Development of generalizable automatic sleep staging using heart rate and movement based on large databases
BIOMEDICAL ENGINEERING LETTERS(2023)
摘要
Purpose With the advancement of deep neural networks in biosignals processing, the performance of automatic sleep staging algorithms has improved significantly. However, sleep staging using only non-electroencephalogram features has not been as successful, especially following the current American Association of Sleep Medicine (AASM) standards. This study presents a fine-tuning based approach to widely generalizable automatic sleep staging using heart rate and movement features trained and validated on large databases of polysomnography. Methods A deep neural network is used to predict sleep stages using heart rate and movement features. The model is optimized on a dataset of 8731 nights of polysomnography recordings labeled using the Rechtschaffen & Kales scoring system, and fine-tuned to a smaller dataset of 1641 AASM-labeled recordings. The model prior to and after fine-tuning is validated on two AASM-labeled external datasets totaling 1183 recordings. In order to measure the performance of the model, the output of the optimized model is compared to reference expert-labeled sleep stages using accuracy and Cohen’s κ as key metrics. Results The fine-tuned model showed accuracy of 76.6% with Cohen’s κ of 0.606 in one of the external validation datasets, outperforming a previously reported result, and showed accuracy of 81.0% with Cohen’s κ of 0.673 in another external validation dataset. Conclusion These results indicate that the proposed model is generalizable and effective in predicting sleep stages using features which can be extracted from non-contact sleep monitors. This holds valuable implications for future development of home sleep evaluation systems.
更多查看译文
关键词
generalizable automatic sleep staging,heart rate,databases
AI 理解论文
溯源树
样例
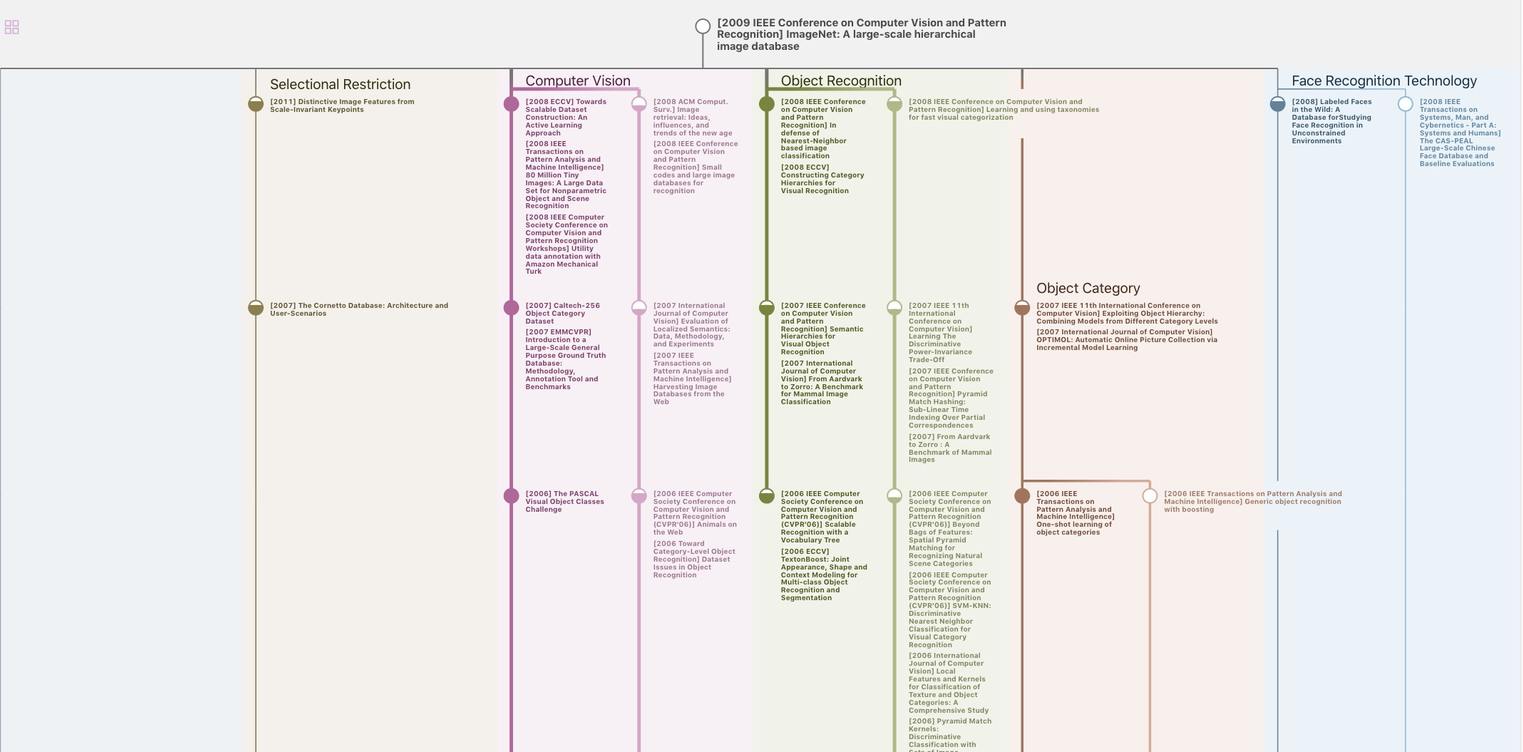
生成溯源树,研究论文发展脉络
Chat Paper
正在生成论文摘要