Genetic-Algorithm-Guided Development of Parametric Aeroelastic Reduced-Order Models with State-Consistence Enforcement
AIAA JOURNAL(2023)
摘要
Data-driven parametric reduced-order models (ROMs) in state-space form are valuable tools for rapid aeroelastic (AE) analysis and aerostructure control synthesis. However, the issue of state inconsistence (significant variations in model parameters over tradespaces) makes ROMs noninterpolatable, and therefore unable to accommodate use over broad flight parameter space. This paper presents a holistic framework that combines a system identification technique with state-consistence enforcement (SCE) and a genetic algorithm (GA) for the automated development of interpolatable AE ROMs across broad flight regimes. The SCE technique introduces a regularization term to the AutoRegressive model with eXogenous inputs (ARX) to specifically penalize model parameter variation between flight conditions. The GA autonomously guides the ROM development process toward optimal SCE-ARX hyperparameter selection that balances between model parameter variations and ROM accuracy. The GA-guided SCE-ARX approach is applied to build a parametric AE-ROM database at selected flight conditions, which, because of its state consistence, can be interpolated to create ROMs at any interstitial conditions, where training data or ROMs are not initially available, hence rapidly establishing the full coverage of the entire parameter space. The ROMs generated by the proposed method are compared with those by ARX, SCE, and GA-guided ARX in prediction accuracy. The individual and combined effects of SCE and GA on model parameter variation and ROM interpolatability are thoroughly investigated. The present method demonstrates the most accurate and robust performance for parametric ROM construction across the broad flight envelope.
更多查看译文
关键词
Genetic Algorithm,Reduced Order Modelling,State Space Representation,Nonlinear Aeroelastic Systems,Aircraft Wing Design,Autoregressive Model,Aircraft Components and Structure,Aerodynamic Force,Computational Fluid Dynamics,Multiple Input Multiple Output
AI 理解论文
溯源树
样例
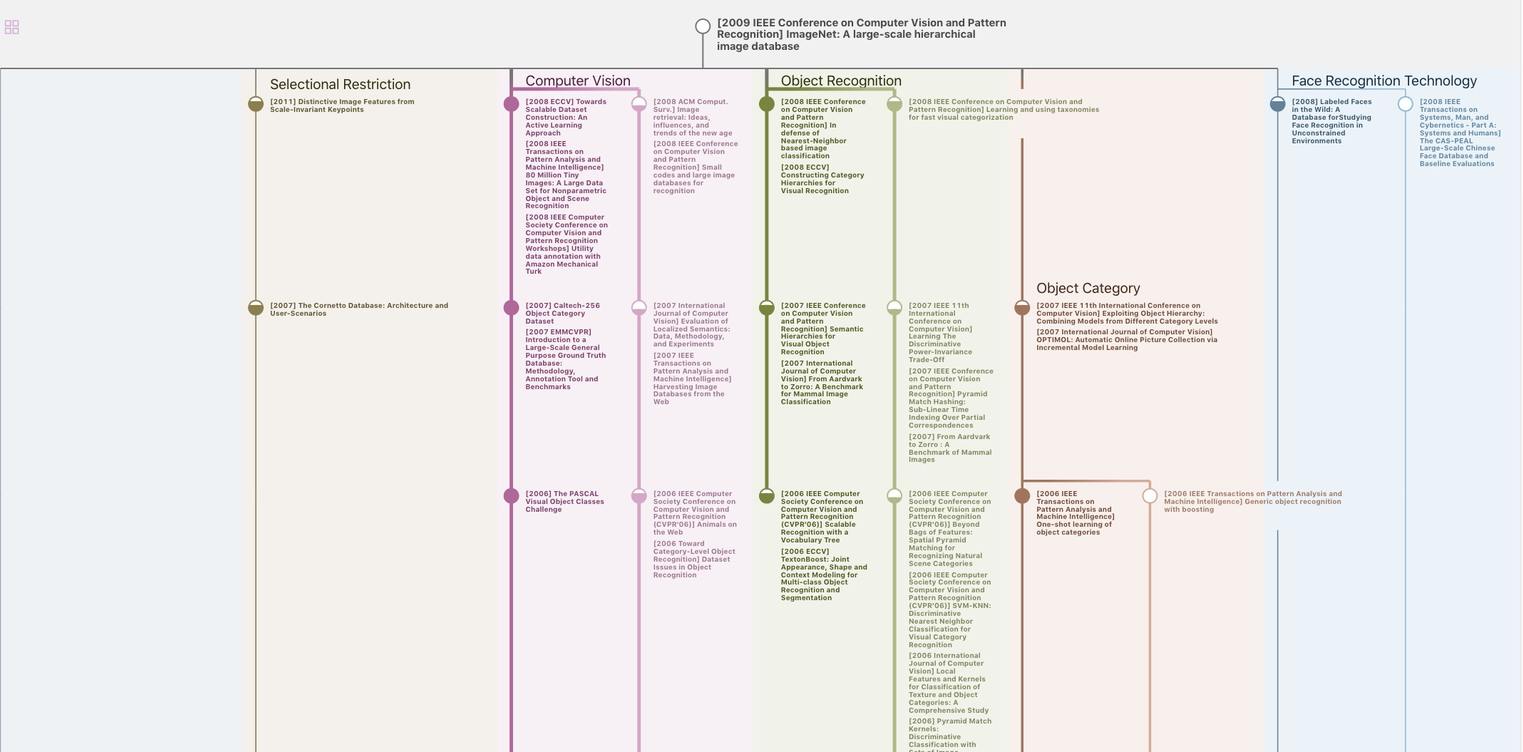
生成溯源树,研究论文发展脉络
Chat Paper
正在生成论文摘要