Profiling and Pairing Catchments and Hydrological Models With Latent Factor Model
WATER RESOURCES RESEARCH(2023)
摘要
Hydrological models are simplified representations of catchments. Finding an appropriate model instance (i.e., a combination of structure and parameter values) for a given catchment is a fundamental problem in hydrological modeling. This study develops a latent factor-based machine learning method to learn to predict the pairwise association between the behavioral characteristics under climate forcing of a catchment and a hydrological model instance. The underlying assumption is that the behaviors of catchments and model instances can be sufficiently well characterized by sets of latent factors that allow accurate prediction of the associations between observed and modeled behaviors. In this study, the proposed method was applied to learn the latent factor vectors (which are numerical values) of 2,649 catchments worldwide and over 1,000,000 model instances from their pairwise associations (i.e., similarities between observed and simulated catchment behaviors). The model instances were created using 36 conceptual model structures or modeling software, and the their parameter values were randomly sampled. The results show that the learned latent factor vectors are able to accurately characterize the associations between catchments and hydrological model instances. The latent factor vector of a "new" catchment (not seen by the training procedures) can be estimated by running a small number of randomly sampled model instances, from which well-fitting model instances created using different conceptual models can be recommended. The recommended instances can be of comparable quality to those derived using a calibration algorithm, and the method is useful for catchments outside the regions from which the latent factor vectors of the model instances were derived.
更多查看译文
关键词
pairing catchments,hydrological models,profiling,factor
AI 理解论文
溯源树
样例
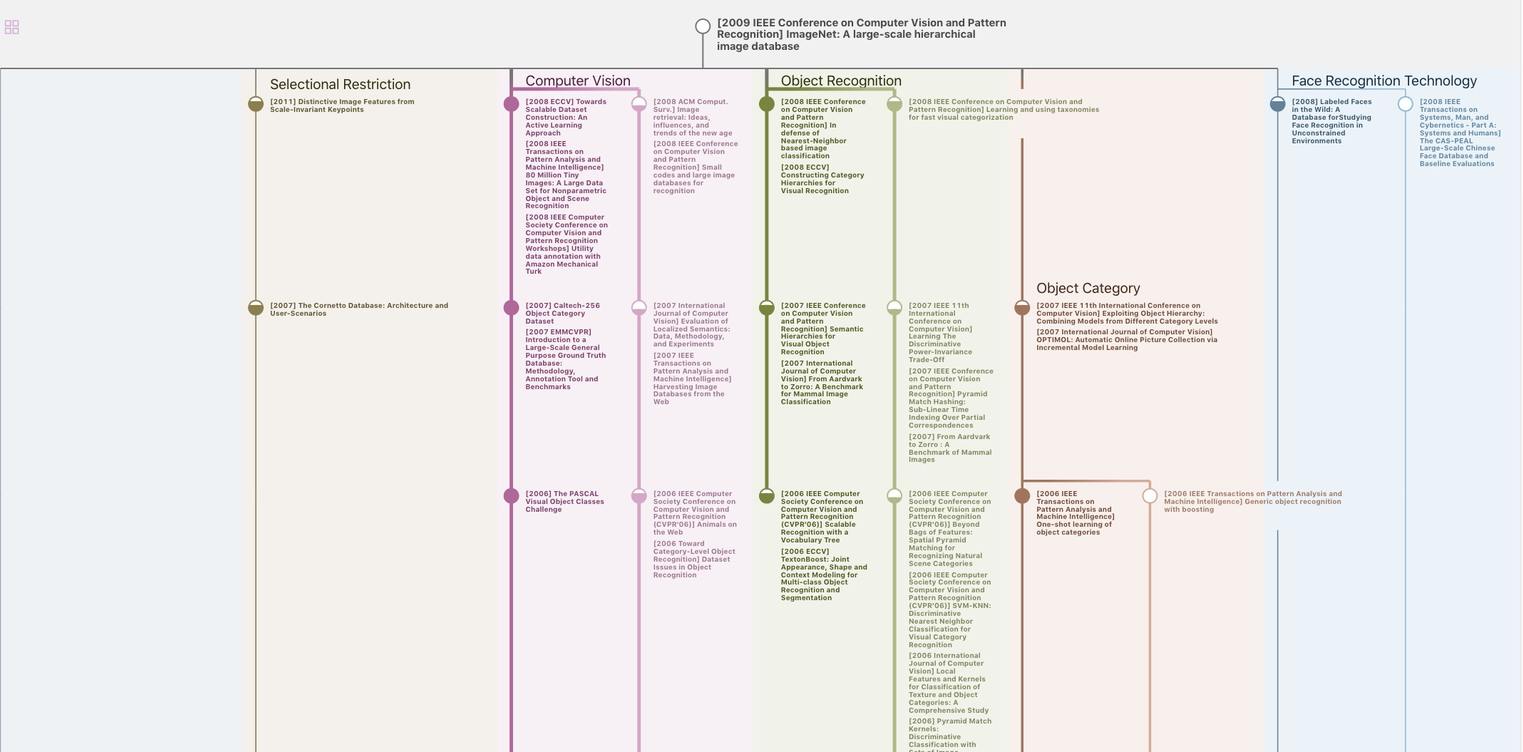
生成溯源树,研究论文发展脉络
Chat Paper
正在生成论文摘要