Parameter Tuned Deep Learning Based Traffic Critical Prediction Model on Remote Sensing Imaging
CMC-COMPUTERS MATERIALS & CONTINUA(2023)
摘要
Remote sensing (RS) presents laser scanning measurements, aerial photos, and high-resolution satellite images, which are utilized for extracting a range of traffic-related and road-related features. RS has a weakness, such as traffic fluctuations on small time scales that could distort the accuracy of predicted road and traffic features. This article introduces an Optimal Deep Learning for Traffic Critical Prediction Model on High-Resolution Remote Sensing Images (ODLTCP-HRRSI) to resolve these issues. The presented ODLTCP-HRRSI technique majorly aims to forecast the critical traffic in smart cities. To attain this, the presented ODLTCP-HRRSI model performs two major processes. At the initial stage, the ODLTCP-HRRSI technique employs a convolutional neural network with an auto-encoder (CNN-AE) model for productive and accurate traffic flow. Next, the hyperparameter adjustment of the CNN-AE model is performed via the Bayesian adaptive direct search optimization (BADSO) algorithm. The experimental outcomes demonstrate the enhanced performance of the ODLTCP-HRRSI technique over recent approaches with maximum accuracy of 98.23%.
更多查看译文
关键词
deep learning,remote sensing imaging,remote sensing,prediction
AI 理解论文
溯源树
样例
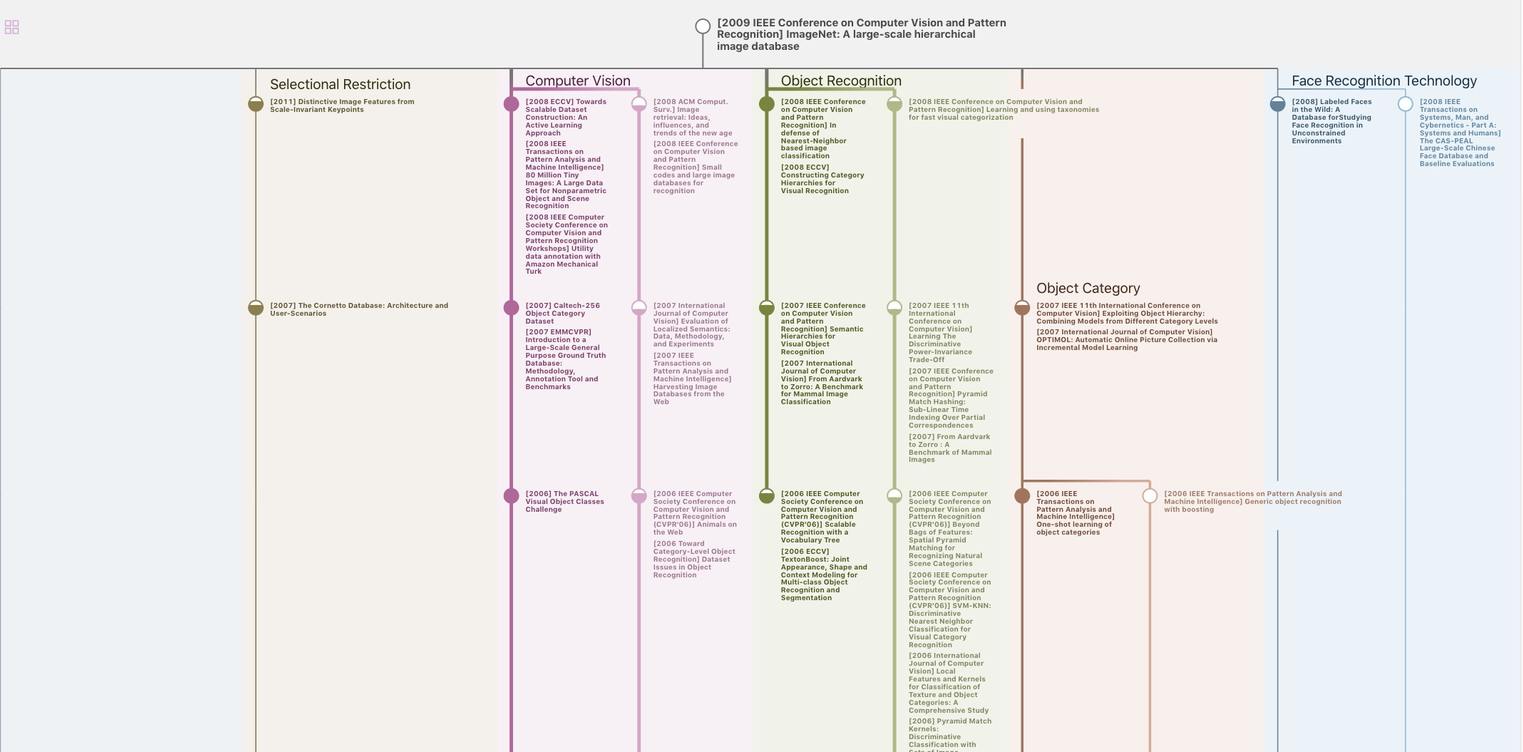
生成溯源树,研究论文发展脉络
Chat Paper
正在生成论文摘要