Enhancing Nonlinear Subspace Identification Using Sparse Bayesian Learning with Spike and Slab Priors
JOURNAL OF VIBRATION ENGINEERING & TECHNOLOGIES(2023)
摘要
Purpose Nonlinear system identification heavily relies on the accuracy of nonlinear unit model selection. To improve identification accuracy, the Sparse Bayesian Learning method is incorporated into the nonlinear subspace. Enhanced nonlinear subspace identification is proposed. Methods The nonlinear term in the system is treated as an internal excitation. By applying low-level excitation, the response of the structure can be approximated as linear, allowing for the determination of the linear frequency response function of the structure. High-level excitation is then applied to separate the response caused by intrinsic nonlinear force excitation. The type of nonlinearity is evaluated using Spike-and-Slab Priors for Sparse Bayesian Learning. Finally, the screened nonlinear elements are substituted into subspace identification to determine nonlinear parameters. Results The effectiveness of this method in dealing with nonlinear stiffness and damping is verified through a simulation example and its robustness is further discussed. Experiments on negative stiffness systems also demonstrate the method's good applicability when dealing with complex damping. Conclusion Incorporating the Sparse Bayesian Learning method into the nonlinear subspace significantly improves the accuracy of nonlinear system identification. The proposed approach effectively deals with nonlinear stiffness and damping, as validated by simulation results. The method's robustness is further demonstrated through extensive discussions, while experiments on negative stiffness systems showcase its applicability in complex damping scenarios.
更多查看译文
关键词
nonlinear subspace identification,sparse bayesian learning,spike
AI 理解论文
溯源树
样例
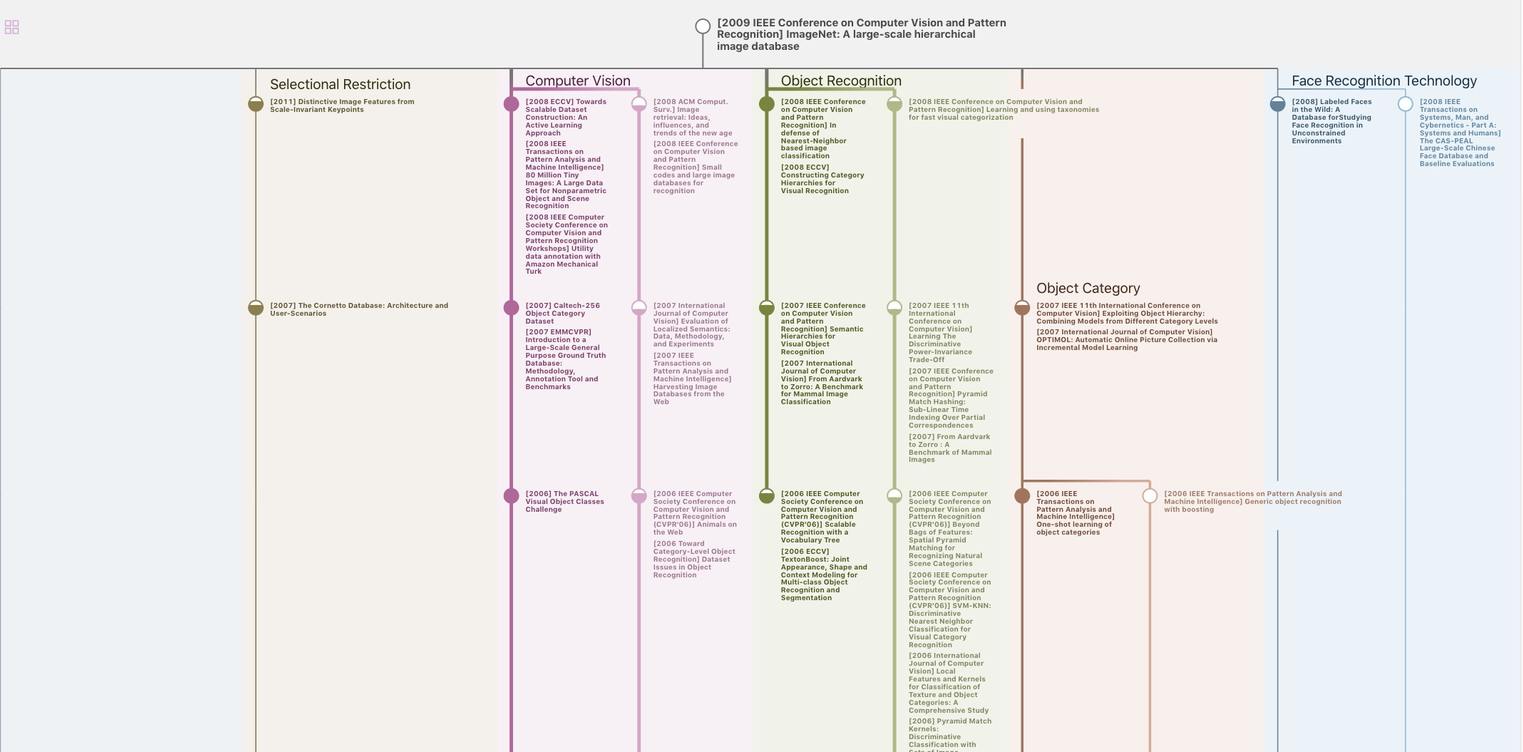
生成溯源树,研究论文发展脉络
Chat Paper
正在生成论文摘要