Classification of 1p/19q Status in Low-Grade Gliomas: Experiments with Radiomic Features and Ensemble- Based Machine Learning Methods
BRAZILIAN ARCHIVES OF BIOLOGY AND TECHNOLOGY(2023)
摘要
Gliomas comprise the vast majority of all malignant brain tumors. Low-grade glioma patients with combined whole-arm losses of 1p and 19q chromosomes were shown to have significantly better overall survival rates compared to non-deleted patients. This work evaluates several approaches for assessment of 1p/19q status from T2-weighted magnetic resonance images, using radiomics and machine learning. Experiments were performed using images from a public database (102 codeleted, 57 non-deleted). We experimented with sets of 68 and 100 radiomic features, and with several classifiers, including support vector machine, k-nearest neighbors, stochastic gradient descent, logistic regression, decision tree, Gaussian naive Bayes, and linear discriminant analysis. We also experimented with several ensemble-based methods, including four boosting-based classifiers, random forest, extra-trees, and bagging. The performance of these methods was compared using various metrics. Our best results were achieved using a bagging ensemble estimator based on the decision tree classifier, using only texture-based radiomics features. Compared to other works that used the same database, this approach provided higher sensitivity. It also achieved higher sensitivity than that provided by neurosurgeons and neuroradiologists analyzing the same images. We also show that including radiomic features associated with first order statistics and shape does not improve the performance of the classifiers, and in many cases worsens it. The molecular assessment of brain tumors through biopsies is an invasive procedure, and is subject to sampling errors. Therefore, the techniques presented in this work have strong potential for aiding in better clinical, surgical, and therapeutic decision -making.
更多查看译文
关键词
low-grade glioma, 1p, 19q codeletion, radiomics, machine learning, ensemble methods
AI 理解论文
溯源树
样例
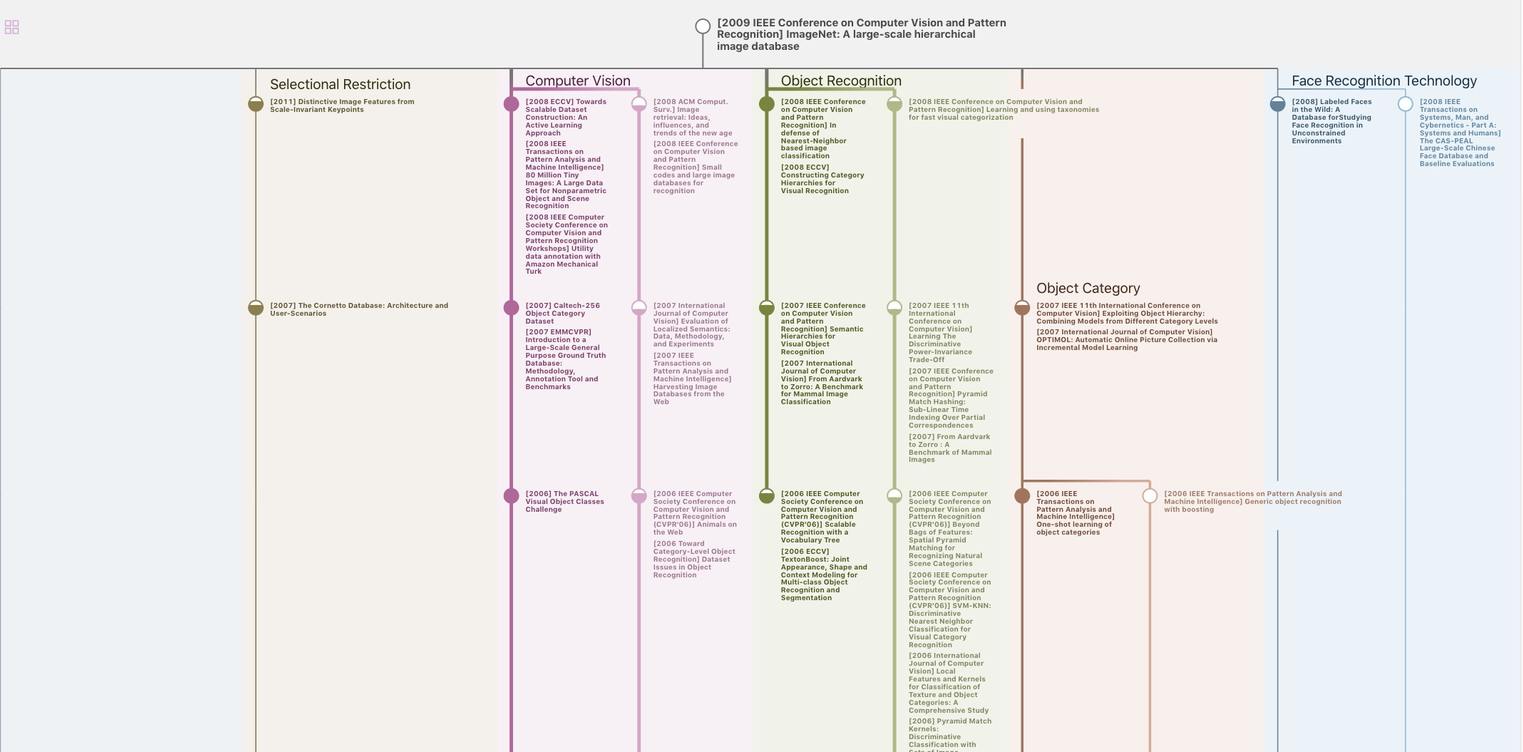
生成溯源树,研究论文发展脉络
Chat Paper
正在生成论文摘要