Exploring and Correcting the Bias in the Estimation of the Gini Measure of Inequality
SOCIOLOGICAL METHODS & RESEARCH(2023)
摘要
The Gini index is probably the most commonly used indicator to measure inequality. For continuous distributions, the Gini index can be computed using several equivalent formulations. However, this is not the case with discrete distributions, where controversy remains regarding the expression to be used to estimate the Gini index. We attempt to bring a better understanding of the underlying problem by regrouping and classifying the most common estimators of the Gini index proposed in both infinite and finite populations, and focusing on the biases. We use Monte Carlo simulation studies to analyse the bias of the various estimators under a wide range of scenarios. Extremely large biases are observed in heavy-tailed distributions with high Gini indices, and bias corrections are recommended in this situation. We propose the use of some (new and traditional) bootstrap-based and jackknife-based strategies to mitigate this bias problem. Results are based on continuous distributions often used in the modelling of income distributions. We describe a simulation-based criterion for deciding when to use bias corrections. Various real data sets are used to illustrate the practical application of the suggested bias corrected procedures.
更多查看译文
关键词
gini measure,inequality,estimation,bias
AI 理解论文
溯源树
样例
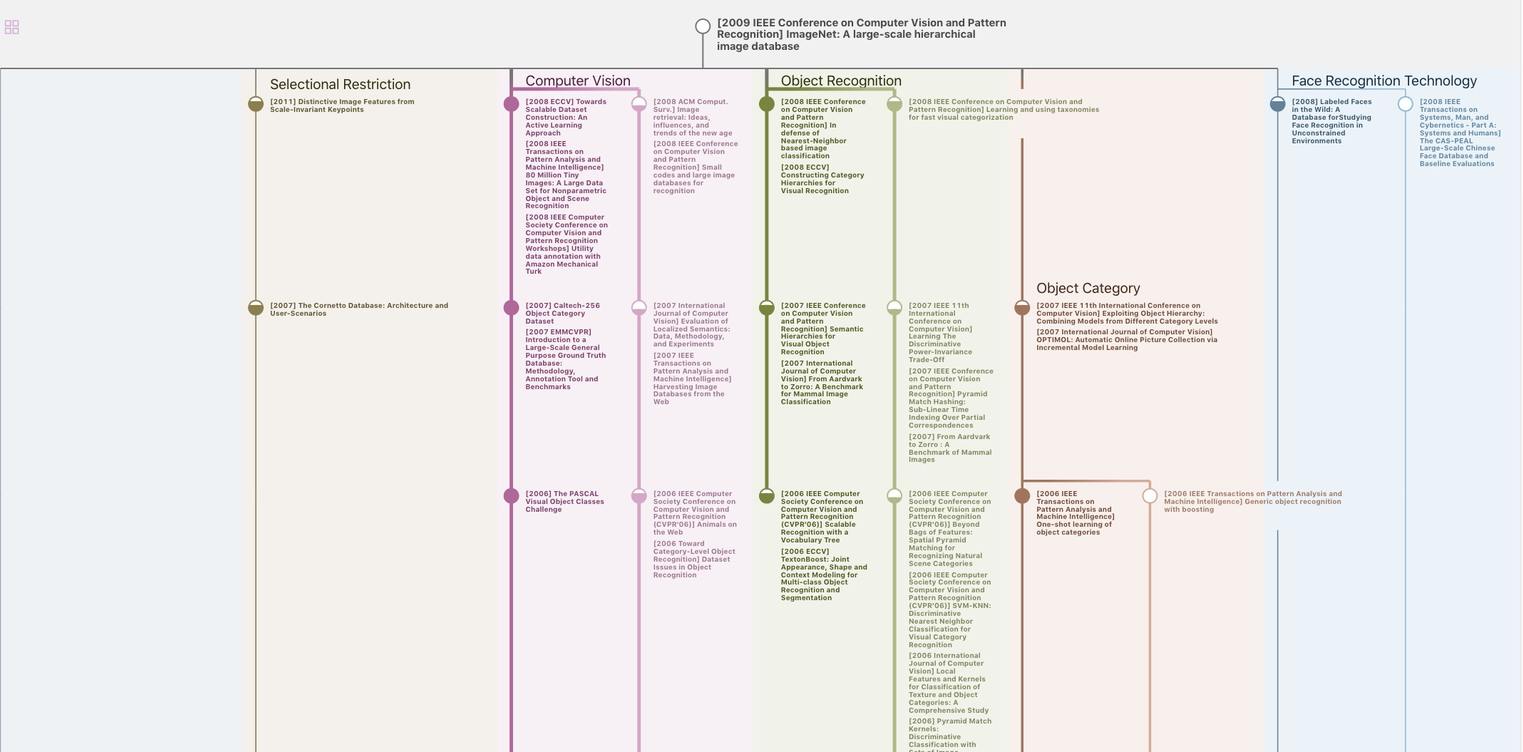
生成溯源树,研究论文发展脉络
Chat Paper
正在生成论文摘要