A Digital Predistortion Technique Based on the Dimension Weighted Blind K-Nearest Neighbor Algorithm br
JOURNAL OF ELECTRONICS & INFORMATION TECHNOLOGY(2023)
摘要
In traditional Digital PreDistortion (DPD) models, the same set of polynomial models and the samememory model are usually used to linearize the Power Amplifier (PA) at all input signal powers. However, thePAs exhibit different nonlinear characteristics and different memory effects at different power levels. In order tosolve this problem, a DPD model based on the blind K-Nearest Neighbor (KNN) algorithm with dimensionweighting is proposed. The input signal sequence is classified by the proposed model according to themagnitudes of amplifier's current input signal and the memory input signal with the dimension-weighted KNNclassification .And sub-models are established for each type of input signal sequence. The proposed method isverified experimentally by a Doherty PA, a three carrier Long Term Evolution (LTE) signal with a bandwidthof 30 MHz and a frequency point of 2.2 GHz is used as the input, the feedback channel is sampled using asampling rate of 122.88 MHz. When the dimensional-weighted blind KNN classification method is combinedwith the Memory Polynomial (MP) model, the forward modeling performance and digital pre-distortionperformance for the PA which exceed the performance of Generalized Memory Polynomial (GMP) model andMP model are manifested in the experiment. The excellent performance of the proposed model is verified in the experiment.
更多查看译文
关键词
Digital PreDistortion(DPD), Blind K-Nearest Neighbor(KNN) classification, Dimension weighting, Power Amplifier(PA), Behavioral model
AI 理解论文
溯源树
样例
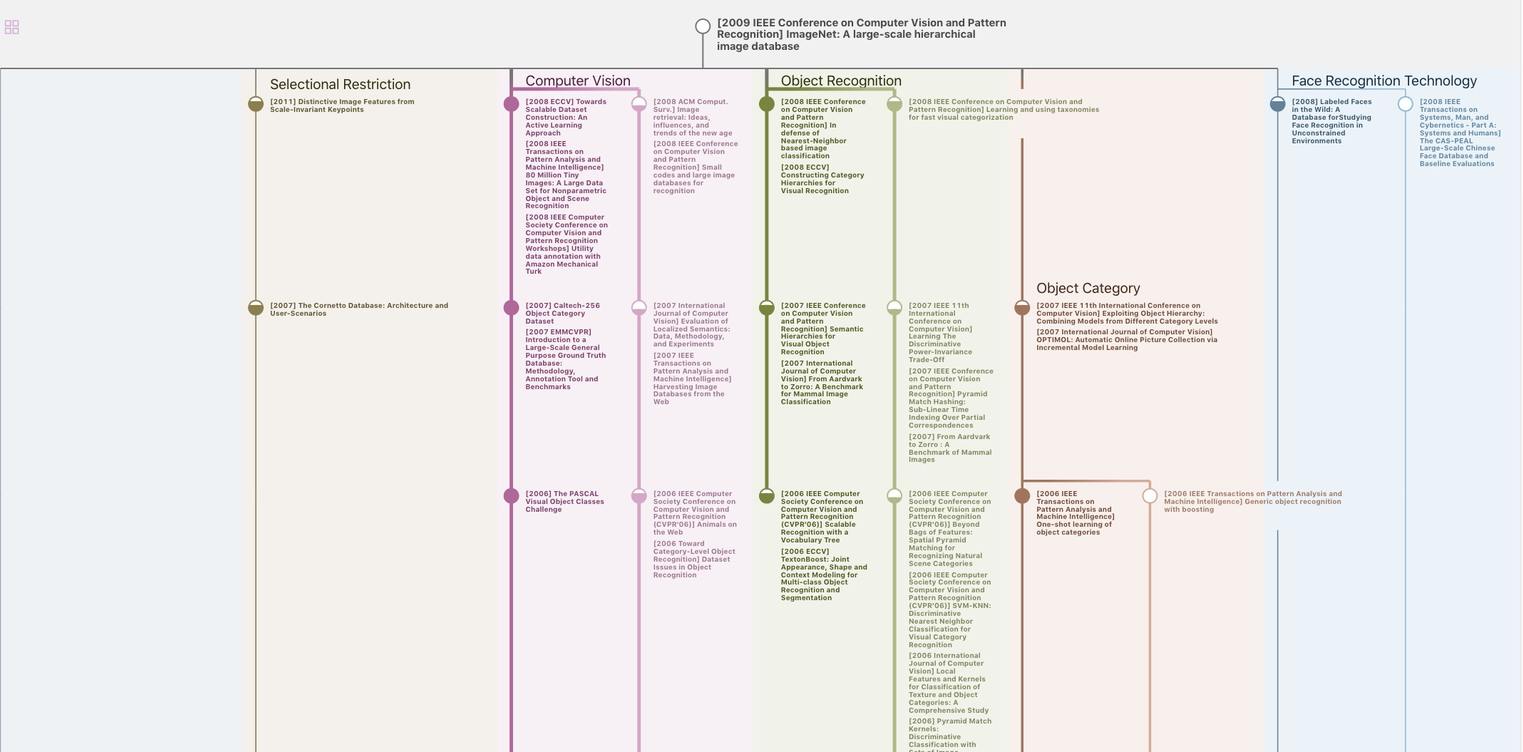
生成溯源树,研究论文发展脉络
Chat Paper
正在生成论文摘要