Intelligent modeling with physics-informed machine learning for petroleum engineering problems
ADVANCES IN GEO-ENERGY RESEARCH(2023)
摘要
The advancement in big data and artificial intelligence has enabled a novel exploration mode for the study of petroleum engineering. Unlike theory-based solution methods, the data-driven intelligent approaches demonstrate superior flexibility, computational efficiency and accuracy for dealing with complex multi-scale, and multi-physics problems. However, these intelligent models often disregard physical laws in pursuit of error minimization, which leads to certain uncertainties. Therefore, physics-informed machine learning approaches have been developed based on data, guided by physics, and supported by machine learning models. This study summarizes four embedding mechanisms for introducing physical information into machine learning models, including input data-based embedding, model architecture-based embedding, loss function-based embedding, and model optimization-based embedding mechanism. These "data + physics" dual-driven intelligent models not only exhibit higher prediction accuracy while adhering to physic laws, but also accelerate the convergence to improve computational efficiency. This paradigm will facilitate the guide developments in solving petroleum engineering problems toward a more comprehensive and efficient direction.
更多查看译文
关键词
intelligent modeling,machine learning,engineering,physics-informed
AI 理解论文
溯源树
样例
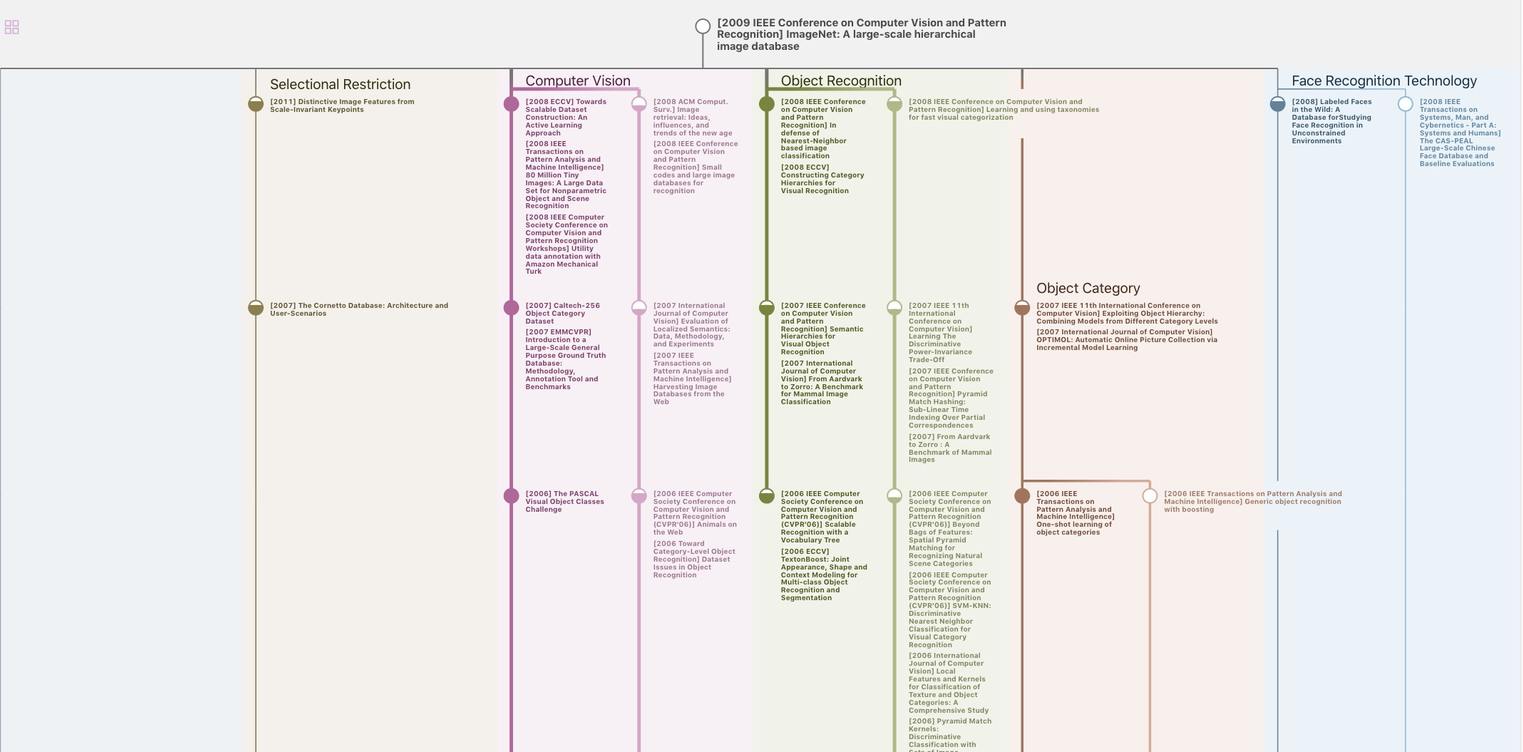
生成溯源树,研究论文发展脉络
Chat Paper
正在生成论文摘要