Knowledge-Transfer-Based Bidirectional Vessel Monitoring System for Remote and Nearshore Images
JOURNAL OF MARINE SCIENCE AND ENGINEERING(2023)
摘要
Vessel monitoring technology involves the application of remote sensing technologies to detect and identify vessels in various environments, which is critical for monitoring vessel traffic, identifying potential threats, and facilitating maritime safety and security to achieve real-time maritime awareness in military and civilian domains. However, most existing vessel monitoring models tend to focus on a single remote sensing information source, leading to limited detection functionality and underutilization of available information. In light of these limitations, this paper proposes a comprehensive ship monitoring system that integrates remote satellite devices and nearshore detection equipment. The system employs ResNet, a deep learning model, along with data augmentation and transfer learning techniques to enable bidirectional detection of satellite cloud images and nearshore outboard profile images, thereby alleviating prevailing issues such as low detection accuracy, homogeneous functionality, and poor image recognition applicability. Empirical findings based on two real-world vessel monitoring datasets demonstrate that the proposed system consistently performs best in both nearshore identification and remote detection. Additionally, extensive supplementary experiments were conducted to evaluate the effectiveness of different modules and discuss the constraints of current deep learning-based vessel monitoring models.
更多查看译文
关键词
ship classification, synthetic-aperture radar (SAR), deep learning, transfer learning, vessel monitoring system
AI 理解论文
溯源树
样例
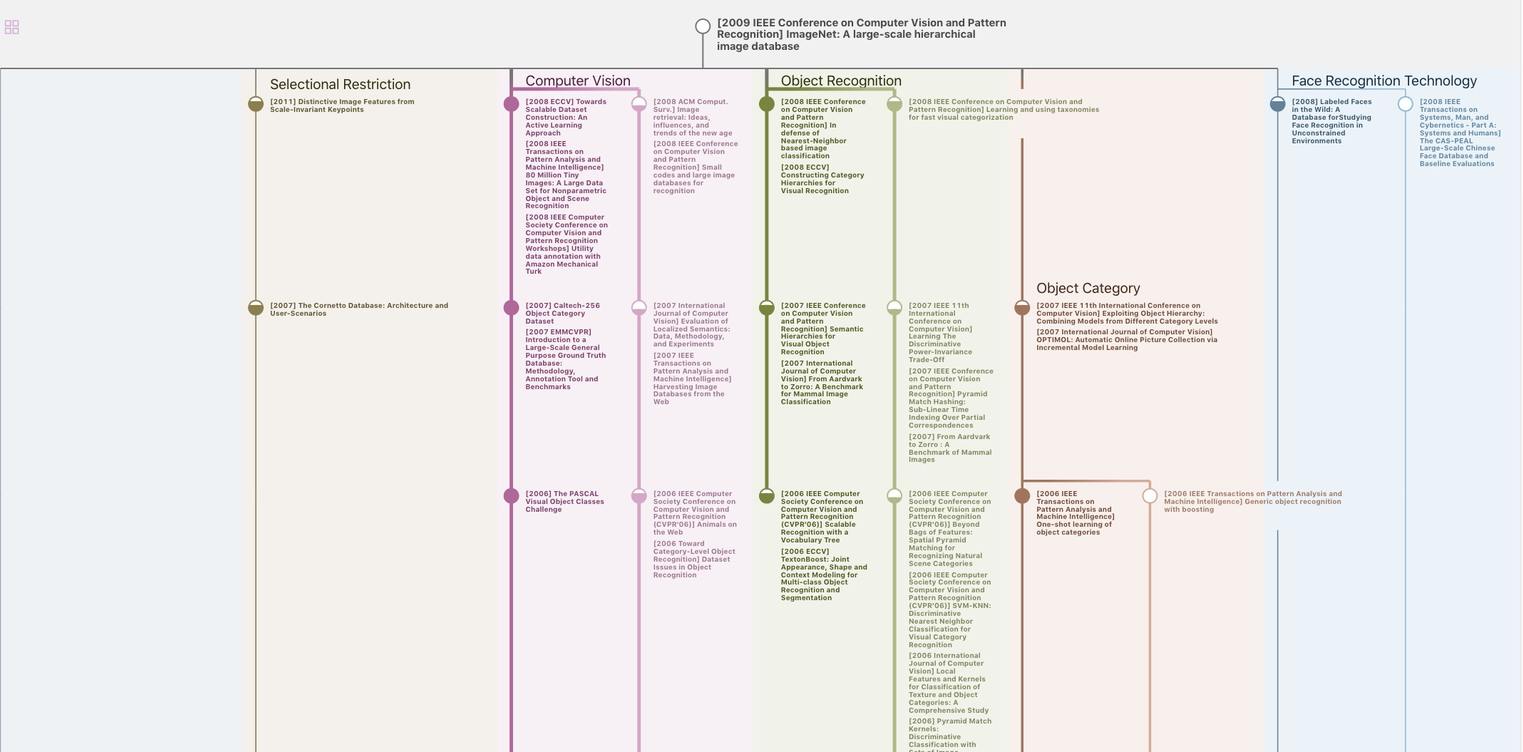
生成溯源树,研究论文发展脉络
Chat Paper
正在生成论文摘要