Multiple information perception-based attention in YOLO for underwater object detection
VISUAL COMPUTER(2024)
摘要
Underwater object detection is a prerequisite for underwater robots to achieve autonomous operation and ocean exploration. However, poor imaging quality, harsh underwater environments, and concealed underwater targets greatly aggravate the difficulty of underwater object detection. In order to reduce underwater background interference and improve underwater object perception, we propose a multiple information perception-based attention module (MIPAM), which is mainly composed of five processes. In information preprocessing, spatial downsampling and channel splitting control parameters and computations of attention module by reducing dimension sizes. In information collection, channel-level information collection and spatial-level information collection enhance the semantic information expression by perceiving multi-dimensional dependency information, multi-dimensional structure information and multi-dimensional global information. In information interaction, channel-driven information interaction and spatial-driven information interaction stimulate the intrinsic interaction potential by further perceiving multi-dimensional diversity information. Adaptive feature fusion further improves the information interaction quality by allocating learnable parameters. In attention activation, the multi-branch structure enhances the attention calibration efficiency by generating multiple attention. In information postprocessing, channel concatenation and spatial upsampling realize the plug-and-play of attention module by restoring original feature states. In order to meet the high-precision and real-time requirements for underwater object detection, we integrate MIPAM into YOLO detectors. The experimental results indicate that our work brings significant performance gains for underwater detection tasks. Our work also provides some performance improvements for other detection tasks, which shows the ideal generalization ability.
更多查看译文
关键词
Underwater object detection,Information perception,Attention mechanism,YOLO detector
AI 理解论文
溯源树
样例
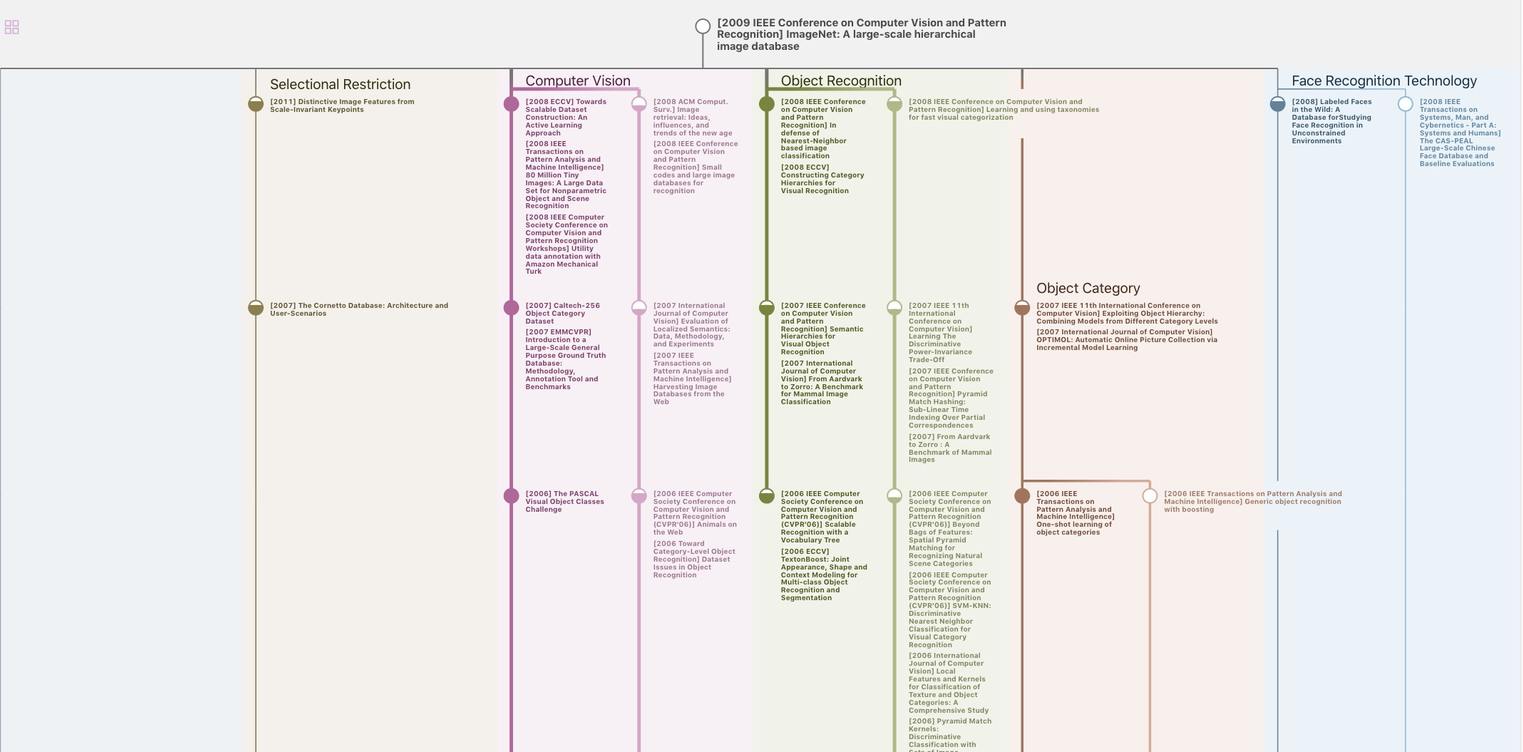
生成溯源树,研究论文发展脉络
Chat Paper
正在生成论文摘要