Multi-objective Game Learning Algorithm Based on Multi-armed Bandit in Underwater Acoustic Communication Networks
SENSORS AND MATERIALS(2023)
摘要
To address the challenges of interference in underwater multi-node communication and enhance the efficiency of underwater acoustic communication, we propose a multi-objective game learning algorithm based on the multi-armed bandit framework. Firstly, the multi-objective optimization problem is constructed as a multi-node multi-armed bandit (MAB) game model. Secondly, we incorporate the overall network interference level and nodes' power cost in the utility function to achieve the desired optimization objectives. Thirdly, we establish the existence and uniqueness of the Nash equilibrium point of the game model and introduce an improved greedy strategy MAB learning algorithm to determine the equilibrium solution. Finally, our simulation results demonstrate that the proposed algorithm effectively optimizes interference management while enhancing the nodes' adaptive capabilities.
更多查看译文
关键词
underwater acoustic communication, reinforcement learning, power allocation, multi-armed bandit
AI 理解论文
溯源树
样例
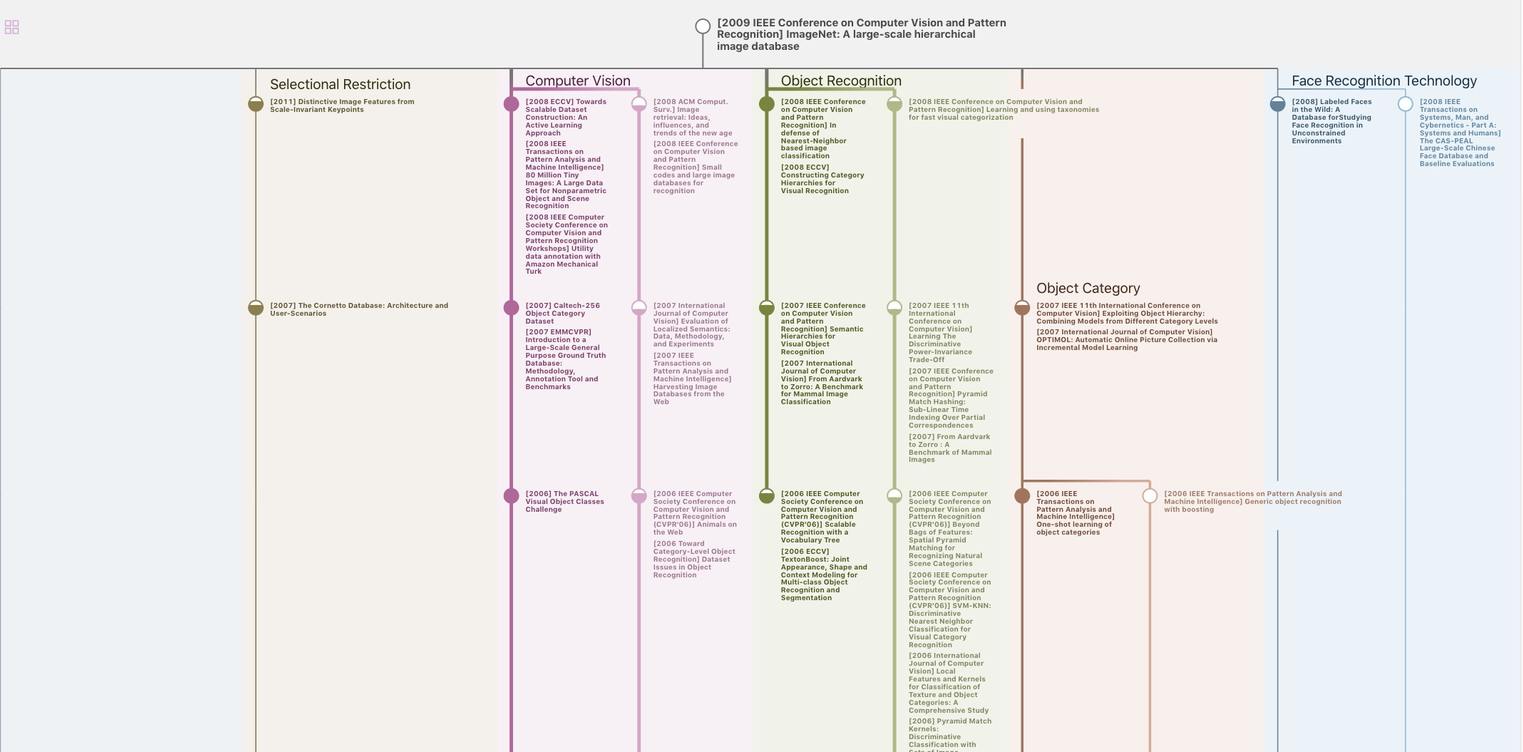
生成溯源树,研究论文发展脉络
Chat Paper
正在生成论文摘要