Area Contrast Distribution Loss for Underwater Image Enhancement
JOURNAL OF MARINE SCIENCE AND ENGINEERING(2023)
摘要
In this paper, we aim to design a lightweight underwater image enhancement algorithm that can effectively solve the problem of color distortion and low contrast in underwater images. Recently, enhancement methods typically optimize a perceptual loss function, using high-level features extracted from pre-trained networks to train a feed-forward network for image enhancement tasks. This loss function measures the perceptual and semantic differences between images, but it is applied globally across the entire image and does not consider semantic information within the image, which limits the effectiveness of the perceptual loss. Therefore, we propose an area contrast distribution loss (ACDL), which trains a flow model to achieve real-time optimization of the difference between output and reference in training. Additionally, we propose a novel lightweight neural network. Because underwater image acquisition is difficult, our experiments have shown that our model training can use only half the amount of data and half the image size compared to Shallow-UWnet. The RepNet network reduces the parameter size by at least 48% compared to previous algorithms, and the inference time is 5 times faster than before. After incorporating ACDL, SSIM increased by 2.70% and PSNR increased by 9.72%.
更多查看译文
关键词
underwater image enhancement,lightweight neural network,perceptual loss function,real time
AI 理解论文
溯源树
样例
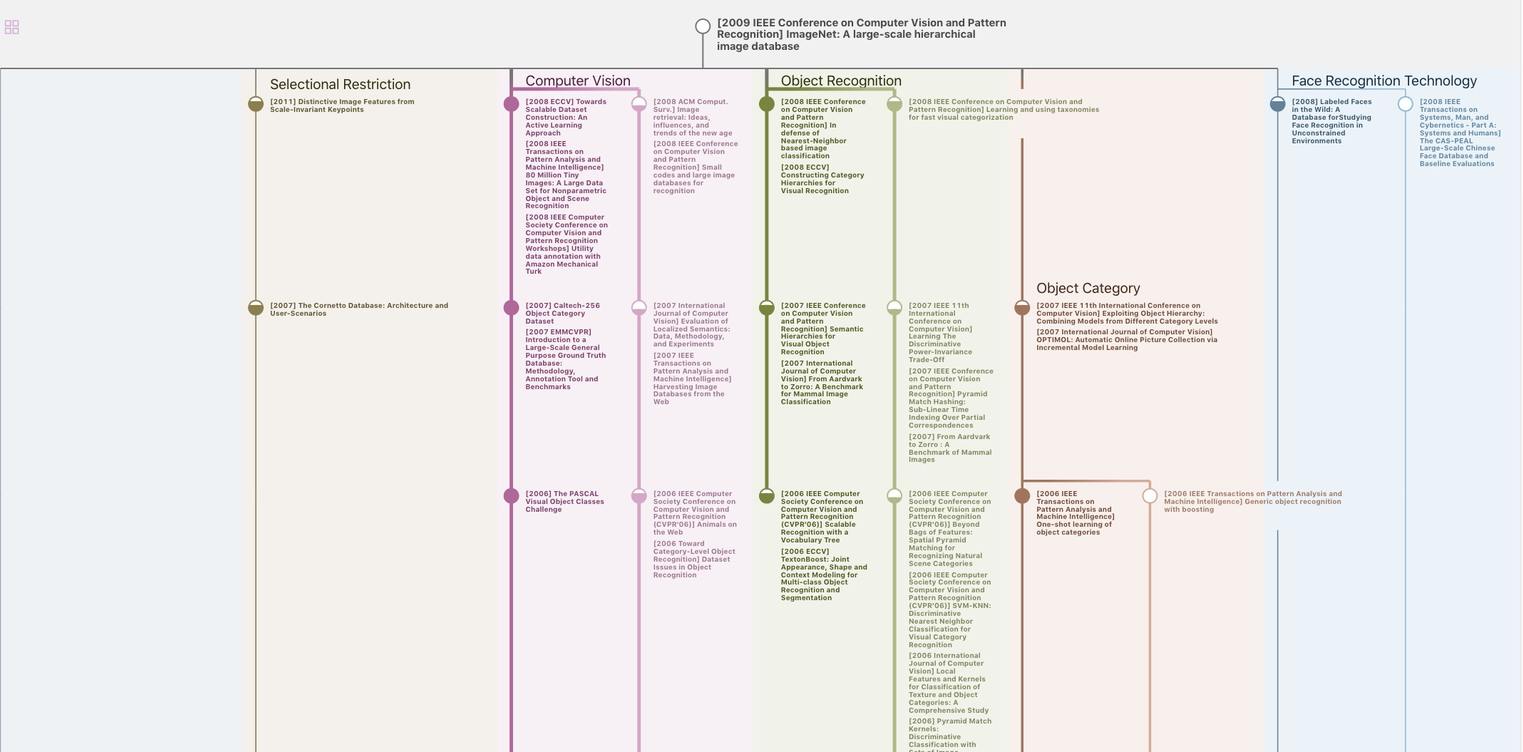
生成溯源树,研究论文发展脉络
Chat Paper
正在生成论文摘要