A Hybrid Atmospheric Model Incorporating Machine Learning Can Capture Dynamical Processes Not Captured by Its Physics-Based Component
GEOPHYSICAL RESEARCH LETTERS(2023)
摘要
It is shown that a recently developed hybrid modeling approach that combines machine learning (ML) with an atmospheric global circulation model (AGCM) can serve as a basis for capturing atmospheric processes not captured by the AGCM. This power of the approach is illustrated by three examples from a decades-long climate simulation experiment. The first example demonstrates that the hybrid model can produce sudden stratospheric warming, a dynamical process of nature not resolved by the low resolution AGCM component of the hybrid model. The second and third example show that introducing 6-hr cumulative precipitation and sea surface temperature (SST) as ML-based prognostic variables improves the precipitation climatology and leads to a realistic ENSO signal in the SST and atmospheric surface pressure.
更多查看译文
关键词
hybrid atmospheric model,machine learning,dynamical processes
AI 理解论文
溯源树
样例
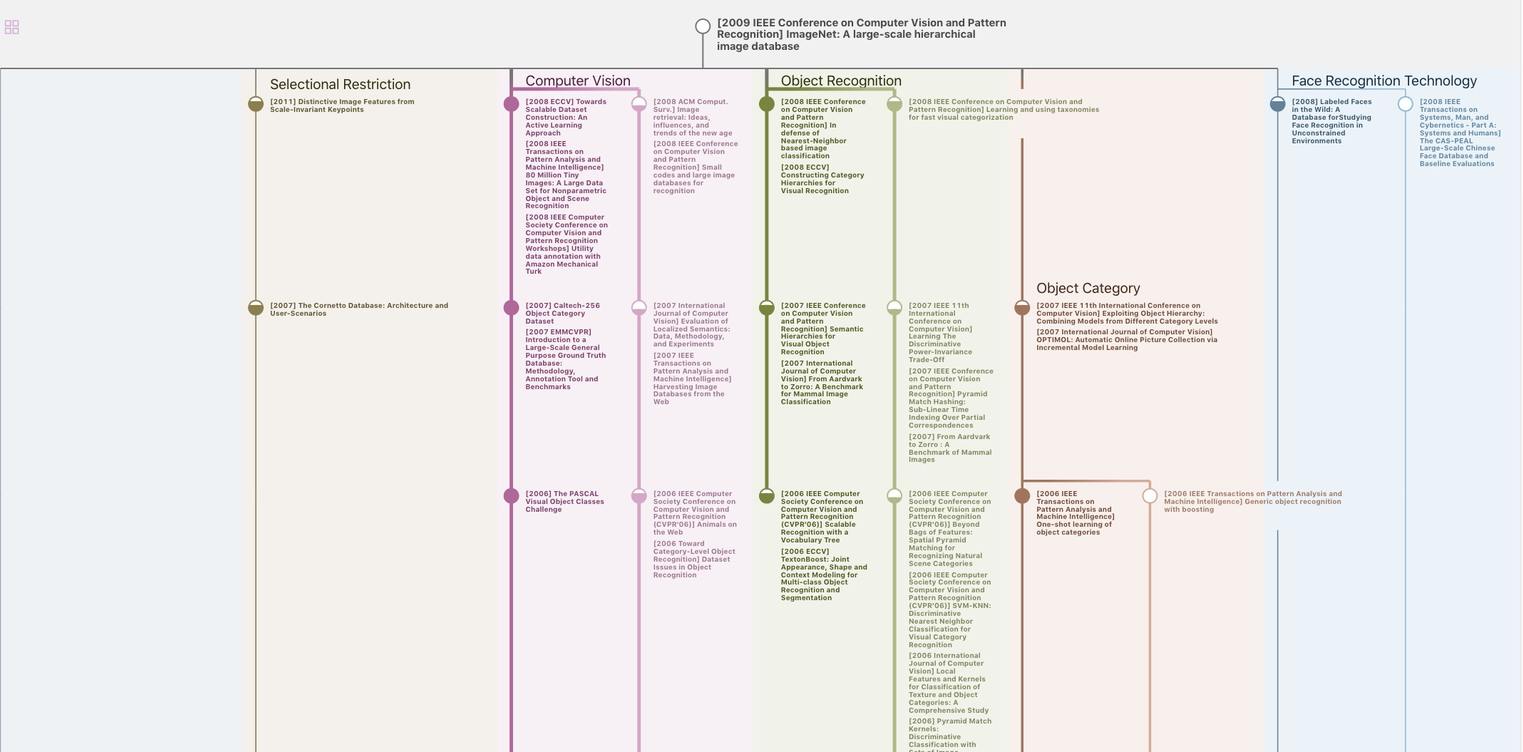
生成溯源树,研究论文发展脉络
Chat Paper
正在生成论文摘要