Matrix-Variate Hidden Markov Regression Models: Fixed and Random Covariates
JOURNAL OF CLASSIFICATION(2023)
摘要
Two families of matrix-variate hidden Markov regression models (MV-HMRMs) are here introduced. The distinction between them relies on the role of the covariates, which can be treated as fixed or random. Parsimony is achieved by using the eigen-decomposition of the components’ covariance matrices. This generates a different number of parsimonious models between the two families: 98 MV-HMRMs with fixed covariates and 9604 MV-HMRMs with random covariates. ECM algorithms are discussed for parameter estimation and, because of the high number of parsimonious models, convenient initialization, and fitting strategies are proposed. Our models are first applied to simulated data, and then to a four-way real dataset concerning the relationship between unemployment and labor force participation in the Italian provinces.
更多查看译文
关键词
Matrix-variate, Hidden Markov models, Parsimonious models
AI 理解论文
溯源树
样例
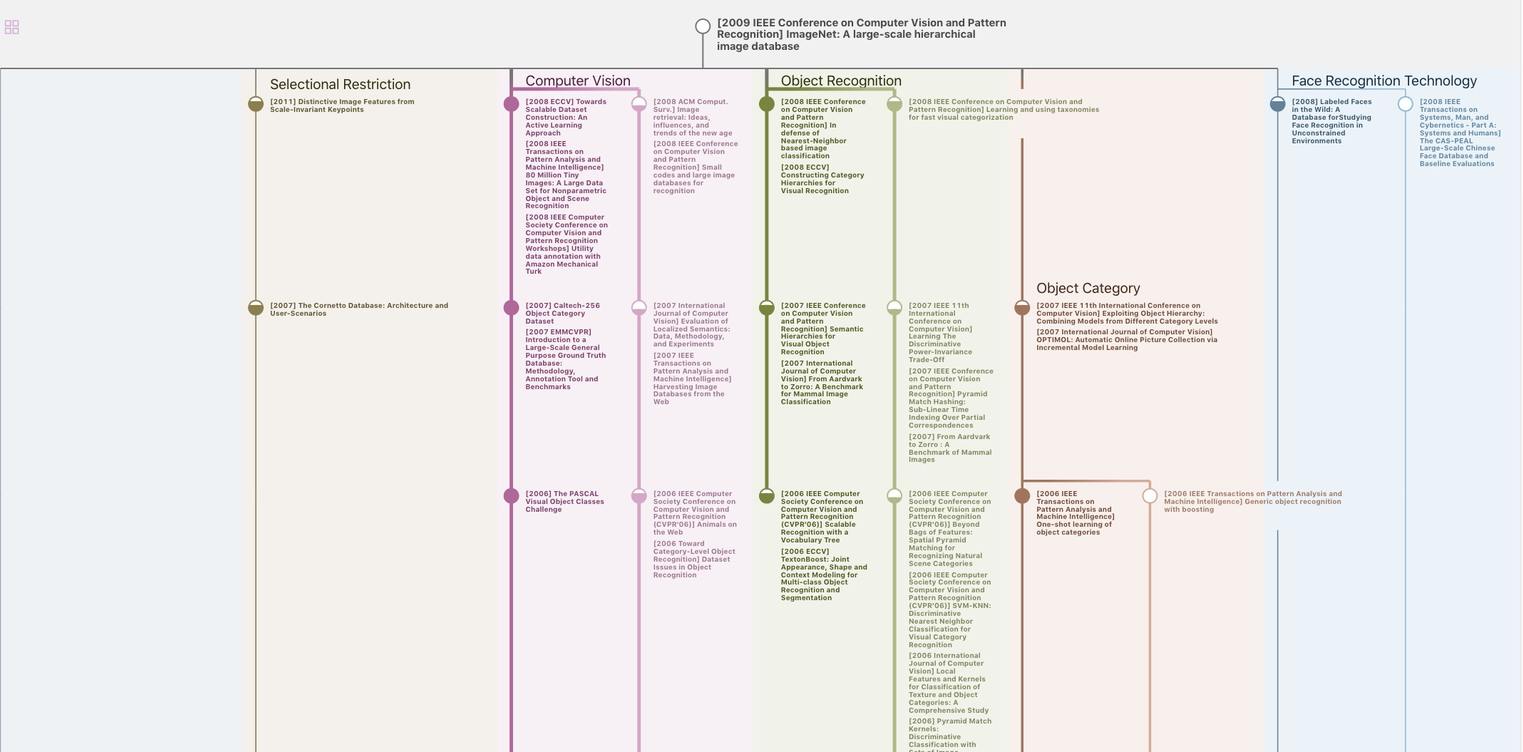
生成溯源树,研究论文发展脉络
Chat Paper
正在生成论文摘要