MFFNet: multimodal feature fusion network for point cloud semantic segmentation
VISUAL COMPUTER(2023)
摘要
We introduce a multimodal feature fusion network (MFFNet) for 3D point cloud semantic segmentation. Unlike previous methods that directly learn from colored point clouds (XYZRGB), MFFNet transforms point clouds to 2D RGB image and frequency image representations for efficient multimodal feature fusion. For each point, MFFNet performs a local projection by automatically learning a weighted orthogonal projection to softly project surrounding points onto 2D images. Regular 2D convolution can thus be applied to these regular grids for efficient semantic feature learning. Then, we fuse 2D semantic features into 3D point cloud features by using a multimodal feature fusion module (MFF). MFF module could employ high-level features from 2D RGB images and frequency images to boost the intrinsic correlation and discriminability of different structure features from the point cloud. In particular, the discriminative descriptions are quantified and leveraged as the local soft attention mask further to enforce the structure feature of the semantic categories. We have evaluated the proposed method on the S3DIS and ScanNet datasets. Experimental results and comparisons with four backbone methods demonstrate that our framework can perform better.
更多查看译文
关键词
Feature fusion,Point cloud semantic segmentation
AI 理解论文
溯源树
样例
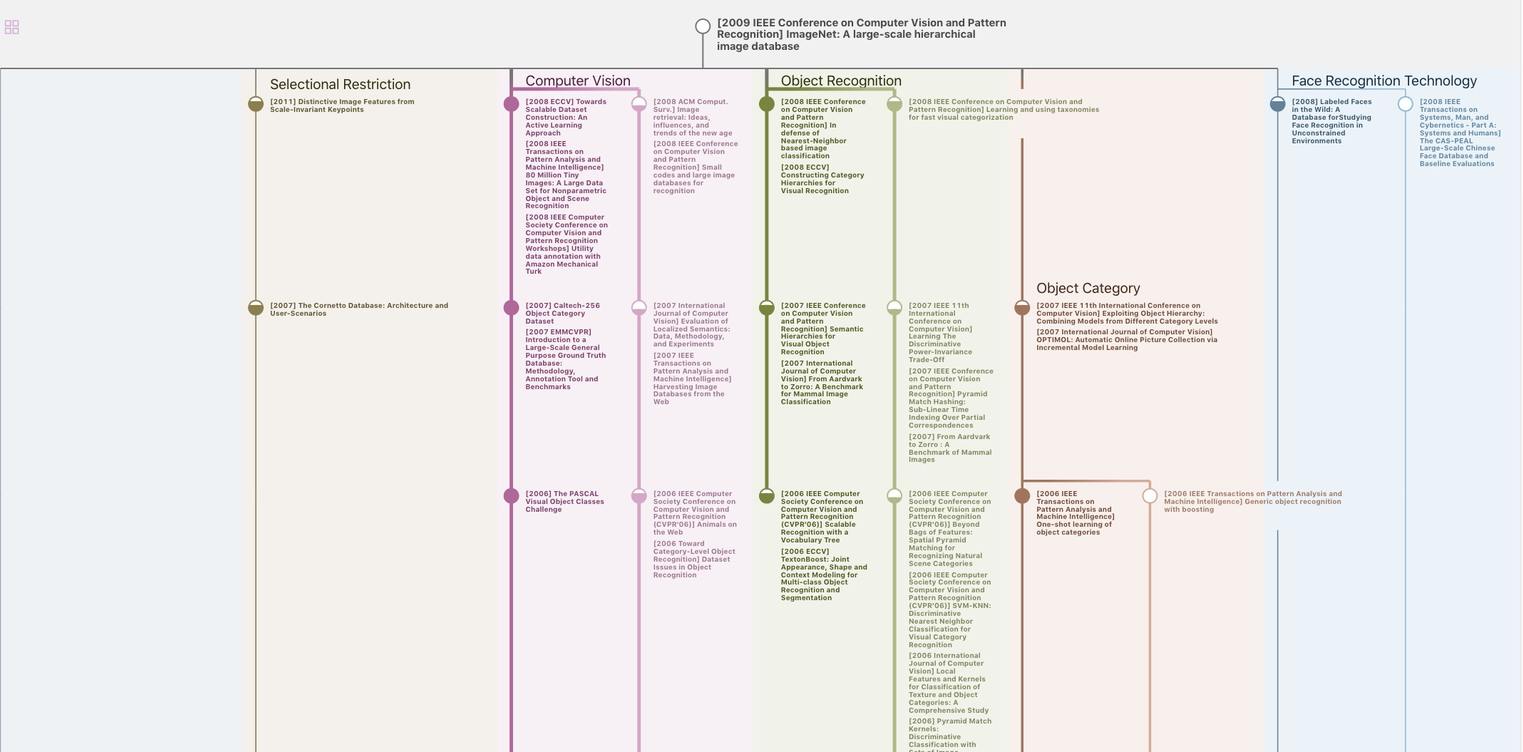
生成溯源树,研究论文发展脉络
Chat Paper
正在生成论文摘要