of liquid atomic arrangements in the inner and surface regions of a nanoparticle, and employ it to show that melting initiates at the outer layers.
更多查看译文
关键词
gold nanoparticles,melting,simulation,data-driven
AI 理解论文
溯源树
样例
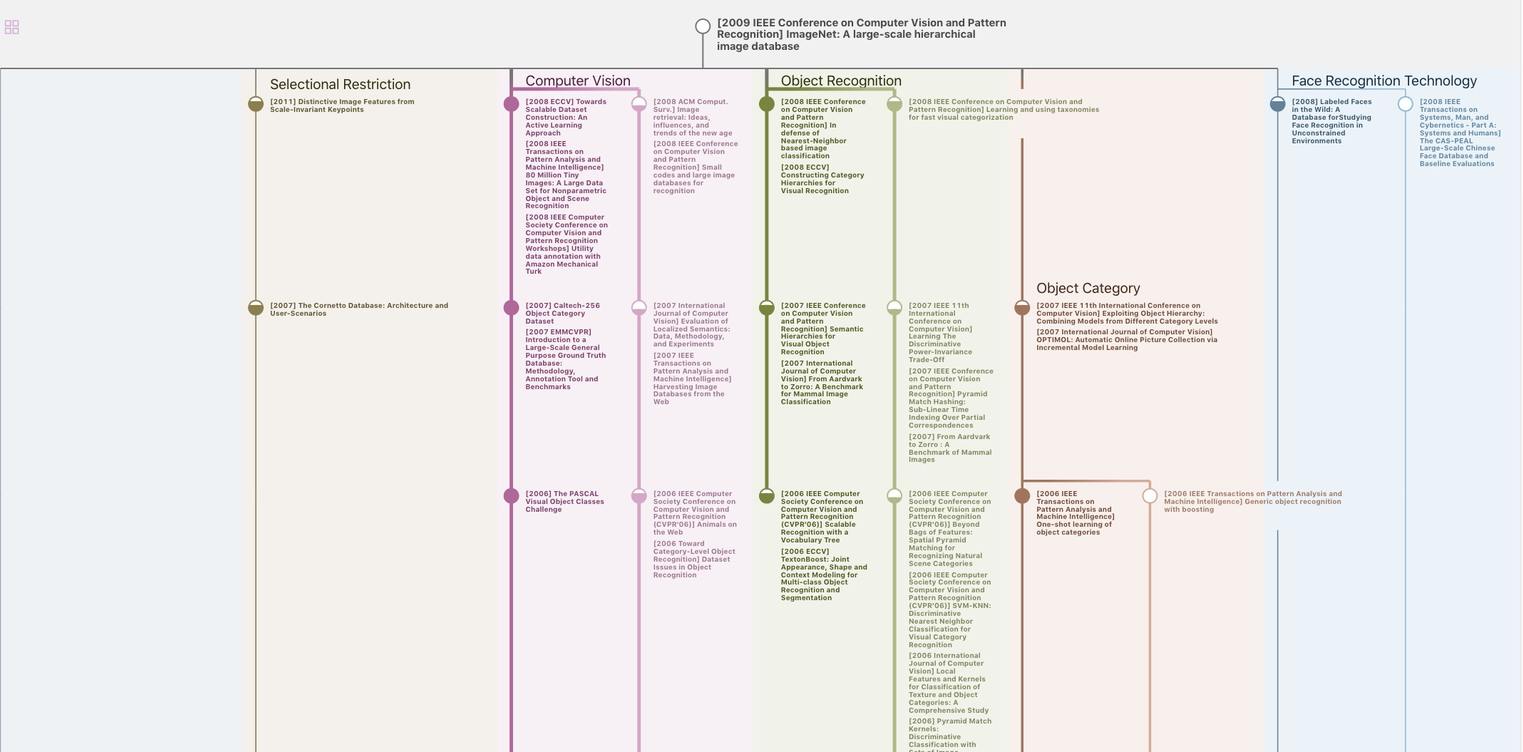
生成溯源树,研究论文发展脉络
Chat Paper
正在生成论文摘要