Ultra-efficient fully programmable membership function generator based on independent double-gate FinFET technology
INTERNATIONAL JOURNAL OF CIRCUIT THEORY AND APPLICATIONS(2023)
摘要
This paper demonstrates an ultra-efficient, fully programmable membership function generator (MFG) utilizing independent double-gate (IDG) FinFET technology. The proposed MFG can produce s-shaped, z-shaped, triangular, and trapezoidal membership functions. By employing only six transistors, the designed MFG provides full controllability over the height, position, width, and slope of the generated waveforms. Without using any additional transistor and changing the dimensions, the proposed MFG can calibrate the slope of the output based on the back-gate bias voltage of the IDG-FinFETs. According to our extensive simulations, the proposed MFG shows promising improvements in transistor count (70%), power-delay-product (PDP) (80%), and maximum absolute error (61%) as compared with its state-of-the-art counterparts. To benchmark the functionality of the proposed MFG in practical applications, our generated membership function is exploited as the neuron's activation function in a multilayer perceptron (MLP) neural network. The simulation results indicate that the training process of the simulated MLP with the proposed MFG closely tracks the results obtained from the ideal MLP with the sigmoid activation function. A figure of merit (FoM) is defined considering the hardware efficiency and accuracy of the neural networks to evaluate the entire performance of the proposed MFG. The FoM simulations demonstrate that the proposed MFG presents an excellent trade-off between hardware performance and accuracy in neural network applications. Our results emphasize that the proposed MFG is a potential candidate for designing high-performance on-chip neural networks.
更多查看译文
关键词
programmable membership function generator
AI 理解论文
溯源树
样例
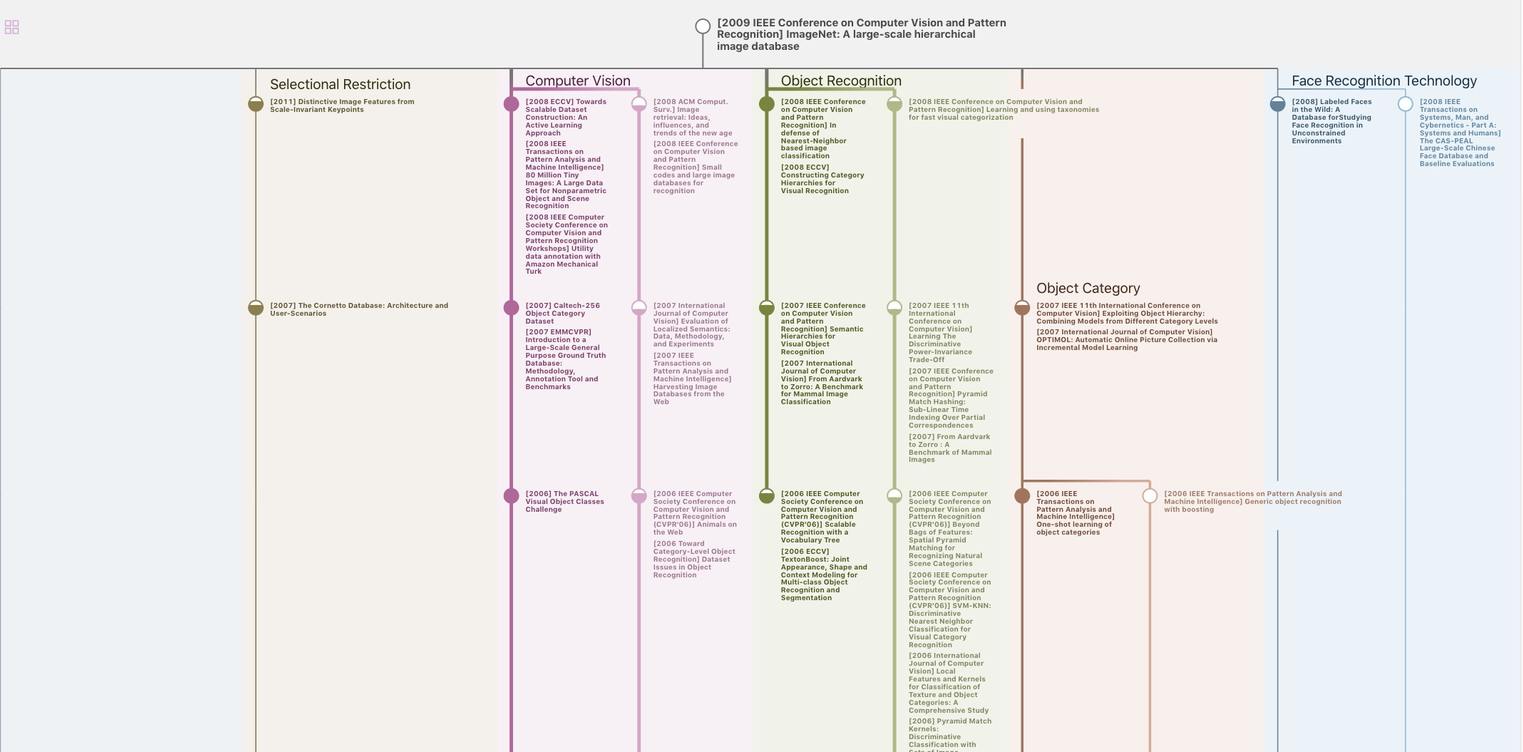
生成溯源树,研究论文发展脉络
Chat Paper
正在生成论文摘要