In Defense of Metrics: Metrics Sufficiently Encode Typical Human Preferences Regarding Hydrological Model Performance
WATER RESOURCES RESEARCH(2023)
摘要
Building accurate rainfall-runoff models is an integral part of hydrological science and practice. The variety of modeling goals and applications have led to a large suite of evaluation metrics for these models. Yet, hydrologists still put considerable trust into visual judgment, although it is unclear whether such judgment agrees or disagrees with existing quantitative metrics. In this study, we tasked 622 experts to compare and judge more than 14,000 pairs of hydrographs from 13 different models. Our results show that expert opinion broadly agrees with quantitative metrics and results in a clear preference for a Machine Learning model over traditional hydrological models. The expert opinions are, however, subject to significant amounts of inconsistency. Nevertheless, where experts agree, we can predict their opinion purely from quantitative metrics, which indicates that the metrics sufficiently encode human preferences in a small set of numbers. While there remains room for improvement of quantitative metrics, we suggest that the hydrologic community should reinforce their benchmarking efforts and put more trust in these metrics.
更多查看译文
关键词
hydrology,metrics,hydrograph,visual inspection,rainfall-runoff,machine learning
AI 理解论文
溯源树
样例
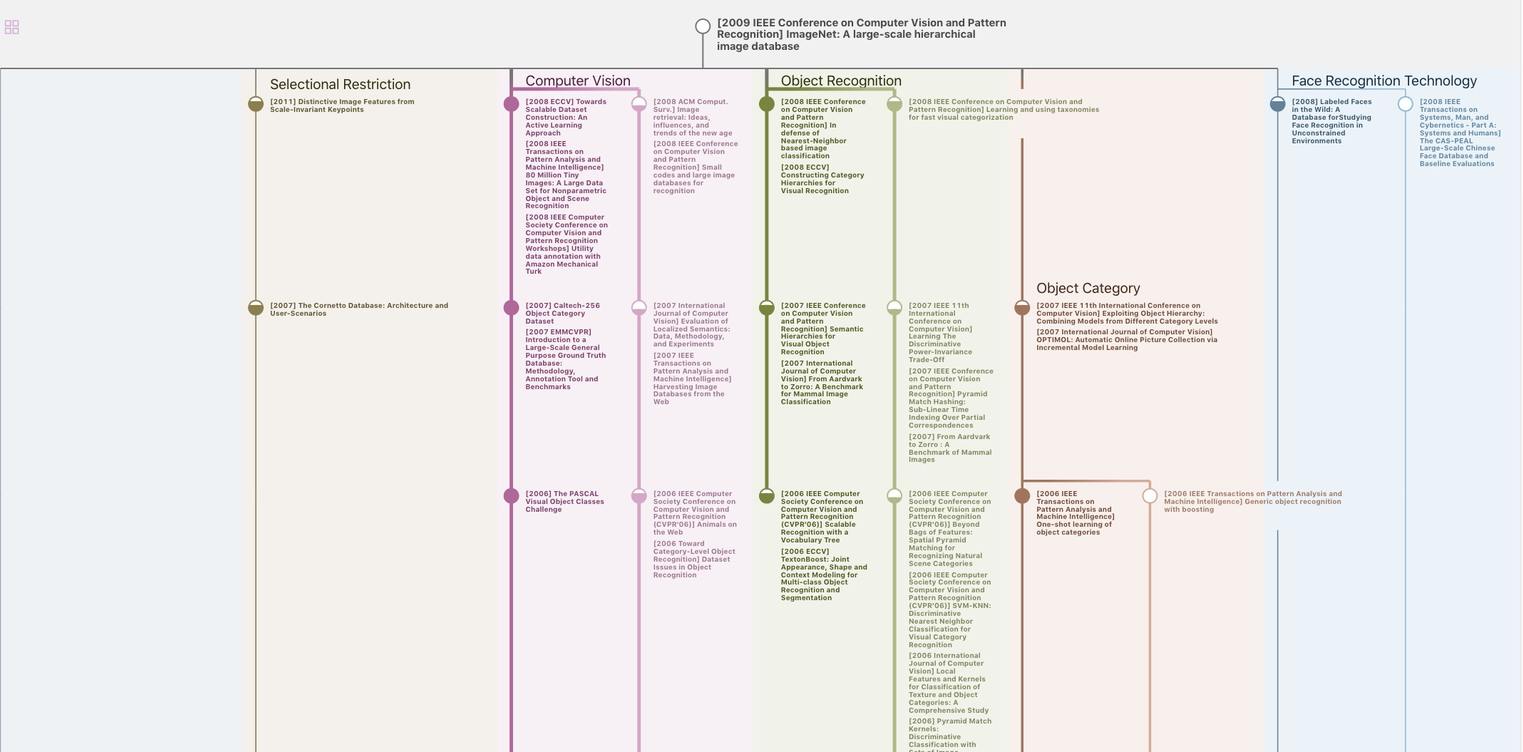
生成溯源树,研究论文发展脉络
Chat Paper
正在生成论文摘要