Teaching quality evaluation-based differential evolution and its application on synthesis of linear sparse arrays
SOFT COMPUTING(2023)
摘要
To enhance the solution quality and accelerate the convergence speed, teaching quality evaluation-based differential evolution algorithm with Levy flight and adaptive parameters is proposed in this paper, which contains two modifications. One is the new multi-population strategy inspired by teaching quality evaluation. In this multi-population strategy, first, the entire evolving population is partitioned into several subpopulations of equal size and each subpopulation is evaluated and assigned an evaluation score like the way in teaching quality evaluation, according to which new individuals are generated. Then the subpopulations integrate and are re-divided to make some individuals in the better subpopulations spread to other subpopulations, thus guiding the evolution of the entire population and accelerating the convergence speed. The other modification is the improved mutation strategy combined with Levy flight and adaptive parameters. Levy flight can avoid the algorithm stuck with the local optimum, and adaptive parameters can change dynamically during the evolutionary process, hence reducing the adverse effects caused by parameter sensitivity. To validate the effectiveness of the proposed algorithm, extensive test experiments on benchmark functions are carried out, and the two modifications are studied separately at length. At the end, the proposed algorithm is applied to synthesize linear sparse array antennas. The results show that it can reduce peak sidelobe level further and enhance the performance of linear sparse arrays.
更多查看译文
关键词
Differential evolution,Teaching quality evaluation,Levy flight,Adaptive parameters,Antenna arrays
AI 理解论文
溯源树
样例
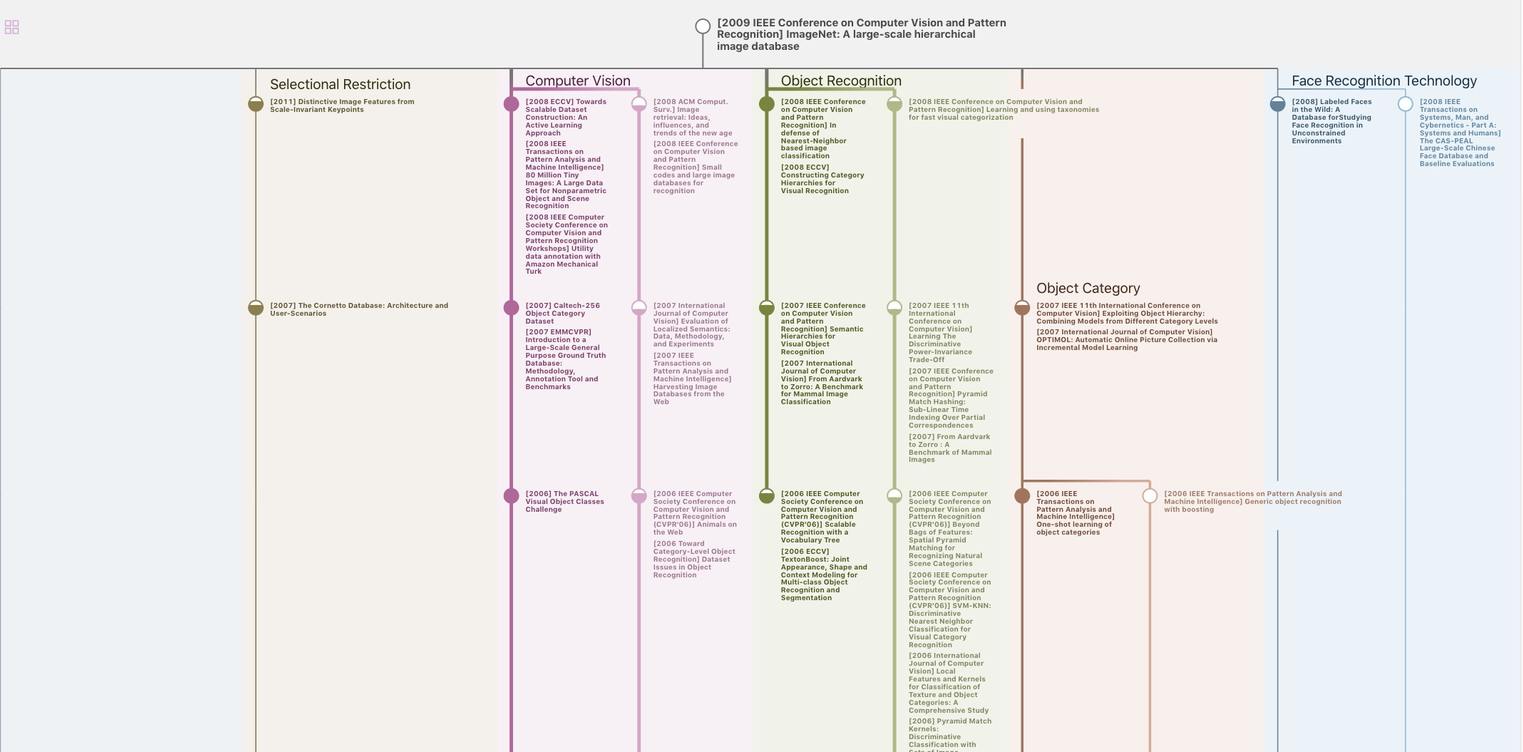
生成溯源树,研究论文发展脉络
Chat Paper
正在生成论文摘要