Fair machine learning through constrained stochastic optimization and an e-constraint method
OPTIMIZATION LETTERS(2023)
摘要
A strategy for fair supervised learning is proposed. It involves formulating an optimization problem to minimize loss subject to a prescribed bound on a measure of unfairness (e.g., disparate impact). It can be embedded within an e-constraint method for multiobjective optimization, allowing one to produce a Pareto front for minimizing loss and unfairness. A stochastic optimization algorithm, designed to be scalable for large data settings, is proposed for solving the arising constrained optimization problems. Numerical experiments on problems pertaining to predicting recidivism and income provide evidence that the strategy can be effective for largescale fair learning.
更多查看译文
关键词
Stochastic multiobjective optimization, Fair machine learning
AI 理解论文
溯源树
样例
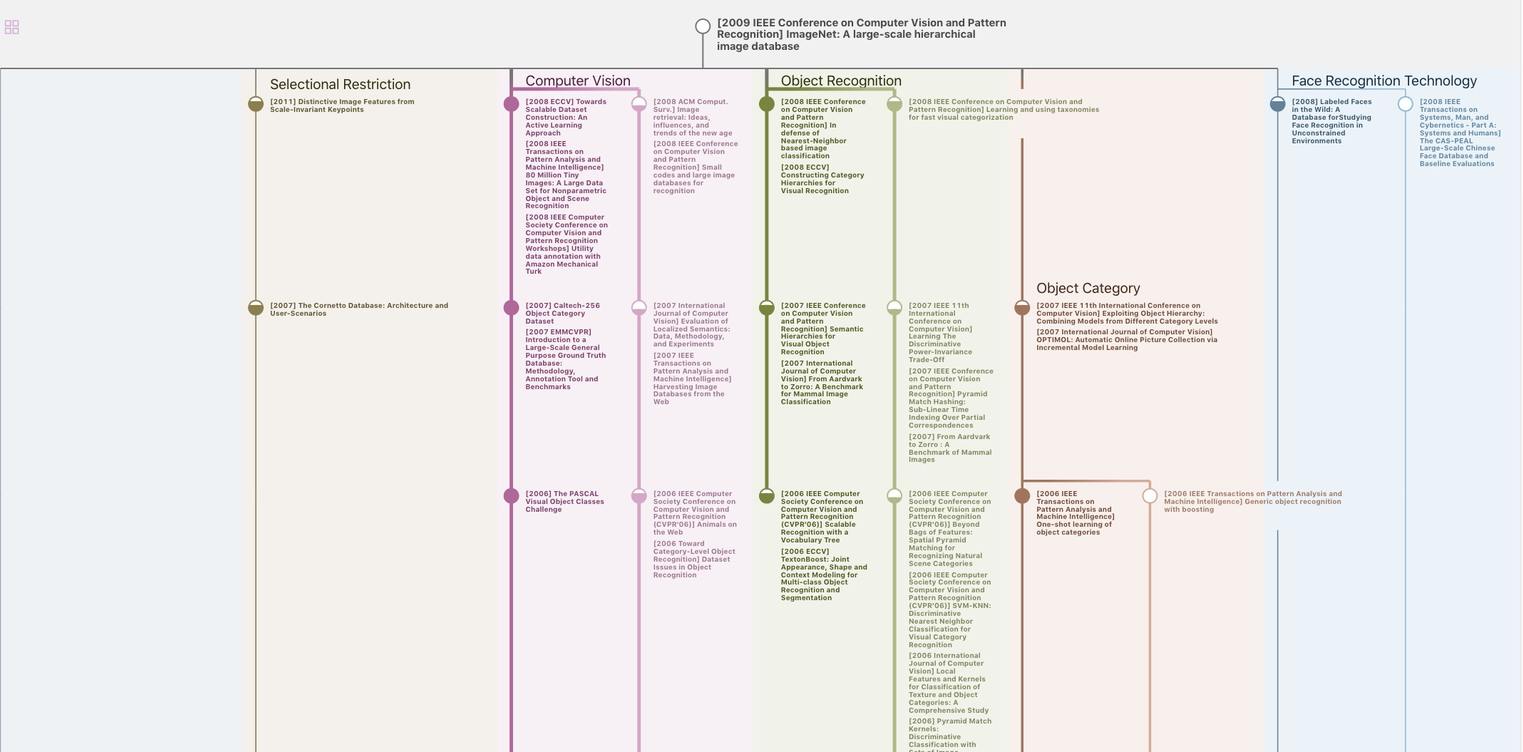
生成溯源树,研究论文发展脉络
Chat Paper
正在生成论文摘要